Drug repositioning based on heterogeneous networks and variational graph autoencoders.
Frontiers in pharmacology(2022)
摘要
Predicting new therapeutic effects (drug repositioning) of existing drugs plays an important role in drug development. However, traditional wet experimental prediction methods are usually time-consuming and costly. The emergence of more and more artificial intelligence-based drug repositioning methods in the past 2 years has facilitated drug development. In this study we propose a drug repositioning method, VGAEDR, based on a heterogeneous network of multiple drug attributes and a variational graph autoencoder. First, a drug-disease heterogeneous network is established based on three drug attributes, disease semantic information, and known drug-disease associations. Second, low-dimensional feature representations for heterogeneous networks are learned through a variational graph autoencoder module and a multi-layer convolutional module. Finally, the feature representation is fed to a fully connected layer and a Softmax layer to predict new drug-disease associations. Comparative experiments with other baseline methods on three datasets demonstrate the excellent performance of VGAEDR. In the case study, we predicted the top 10 possible anti-COVID-19 drugs on the existing drug and disease data, and six of them were verified by other literatures.
更多查看译文
关键词
COVID-19,drug repositioning,graph representation learning,heterogeneous network,variational graph autoencoders
AI 理解论文
溯源树
样例
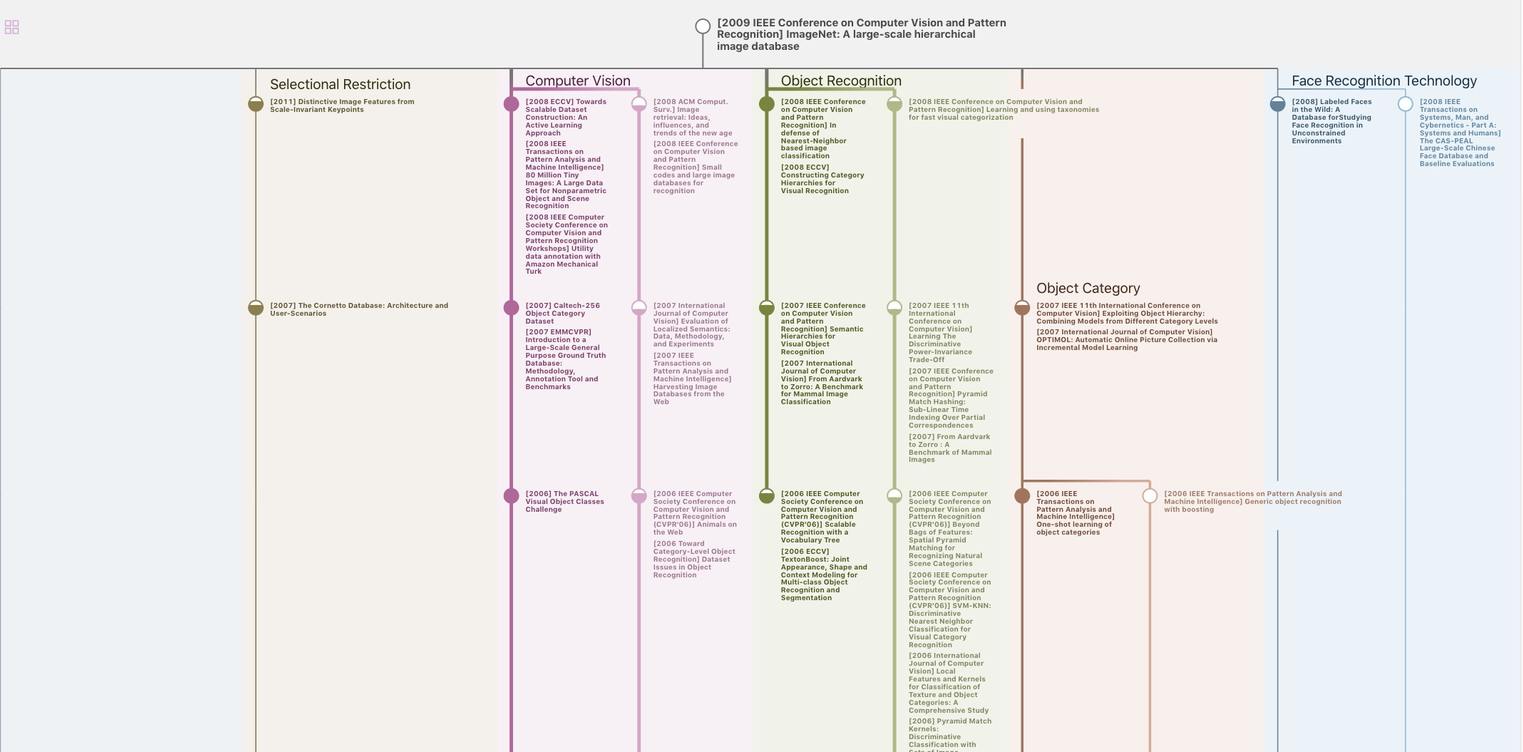
生成溯源树,研究论文发展脉络
Chat Paper
正在生成论文摘要