A novel prediction model for wind power based on improved long short-term memory neural network
Energy(2023)
Abstract
Wind power generation technology has attracted worldwide attention. However, its inherent nonlinearity and uncertainty make itself hard to be accurately predicted. As a result, exploring the ways to remedy these defects become the key to the stable operation of power grid. This paper proposed a wind power prediction model based on the improved Long Short-Term Memory (LSTM) network to fit the nonlinearity between data variables and wind power. The chaotic sequence and Gaussian mutation strategy are introduced into the original sparrow algorithm, so as to improve its stability and search performance. Then, the modified sparrow algorithm is implemented to adjust the LSTM network's hyperparameters like batch size, cell number and learning rate; and therefore the prediction accuracy is increased. After that, the improved model is applied to the data sets of a wind farm in Hunan province during the four seasons of 2020. And then it is compared with other four combined models. The experimental results show that, the RMSE of the proposed prediction method is reduced respectively by 37.37%, 13.44%, 10.64% and 20.78% in four seasons. It is proved that the proposed method improves the accuracy for wind power prediction and the effectiveness for power dispatching.
MoreTranslated text
Key words
Wind power prediction,Long short-term memory neural network,Improved sparrow algorithm,Gaussian mutation strategy,Tent chaotic sequence
AI Read Science
Must-Reading Tree
Example
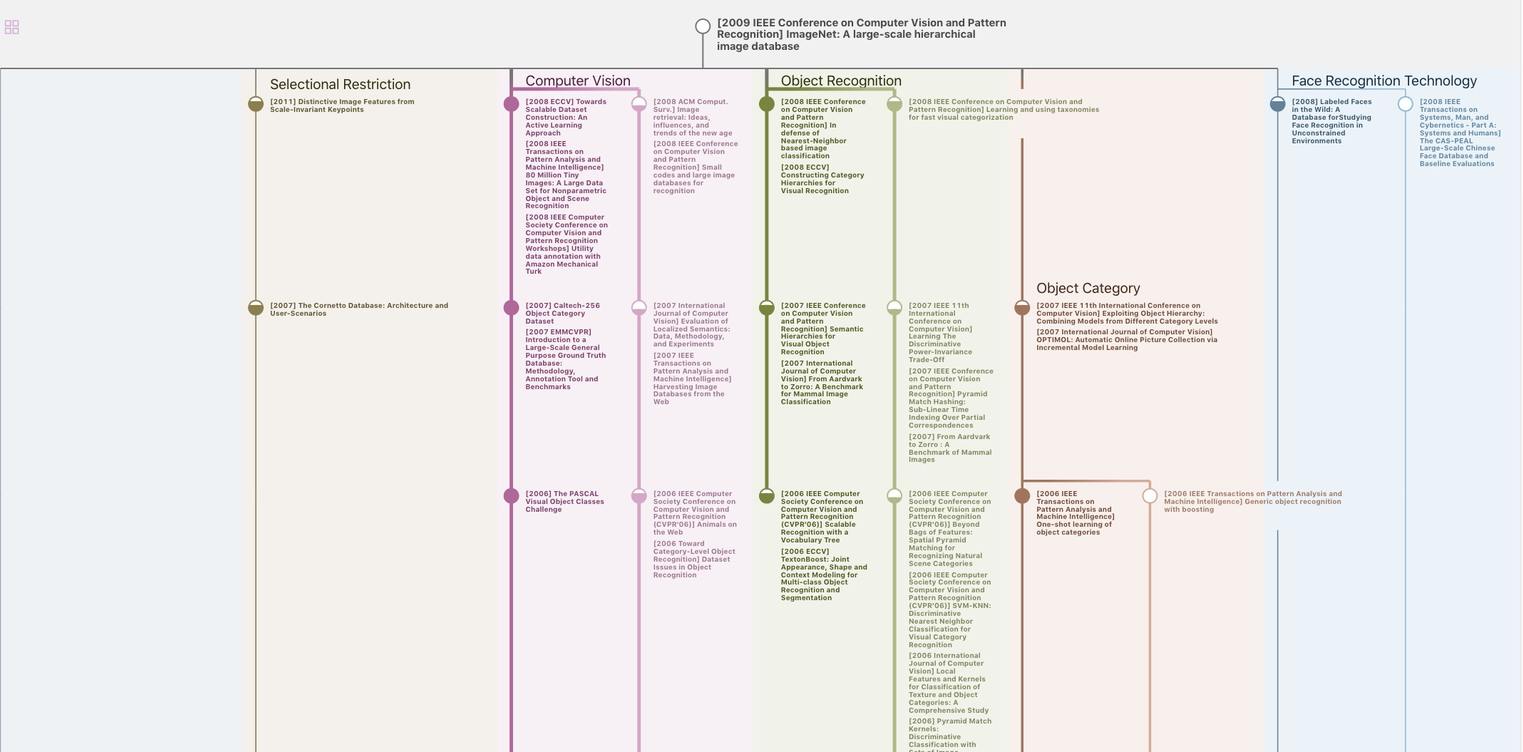
Generate MRT to find the research sequence of this paper
Chat Paper
Summary is being generated by the instructions you defined