Generalized Label Grouping for Scalable Trajectory Estimation
2022 11th International Conference on Control, Automation and Information Sciences (ICCAIS)(2022)
摘要
Multi-Object Tracking (MOT) is concerned with estimating trajectories from sensor measurements. MOT using the Random Finite Set (RFS) framework has been gaining popularity due to its rigorous mathematical foundation and versatility in applications. Notably, large-scale trajectory estimation can be successfully achieved by the label-partitioned Generalized Labeled Multi-Bernoulli (GLMB) filter framework. In this work, we propose an efficient method for grouping object labels in scalable GLMB filtering. Specifically, the label grouping problem for parallel computation is generalized by considering the intersection of predicted measurements, i.e., uncertainty regions. The proposed approach provides a flexible criterion to construct label graphs, whereupon a large number of object labels can be rapidly determined whether to be grouped or not. We demonstrate the performance of our method via large-scale data sets.
更多查看译文
关键词
Multi-Object Tracking,Random Finite Set,Generalized Labeled Multi-Bernoulli,Label Grouping
AI 理解论文
溯源树
样例
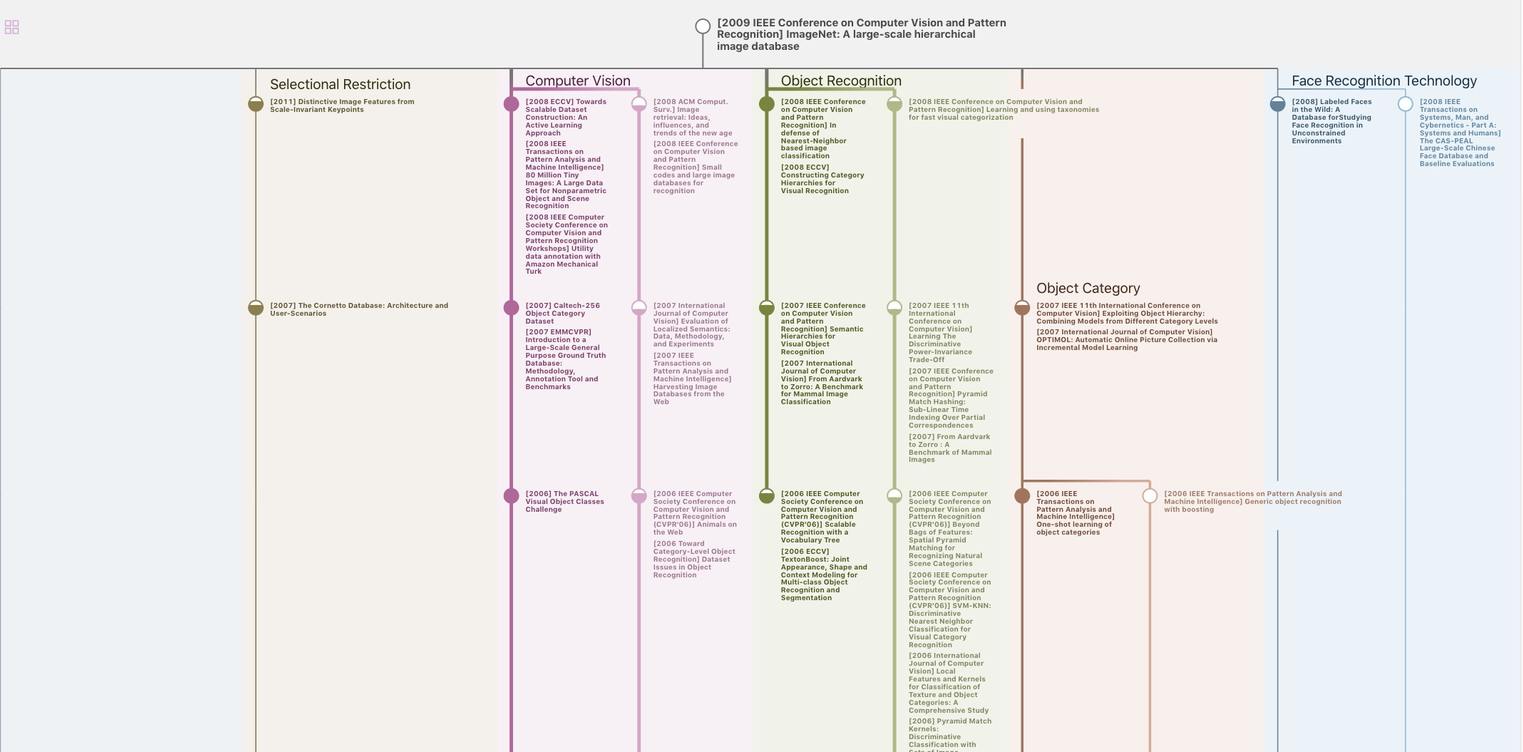
生成溯源树,研究论文发展脉络
Chat Paper
正在生成论文摘要