Conditional Generative Adversarial Networks for modelling fuel sprays
Energy and AI(2023)
摘要
In this study, the probabilistic, data driven nature of the generative adversarial neural networks (GANs) was utilized to conduct virtual spray simulations for conditions relevant to aero engine combustors. The model consists of two sub-modules: (i) an autoencoder converting the variable length droplet trajectories into fixed length, lower dimensional representations and (ii) a Wasserstein GAN that learns to mimic the latent representations of the evaporating droplets along their lifetime. The GAN module was also conditioned with the injection location and the diameters of the droplets to increase the generalizability of the whole framework. The training data was provided from highly resolved 3D, transient Eulerian–Lagrangian, large eddy simulations conducted with OpenFOAM. Neural network models were created and trained within the open source machine learning framework of PyTorch. Predictive capabilities of the proposed method was discussed with respect to spray statistics and evaporation dynamics. Results show that conditioned GAN models offer a great potential as low order model approximations with high computational efficiency. Nonetheless, the capabilities of the autoencoder module to preserve local dependencies should be improved to realize this potential. For the current case study, the custom model architecture was capable of conducting the simulation in the order of seconds after a day of training, which had taken one week on HPC with the conventional CFD approach for the same number of droplets (200,000 trajectories).
更多查看译文
关键词
Generative Adversarial Networks,Generative learning,Fuel injection,Aero engines,Multivariate time series
AI 理解论文
溯源树
样例
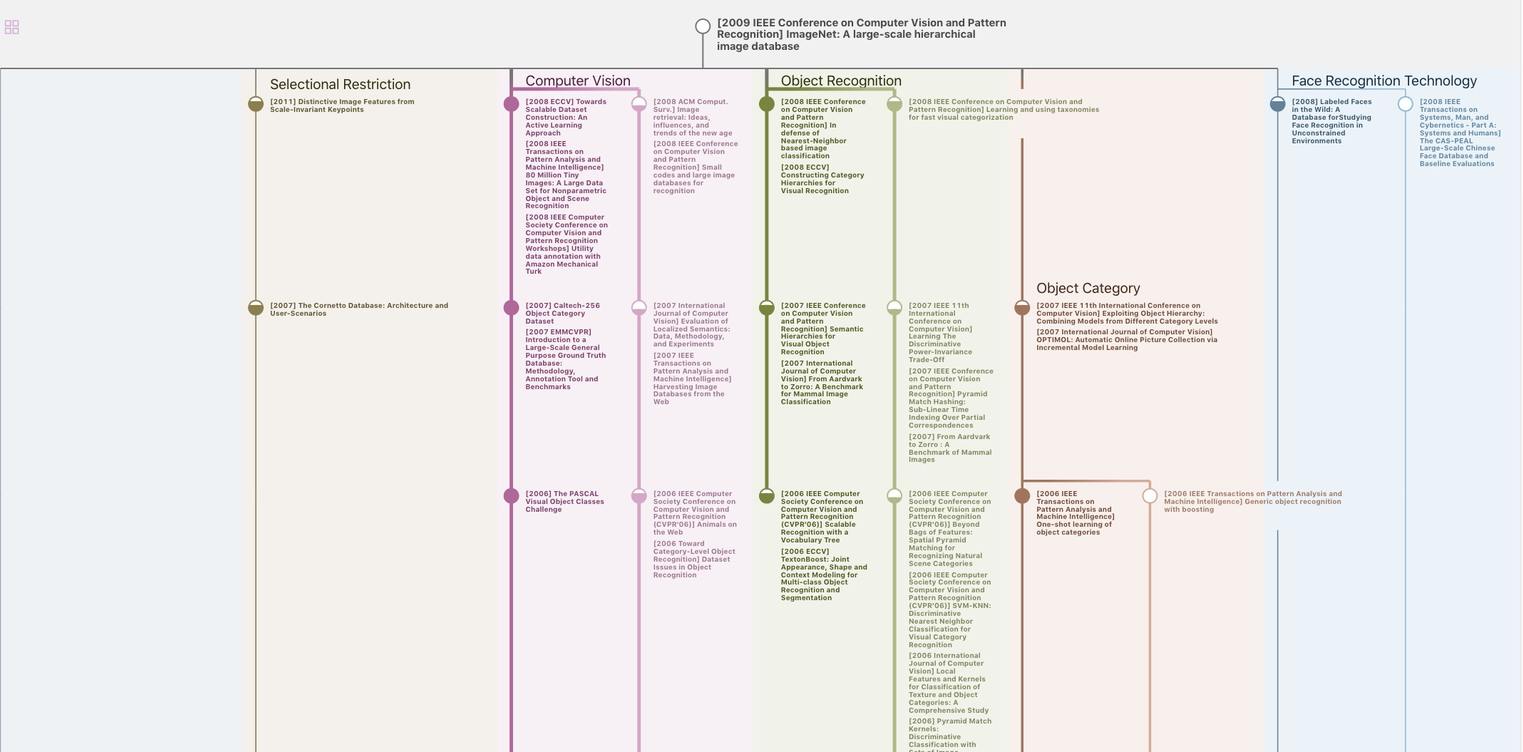
生成溯源树,研究论文发展脉络
Chat Paper
正在生成论文摘要