Using Autoencoders to Reduce Dimensionality of DICOM Metadata
2022 International Conference on Electrical, Computer, Communications and Mechatronics Engineering (ICECCME)(2022)
摘要
Digital Imaging and Communication in Medicine (DICOM) is a standardized format for storing medical images enriched with descriptive data. It consists of a number of informative header tags. E.g., these tags contain information concerning imaging technique, patient weight, patient age and so on. In machine learning, these tags can, among other things, be used for categorization, classification, and general manipulation of DICOM data. However, because of their number and variety, using them for automation undoubtedly impacts computational performance. To tackle this problem, the possibility of using auto encoders is explored in this manuscript. It was hypothesized that clustering data compressed with autoencoders can achieve the same results as when working with raw - uncompressed data. To support this claim, clustering of compressed and uncom- pressed data was performed on a dataset of 25, 000 DICOM files from the Clinical Hospital Centre Rijeka PACS. The results show no significant difference between compressed and uncompressed data (p > 0.05), thus confirming that equally good clustering results can be accomplished using a smaller representation.
更多查看译文
关键词
DICOM,Autoencoders,Medical Imaging,Clustering
AI 理解论文
溯源树
样例
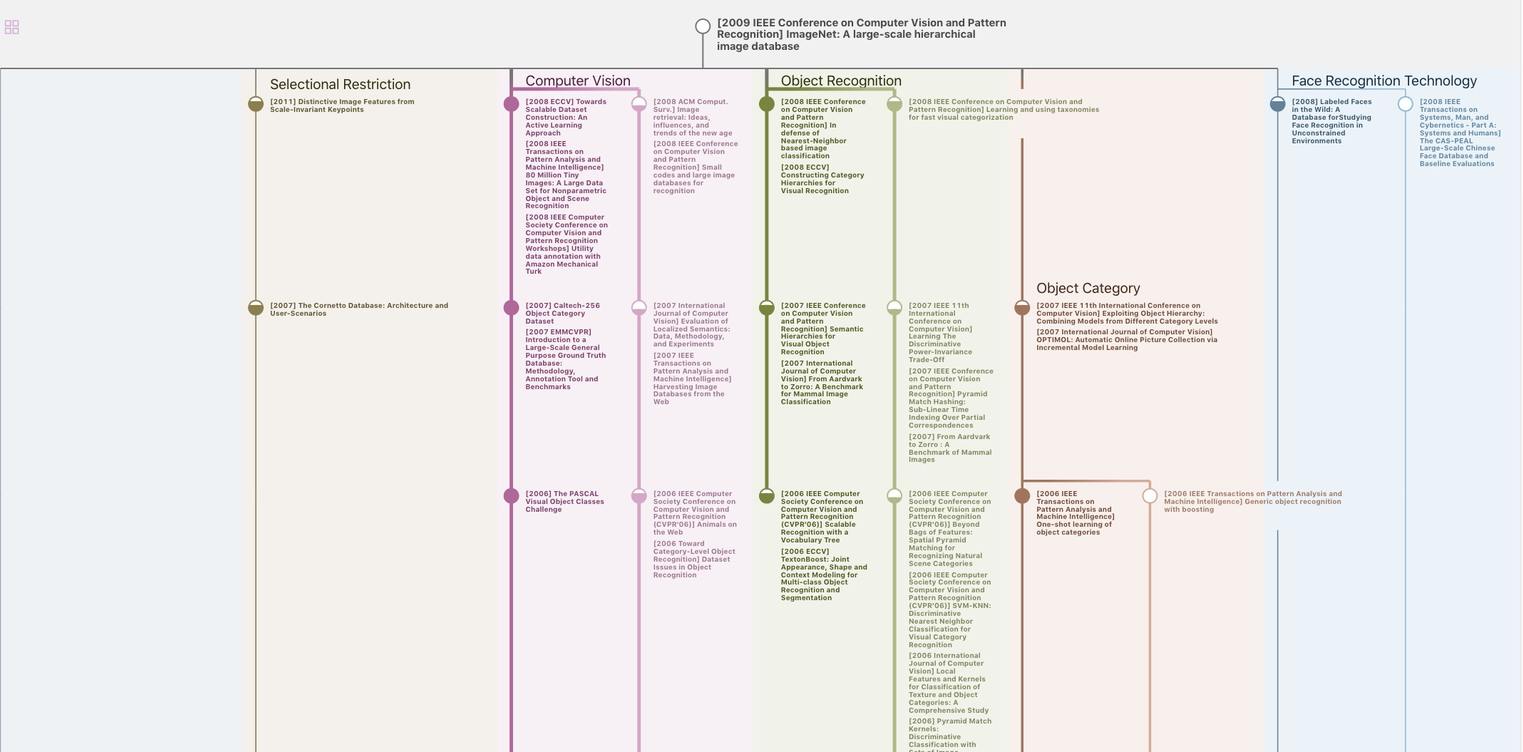
生成溯源树,研究论文发展脉络
Chat Paper
正在生成论文摘要