A Novel Short-term Vehicle Location Prediction using Temporal Graph Neural Networks
2022 IEEE Latin-American Conference on Communications (LATINCOM)(2022)
摘要
Location prediction is essential for location-based applications such as route recommendation systems, resource allocation optimization in congested areas, congestion avoidance, traffic management, just to mention a few. Knowing approximate vehicle locations can enhance our ability to better manage and prepare the existing mobile edge computing resources in a Vehicular ad hoc network. In this paper, we focus on a graph neural network model to estimate vehicle locations in a timely manner. We first model the vehicle locations based on events that happen between different regions of an area and the car itself. Leveraging our event-based model, we introduce Temporal Location Prediction (TLP) to capture essential features from each node, edges, and neighboring nodes to achieve timely location prediction. Afterwards, instead of using GPS coordinates as input, we demonstrate a new data structure to feed Bidirectional LSTM (BiLSTM) and LSTM for vehicle location prediction in different time intervals. Thus, our main contribution is a network model that utilizes a Temporal Graph neural network for dynamic location prediction. We explain the advantages and disadvantages of each model and how we can improve them. Our experiments on a real dataset show that our model (TLP) outperforms LSTM and BiLSTM in short-term prediction, considering the same scenario and conditions.
更多查看译文
关键词
vehicle location prediction,time series,VANET,LSTM,GNN,TGN
AI 理解论文
溯源树
样例
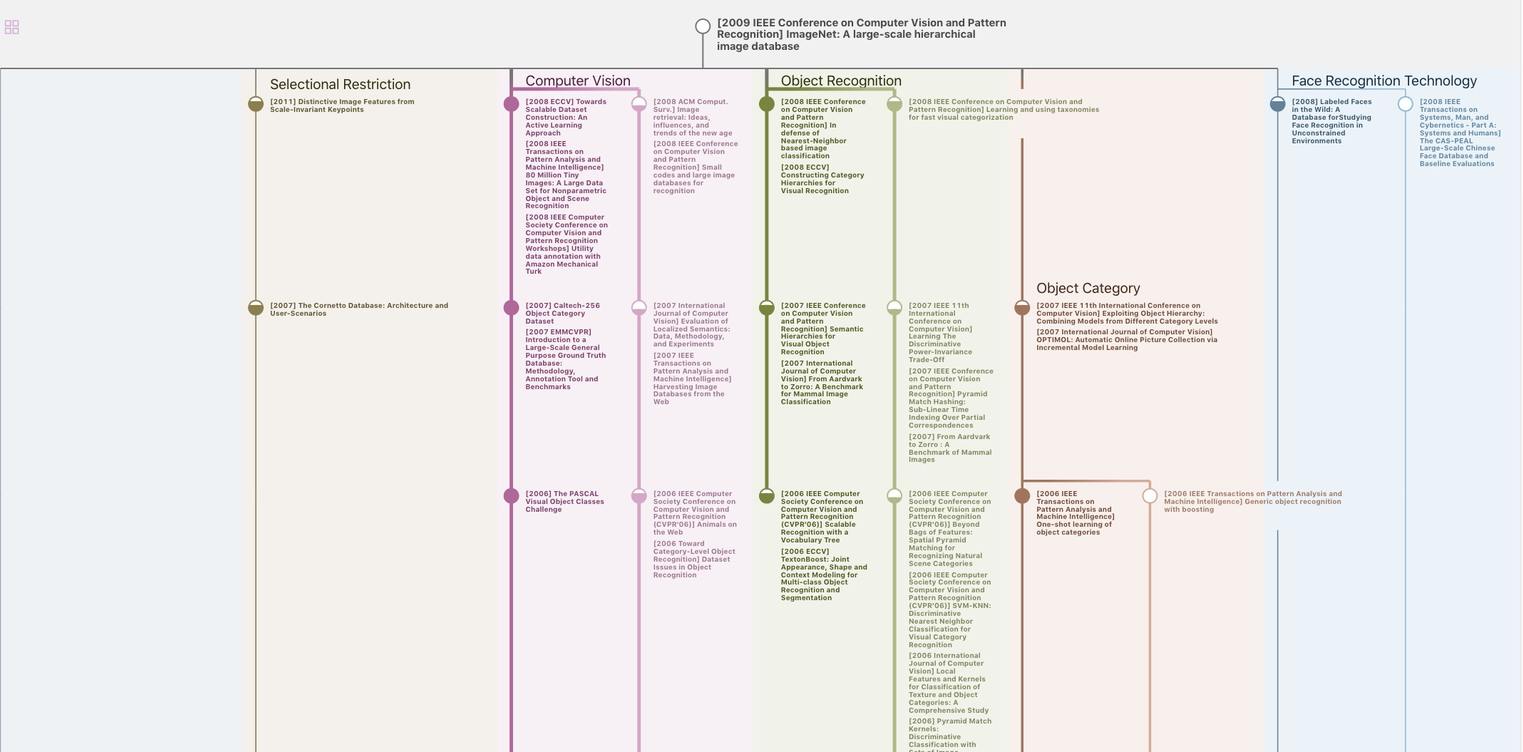
生成溯源树,研究论文发展脉络
Chat Paper
正在生成论文摘要