Few-shot Medical Image Classification with MAML Based on Dice Loss
2022 IEEE 2nd International Conference on Data Science and Computer Application (ICDSCA)(2022)
Abstract
In medical image processing based on deep learning, medical image datasets are usually few-shot and unbalanced between classes. This will cause the model to overfit and be biased towards the class with more samples during training and the accuracy of the class with fewer samples will decrease. As a result, the overall accuracy will also decrease. To address these issues, the model-agnostic meta-learning based on dice loss is proposed. The algorithm avoids the need for a large amount of data to be driven and can quickly adapt to new tasks with only a small amount of labeled data. Meanwhile, the objective function can be optimized autonomously in the direction of a small number of classes during training. Finally, good results are achieved on two few-shot datasets of medical images. Our method can provide a solution to achieve good results with less data cost in medical image processing.
MoreTranslated text
Key words
Machine learning,few-shot learning,model-agnostic meta-learning,imbalanced learning,dice loss
AI Read Science
Must-Reading Tree
Example
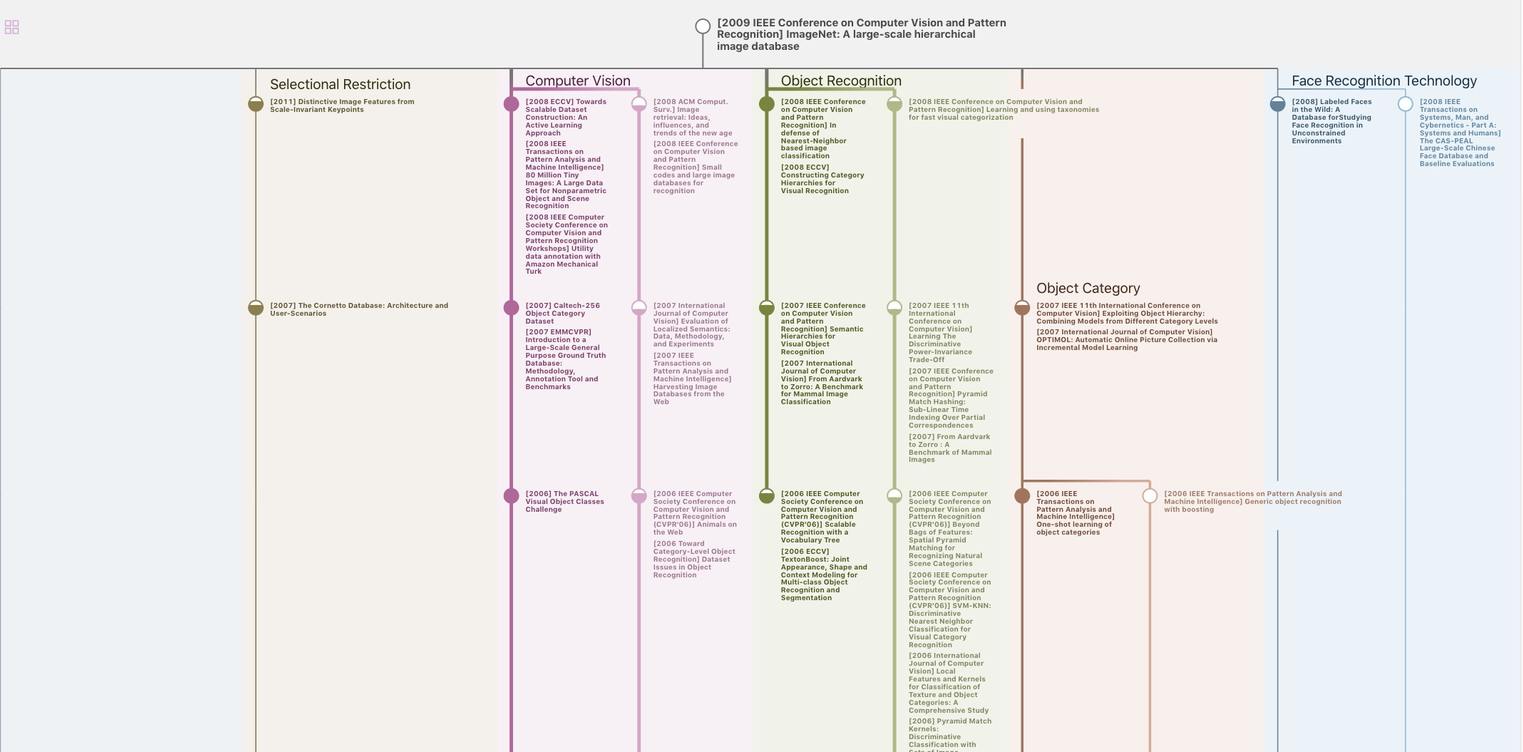
Generate MRT to find the research sequence of this paper
Chat Paper
Summary is being generated by the instructions you defined