Multi-dimensional alignment via Variational Autoencoders for Generalized Zero-Shot and Few-Shot Learning
2022 9th International Conference on Behavioural and Social Computing (BESC)(2022)
摘要
Generalized zero-shot learning (GZSL) aims to solve the category recognition tasks for unseen categories under the setting that training samples only contain seen classes while unseen classes are not available. This research is vital as there are always existing new categories and large amounts of unlabeled data in realistic scenarios. Previous work for GZSL usually maps the visual information of the visible classes and the semantic description of the invisible classes into the identical embedding space to bridge the gap between the disjointed visible and invisible classes, while ignoring the intrinsic features of visual images, which are sufficiently discriminative to classify themselves. To better use discriminative information from visual classes for GZSL, we propose the n-CADA-VAE. In our approach, we map the visual feature of seen classes to a high-dimensional distribution while mapping the semantic description of unseen classes to a low-dimensional distribution under the same latent embedding space, thus projecting information of different modalities to corresponding space positions more accurately. We conducted extensive experiments on four benchmark datasets (CUB, SUN, AWA1, and AWA2). The results show our model’s superior performance in generalized zero-shot as well as few-shot learning.
更多查看译文
关键词
multi-dimensional alignment,intrinsic discriminative features,generalized zero-shot learning,Variational autoencoder
AI 理解论文
溯源树
样例
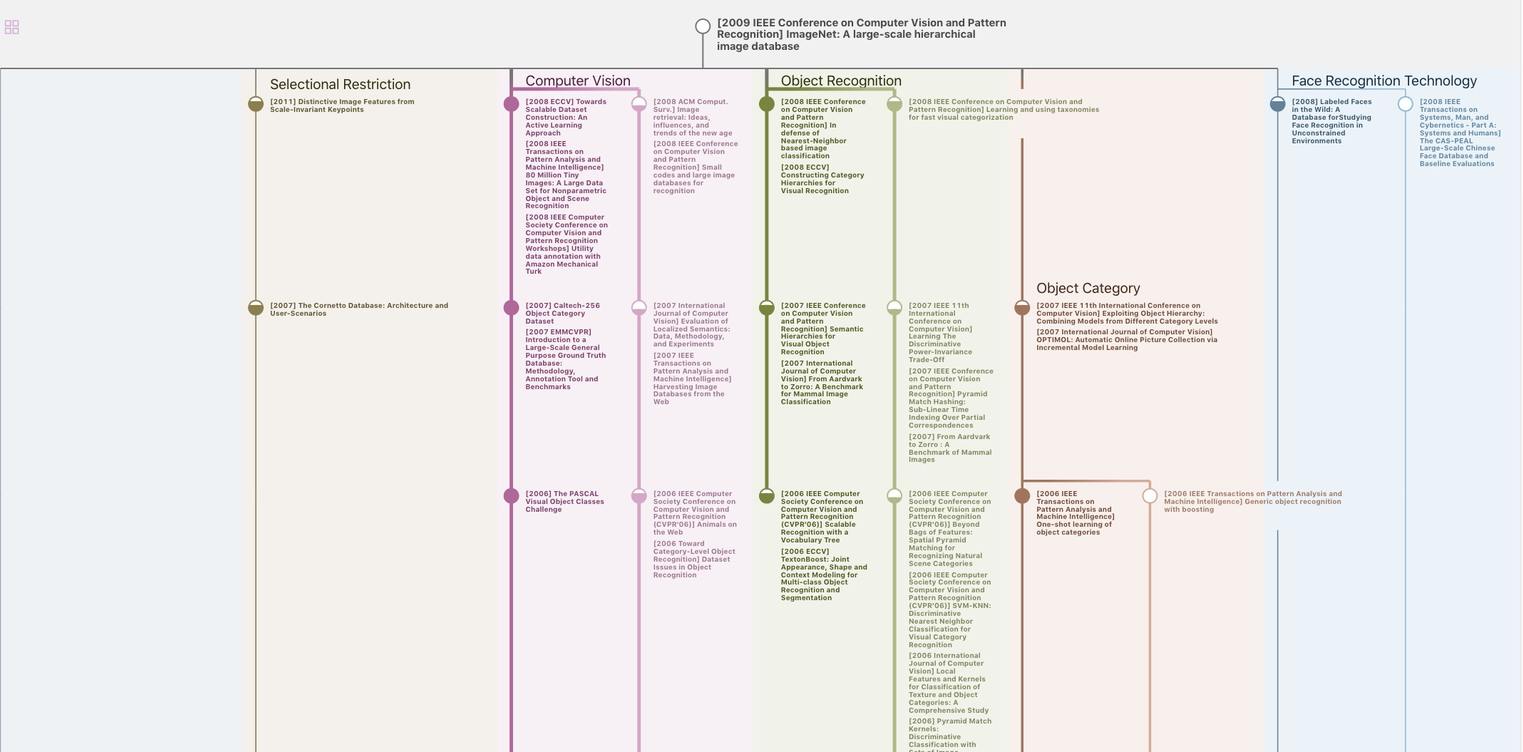
生成溯源树,研究论文发展脉络
Chat Paper
正在生成论文摘要