Hyperdimensional Hybrid Learning on End-Edge-Cloud Networks
2022 IEEE 40th International Conference on Computer Design (ICCD)(2022)
摘要
In this paper, we present Hyperdimensional Hybrid Learning (HDHL), which combines model-free and model-based Reinforcement Learning, to effectively reduce the computational cost and environment interaction for optimizing an intelligent cloud service. We first show that Hyperdimensional Q-Learning (QHD), the state-of-the-art Hyperdimensional Computing value-based Reinforcement Learning algorithm, is computationally faster than the Deep Q-Network (DQN) for this task. In addition, we demonstrate how HDHL reduces the number of environment interactions by 4.8× to learn the near optimal configuration. Our evaluation shows that HDHL is computationally more efficient than both Q-Learning algorithms, with the total time being reduced by 21.0× compared to DQN and 16.5× compared to QHD.
更多查看译文
关键词
Resource Management, Online Learning, Hyperdimensional Computing, Edge-Computing, Reinforcement Learning
AI 理解论文
溯源树
样例
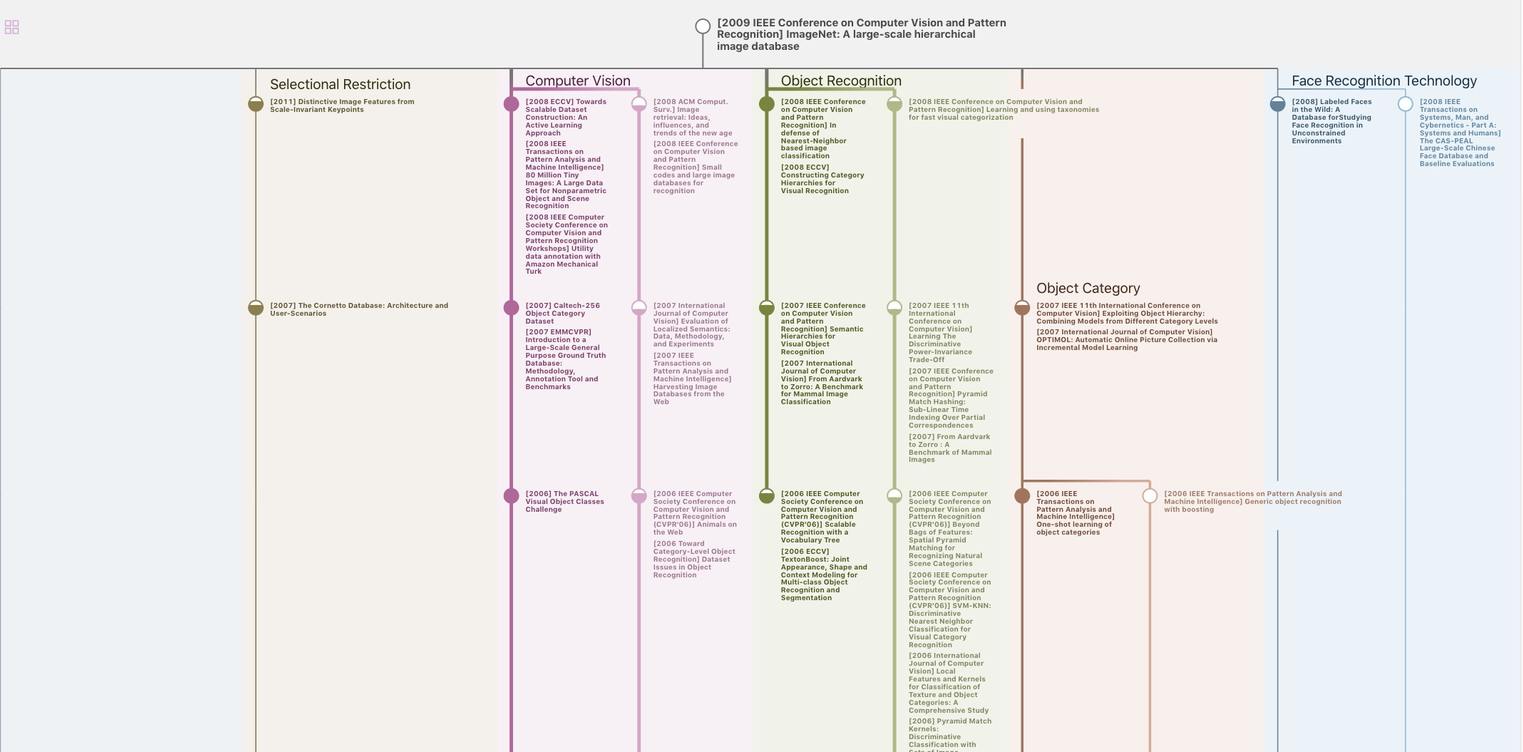
生成溯源树,研究论文发展脉络
Chat Paper
正在生成论文摘要