Computational Forecast of PM2.5 Pollution Based on Gas Emission and Traffic Volume Observations
2022 IEEE 5th International Conference on Knowledge Innovation and Invention (ICKII )(2022)
摘要
Air pollution has recently been a prevalent issue due to the fast development of cities in countries. Thus, issues related to particulate matter, PM2.5 have been investigated as it is a major indicator of air quality and causes respiratory and cardiovascular diseases in long-term exposure. We propose an adaptive long short-term memory (LSTM) model for short-term prediction and a hierarchical combination of the LSTM and convolutional neural network (CNN) models to deal with larger data for long-term prediction. The traffic data is obtained from Google Maps, and the gas emission data is obtained from the environmental protection administration (EPA) of Taiwan via various weather monitoring stations in the proximity of the target cities. The aim of this study is t is to guide the government toward a greener urban environment. The analysis result provides important protocols for gas emission and traffic control to reduce PM2.5 pollution for a greener urban environment.
更多查看译文
关键词
convolutional neural network,particular matter,long short-term memory,air pollution forecasting
AI 理解论文
溯源树
样例
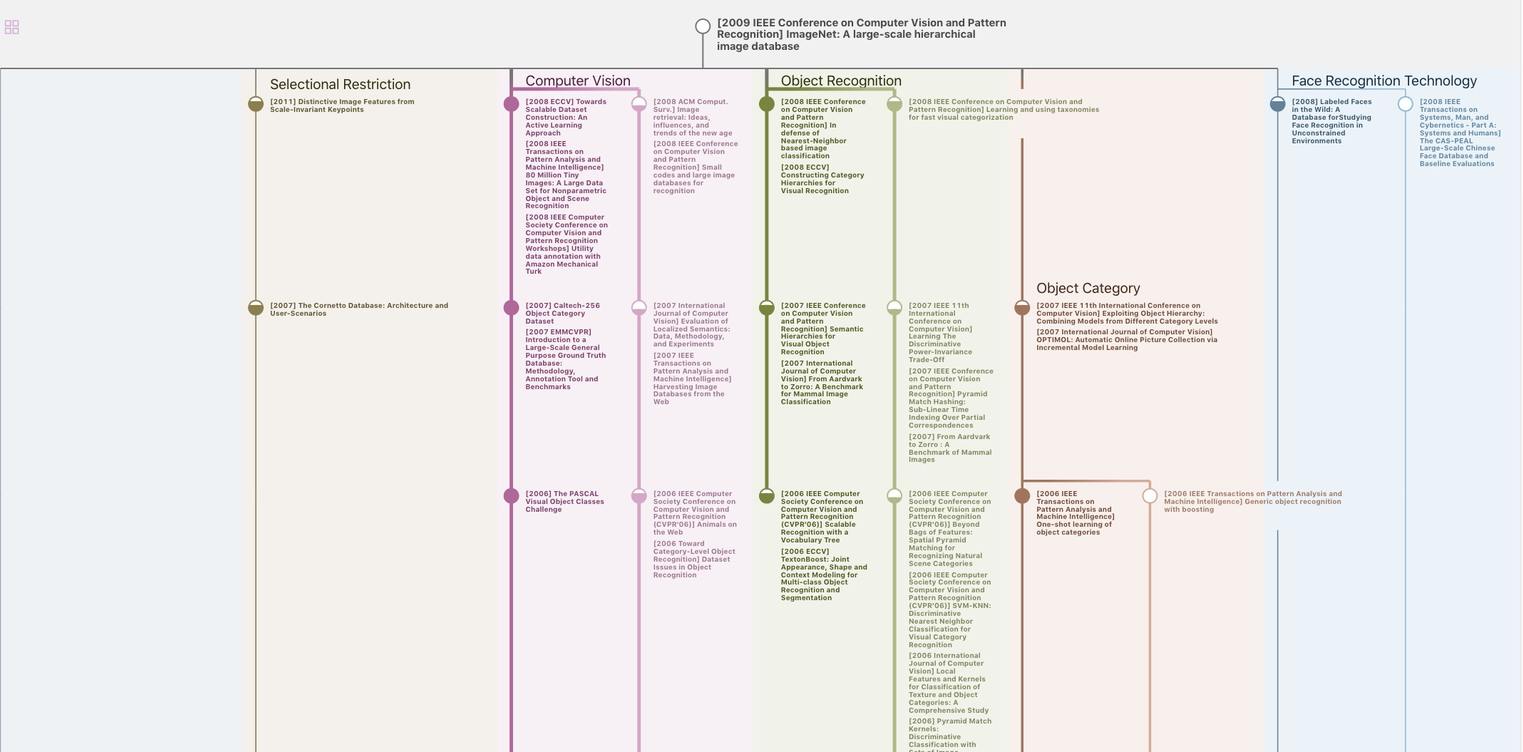
生成溯源树,研究论文发展脉络
Chat Paper
正在生成论文摘要