Federated Fuzzy Clustering for Longitudinal Health Data
2022 IEEE/ACM Conference on Connected Health: Applications, Systems and Engineering Technologies (CHASE)(2022)
摘要
Traditional implementations of federated learning for preserving data privacy are unsuitable for longitudinal health data. To remedy this, we develop a federated enhanced fuzzy c-means clustering (FeFCM) algorithm that can identify groups of patients based on complex behavioral intervention responses. FeFCM calculates a global cluster model by incorporating data from multiple healthcare institutions without requiring patient observations to be shared. We evaluate FeFCM on simulated clusters as well as empirical data from four different dietary health studies in Massachusetts. Results find that FeFCM converges rapidly and achieves desirable clustering performance. As a result, FeFCM can promote pattern recognition in longitudinal health studies across hundreds of collaborating healthcare institutions while ensuring patient privacy.
更多查看译文
关键词
longitudinal,fuzzy,clustering,federated learning
AI 理解论文
溯源树
样例
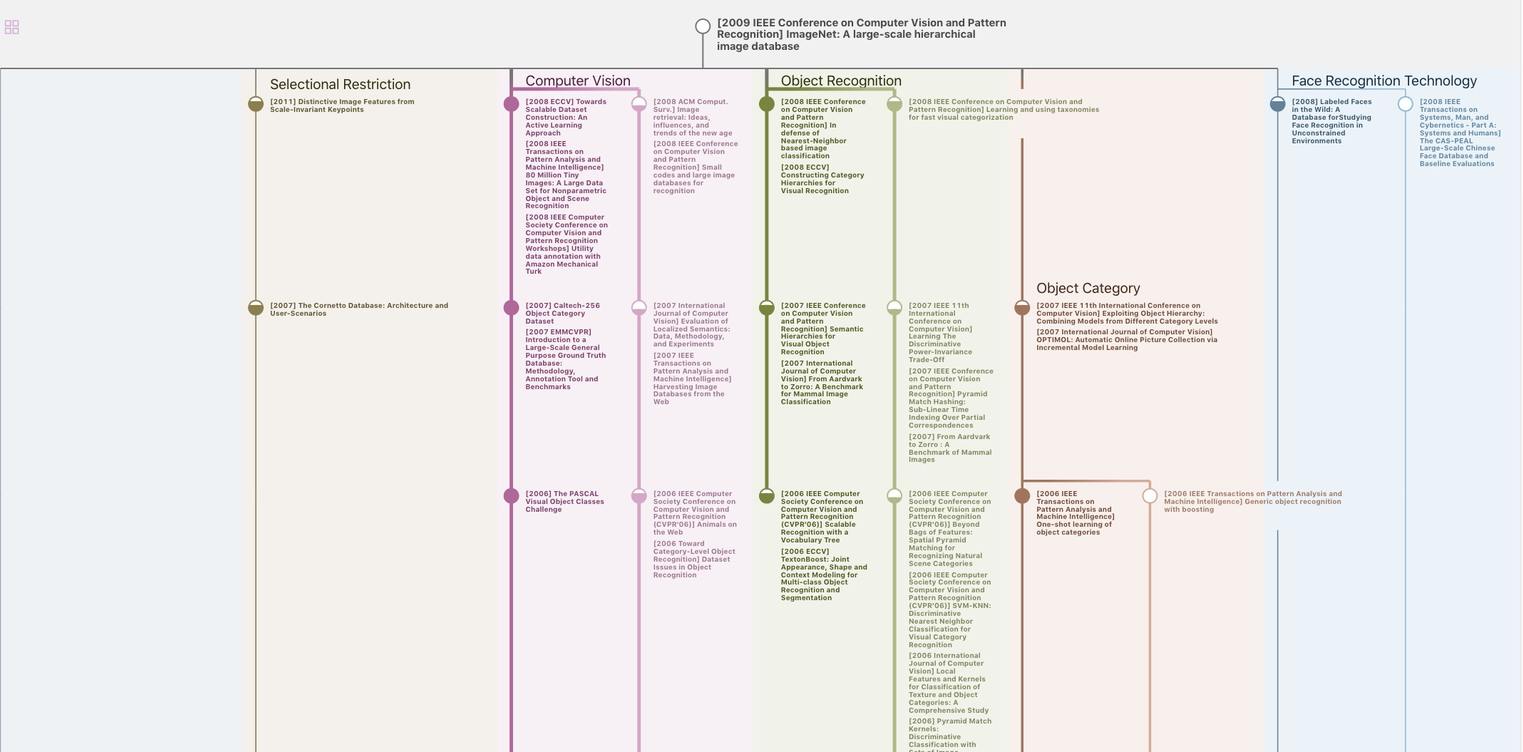
生成溯源树,研究论文发展脉络
Chat Paper
正在生成论文摘要