An Empirical Study of Training Mixture Generation Strategies on Speech Separation: Dynamic Mixing and Augmentation
2022 Asia-Pacific Signal and Information Processing Association Annual Summit and Conference (APSIPA ASC)(2022)
摘要
Deep learning has dramatically advanced speech separation (SS) in the past decade. Although advances in model architectures play an essential role in improving the separation performance, an efficient training strategy is also important. In this study, we investigate various strategies for training mixture generation in SS, considering that such strategies are likely essential in improving the generalization abilities of the trained models. More specifically, instead of using the vanilla training mixtures pre-generated by a given dataset, we remix clean source signals to generate more mixtures by using dynamic mixing (DM), which is an on-the-fly speech mixing strategy for model training. In addition, we combine DM with other data augmentation methods to further improve the separation performance. We analyze the effects of training data generation strategies for training sets at different scales and with various diversities. Evaluation results on multiple public datasets suggest that increasing the number of speech mixtures using DM with data augmentations is a very effective strategy for SS, especially for training sets with a limited number of clean sources.
更多查看译文
AI 理解论文
溯源树
样例
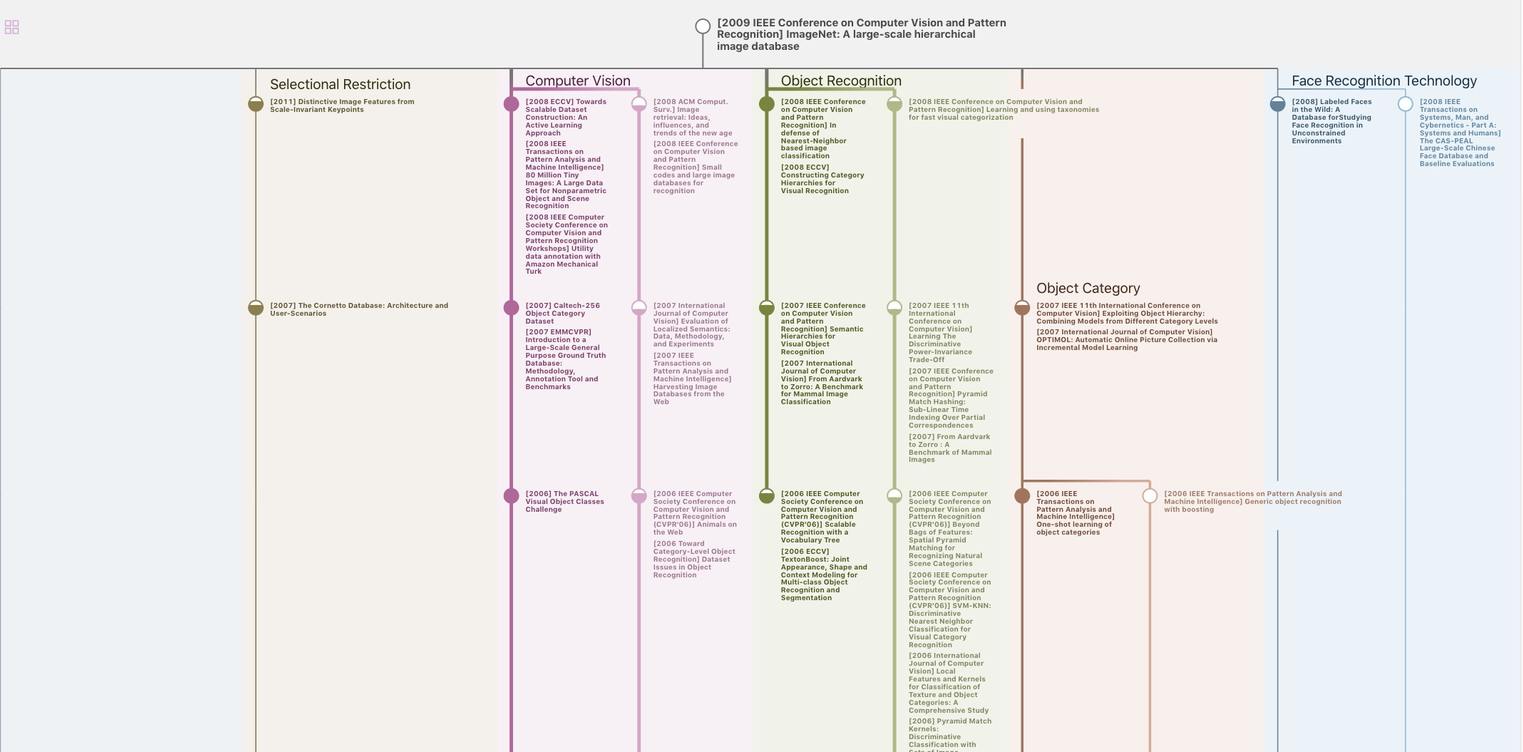
生成溯源树,研究论文发展脉络
Chat Paper
正在生成论文摘要