A multi-task learning approach for chemical process abnormity locations and fault classifications
Chemometrics and Intelligent Laboratory Systems(2023)
摘要
The fault classification of chemical processes based on supervised learning generally demands for enough labeled fault samples, which represents a big challenge for practical engineering issues. In the industrial field, when capturing an appearance of process abnormities, human operators usually take account of correlations of relevant variables so as to locate process variables associated with the abnormity before further classifying or diagnosing the corresponding faults. In this context, accurate abnormity localizations can be an effective help for classifying the fault type. Nowadays, deep learning based fault classifications have been extensively studied and widely circulated in the literature. However, the existing approaches rarely concern the supporting role of abnormity locations for fault classifications. Motivated by this observation, this paper proposes a multi-task learning (MTL) based convolutional neural network (CNN) model for concurrent abnormity locations and fault classifications for chemical processes. Specifically, fault samples are labeled with both abnormal variable locations and fault categories before constructing a multi-task learning CNN classifier performing an auxiliary task, abnormity locations, and a primary task, fault classifications. In addition, dynamic task weights are assigned to facilitate task contributions. This method can enjoy effectively reducing the necessary amounts of labeled fault samples in achieving satisfactory fault classification performances. Experiments are carried out on the TE process simulation platform and an industrial coal gasification plant along with comparisons with conventional fault classification methods, verifying the effectiveness of the proposed method.
更多查看译文
关键词
Multi-task learning,Fault classification,Abnormity location,Chemical process
AI 理解论文
溯源树
样例
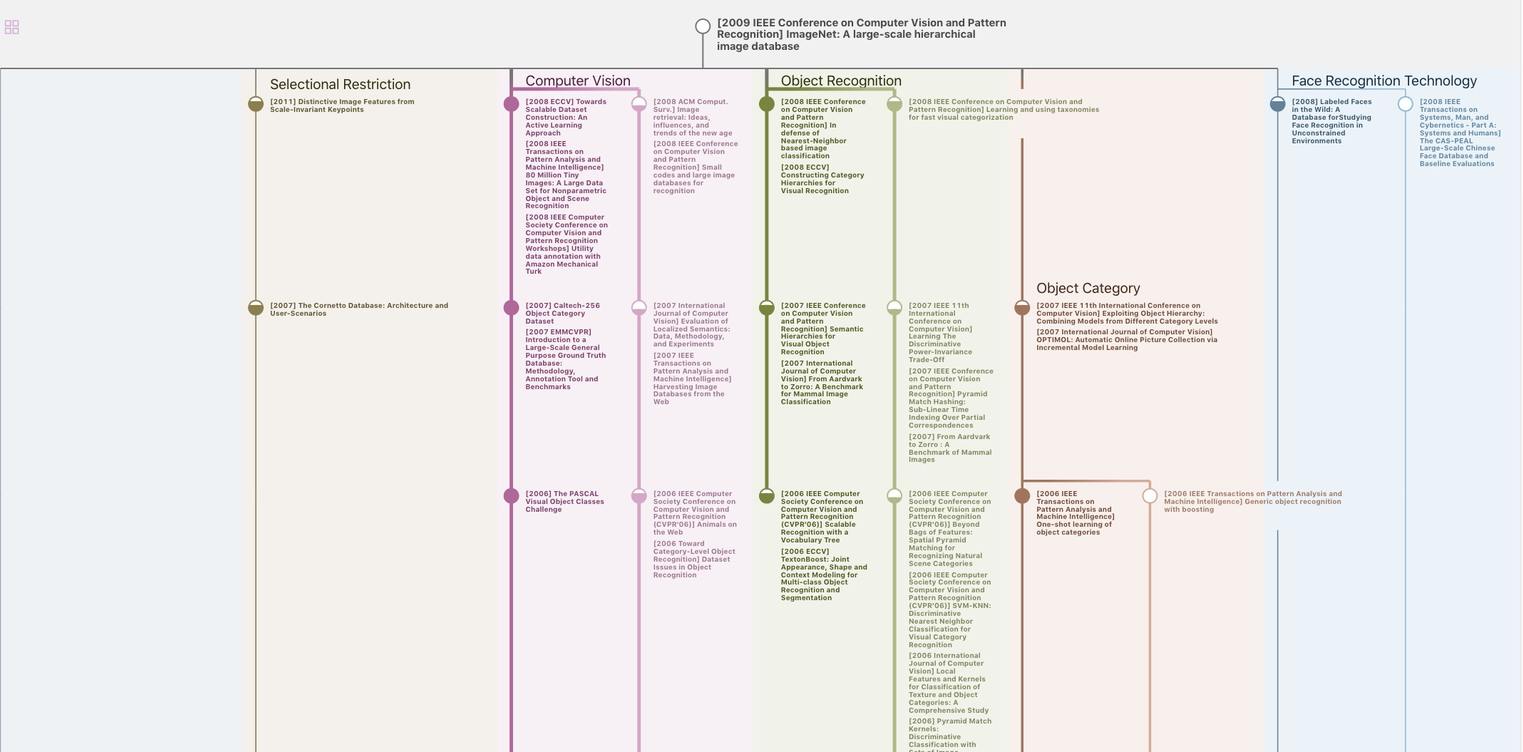
生成溯源树,研究论文发展脉络
Chat Paper
正在生成论文摘要