Matching calipers and the precision of index estimation
arXiv (Cornell University)(2023)
Abstract
This paper characterizes the precision of index estimation as it carries over into precision of matching. In a model assuming Gaussian covariates and making best-case assumptions about matching quality, it sharply characterizes average and worst-case discrepancies between paired differences of true versus estimated index values. In this optimistic setting, worst-case true and estimated index differences decline to zero if $p=o[n/(\log n)]$, the same restriction on model size that is needed for consistency of common index models. This remains so as the Gaussian assumption is relaxed to sub-gaussian, if in that case the characterization of paired index errors is less sharp. The formula derived under Gaussian assumptions is used as the basis for a matching caliper. Matching such that paired differences on the estimated index fall below this caliper brings the benefit that after matching, worst-case differences onan underlying index tend to 0 if $p = o\{[n/(\log n)]^{2/3}\}$. (With a linear index model, $p=o[n/(\log n)]$ suffices.) A proposed refinement of the caliper condition brings the same benefits without the sub-gaussian condition on covariates. When strong ignorability holds and the index is a well-specified propensity or prognostic score, ensuring in this way that worst-case matched discrepancies on it tend to 0 with increasing $n$ also ensures the consistency of matched estimators of the treatment effect.
MoreTranslated text
Key words
calipers,index estimation,matching,precision
AI Read Science
Must-Reading Tree
Example
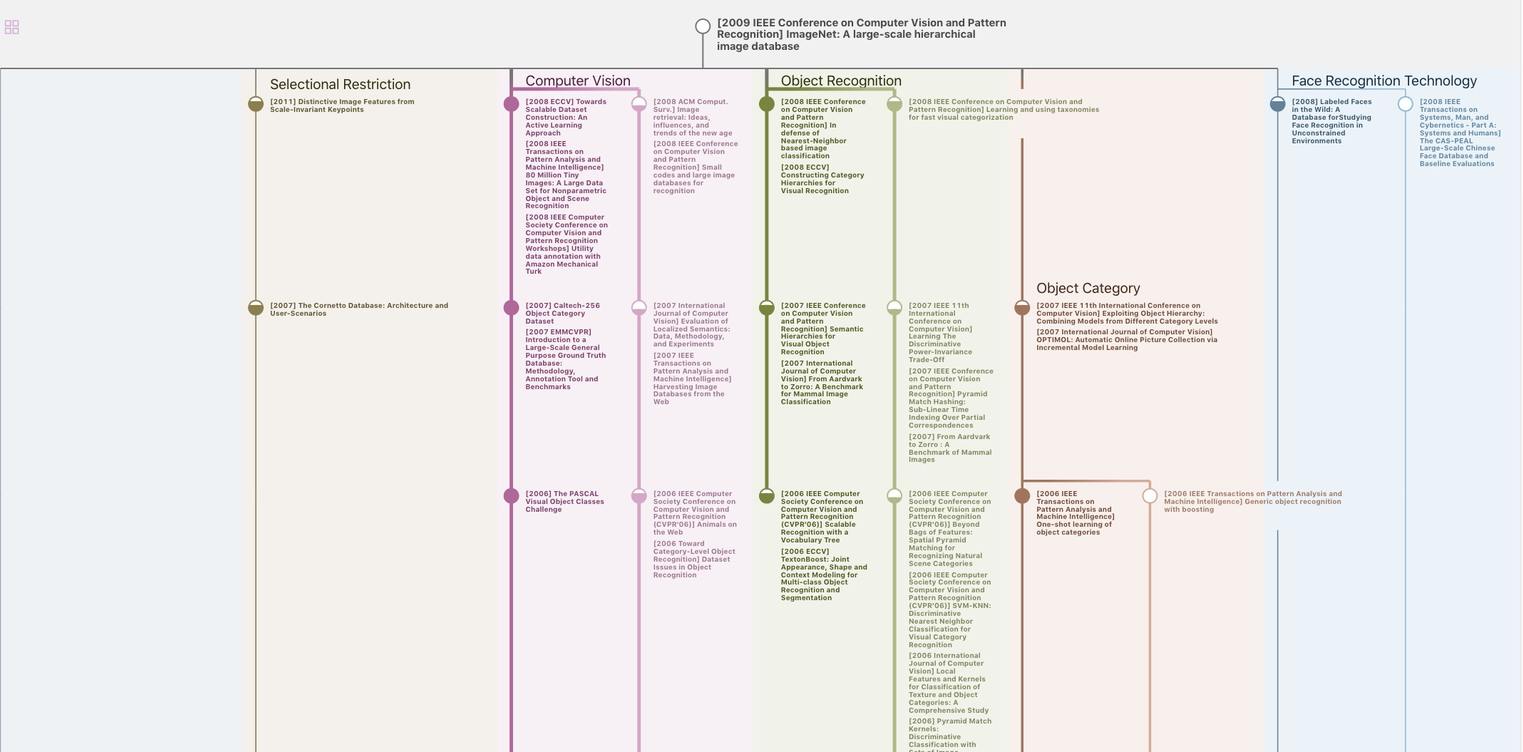
Generate MRT to find the research sequence of this paper
Chat Paper
Summary is being generated by the instructions you defined