Understanding the Complexity and Its Impact on Testing in ML-Enabled Systems
arxiv(2023)
摘要
Machine learning (ML) enabled systems are emerging with recent breakthroughs in ML. A model-centric view is widely taken by the literature to focus only on the analysis of ML models. However, only a small body of work takes a system view that looks at how ML components work with the system and how they affect software engineering for MLenabled systems. In this paper, we adopt this system view, and conduct a case study on Rasa 3.0, an industrial dialogue system that has been widely adopted by various companies around the world. Our goal is to characterize the complexity of such a largescale ML-enabled system and to understand the impact of the complexity on testing. Our study reveals practical implications for software engineering for ML-enabled systems.
更多查看译文
关键词
complexity,testing,systems,ml-enabled
AI 理解论文
溯源树
样例
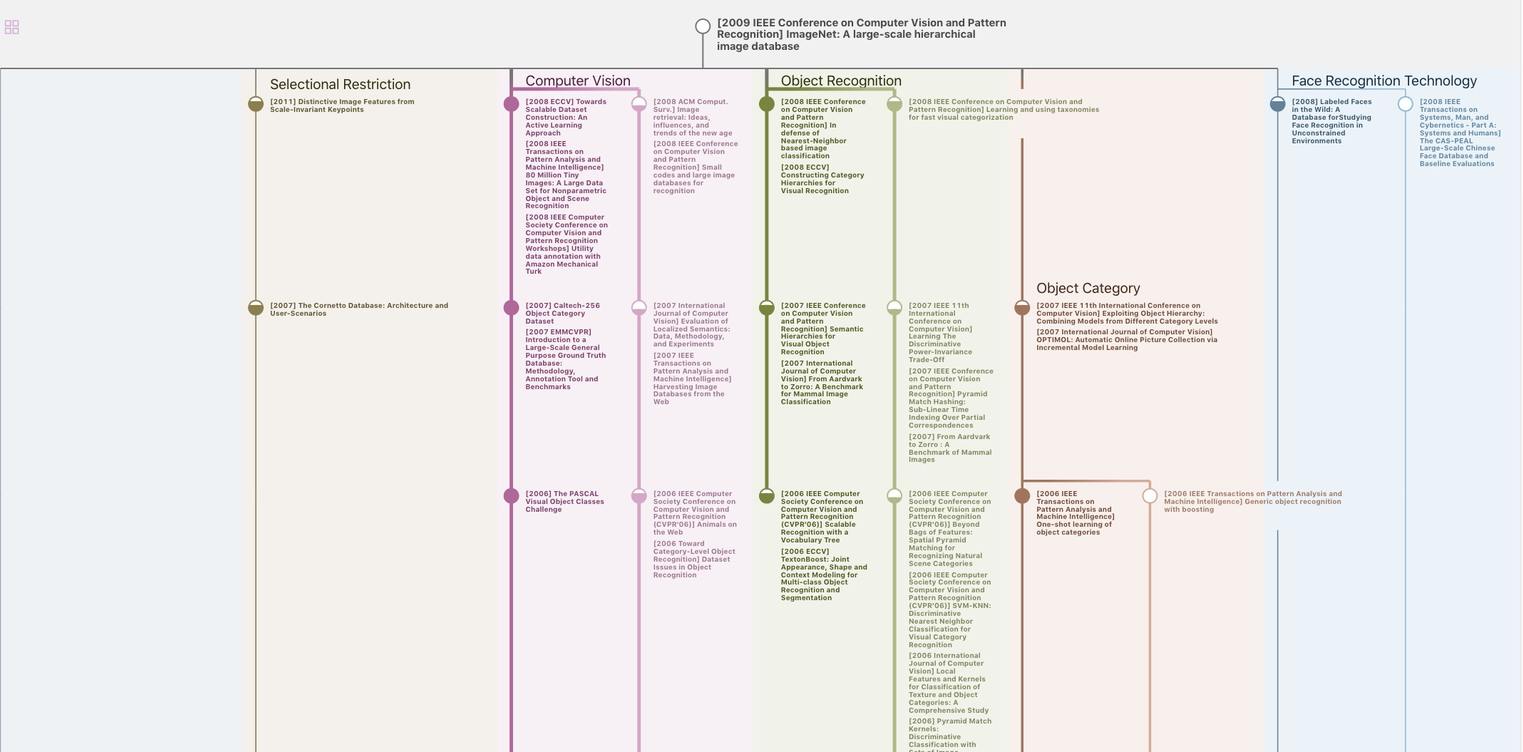
生成溯源树,研究论文发展脉络
Chat Paper
正在生成论文摘要