Fail-Safe: Securing Cyber-Physical Systems against Hidden Sensor Attacks
2022 IEEE Real-Time Systems Symposium (RTSS)(2022)
摘要
In Cyber-Physical Systems (CPS), integrating new technologies that interact with and control physical systems raises new security risks beyond the classical cyber security domain. These risks motivated many attack detectors that focus on the binary outcome. However, one pressing risk in CPS is hidden sensor attacks that are well-designed by powerful attackers who gained full knowledge of our systems and detector. The hidden attacks inject such a small malicious signal into sensor measurement that they can stay undetected but eventually lead to a significant deviation. Thus, to secure the CPS, we propose a detection framework to identify these sensor attacks that can drive the system's physical states to an unsafe state within a given period, even if they are not detected. First, we solve optimization problems to find the optimal hidden sensor attack that leads to the minimal distance to a pre-defined unsafe state region within an observation window for a given system and detector. Then, based on this algorithm, we perform offline profiling to search for a conditionally safe region, where the system states are guaranteed to be safe within the observation window as long as the detector does not raise any alerts. Finally, the framework can online discover potential hidden sensor attacks that endanger the system by checking if the current system state moves out of the region and raising a yellow alert. The evaluation shows that the optimal hidden sensor attack results in the minimum distance to unsafe, within a given observation window among existing hidden sensor attacks. We implemented our method on four linear simulators to show the effectiveness of our method. Additionally, we provided a discussion on the challenges of applying the proposed method to non-linear systems.
更多查看译文
关键词
hidden sensor attack,cyber-physical systems,detection
AI 理解论文
溯源树
样例
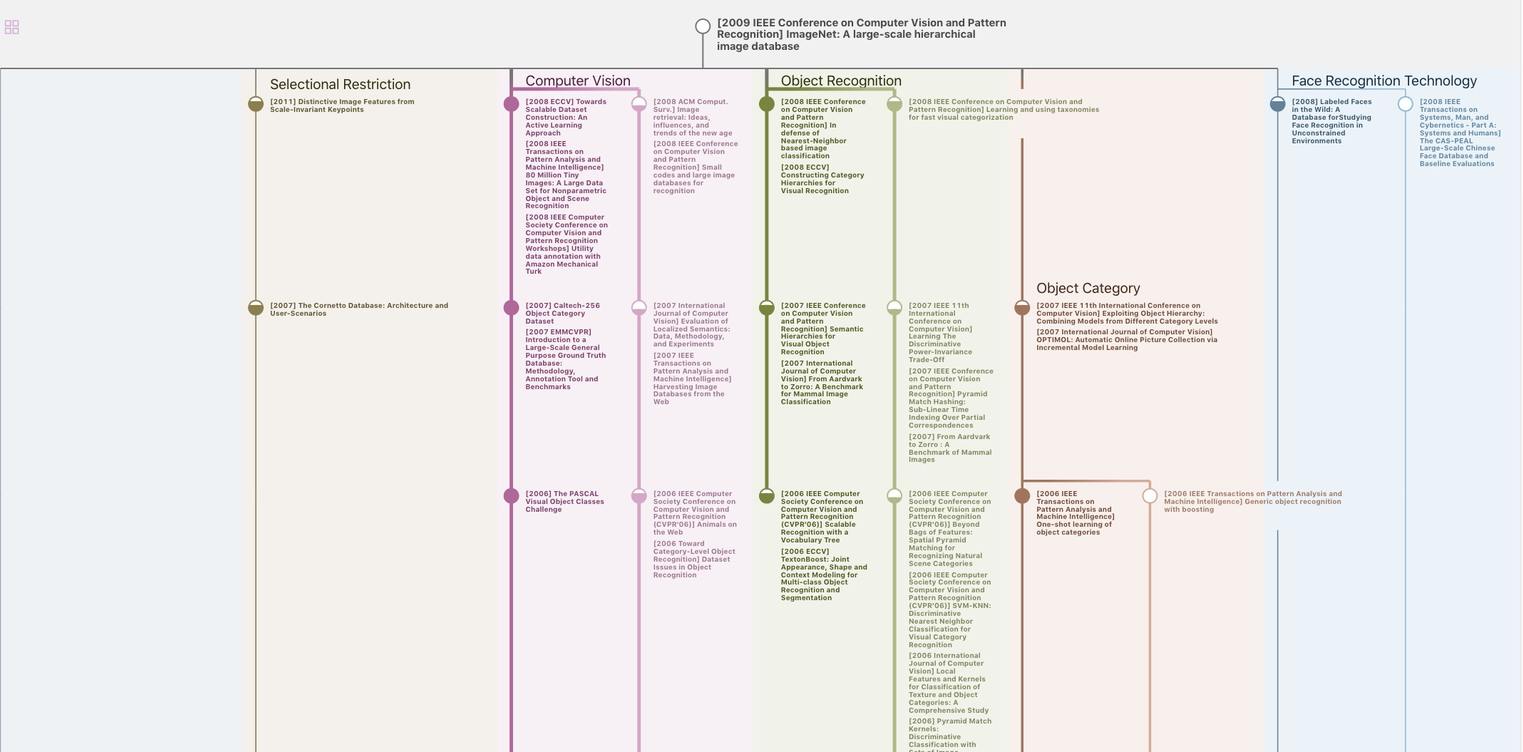
生成溯源树,研究论文发展脉络
Chat Paper
正在生成论文摘要