RIBoNN: Designing Robust In-Memory Binary Neural Network Accelerators
2022 IEEE International Test Conference (ITC)(2022)
摘要
RRAM crossbar-based accelerators show promise to execute compute intensive Deep Learning applications at the edge. For highly energy-constrained systems, Binary Neural Networks (BNNs) have gained momentum in recent times as the reduced precision alleviates the costs associated with storage, compute and communication. However, faults manifested in a unit bitcell of a RRAM crossbar-based accelerator may lead to drastic degradation in accuracy of the BNN, resulting in unintended system behavior. In this paper, we propose RIBoNN, a robust RRAM-based in-memory BNN accelerator, that consists of a 2T2R differential bitcell as the basic element of the crossbar. By leveraging the inherent characteristics of the proposed bitcell, RIBoNN is capable of achieving in-situ fault tolerance, thus circumventing the need to stall the deployed application for detection or diagnosis at the edge. RIBoNN, when evaluated on image-based datasets yields up to 96.57 % improvement in BNN classification accuracy, at a fault rate of 5 %; thereby demonstrating significant fault-tolerance over the state-of-the-art XNOR-RRAM BNN accelerator. Even though RiBoNN furnishes a negligible energy overhead of 2.62% over XNOR-RRAM, our proposed accelerator significantly reduces the inference latency by performing 24.4 % faster MAC operations with identical area footprint, while providing immense fault tolerance at the edge.
更多查看译文
关键词
BNN Accelerators,RRAM,Compute-in-Memory,Fault Tolerance
AI 理解论文
溯源树
样例
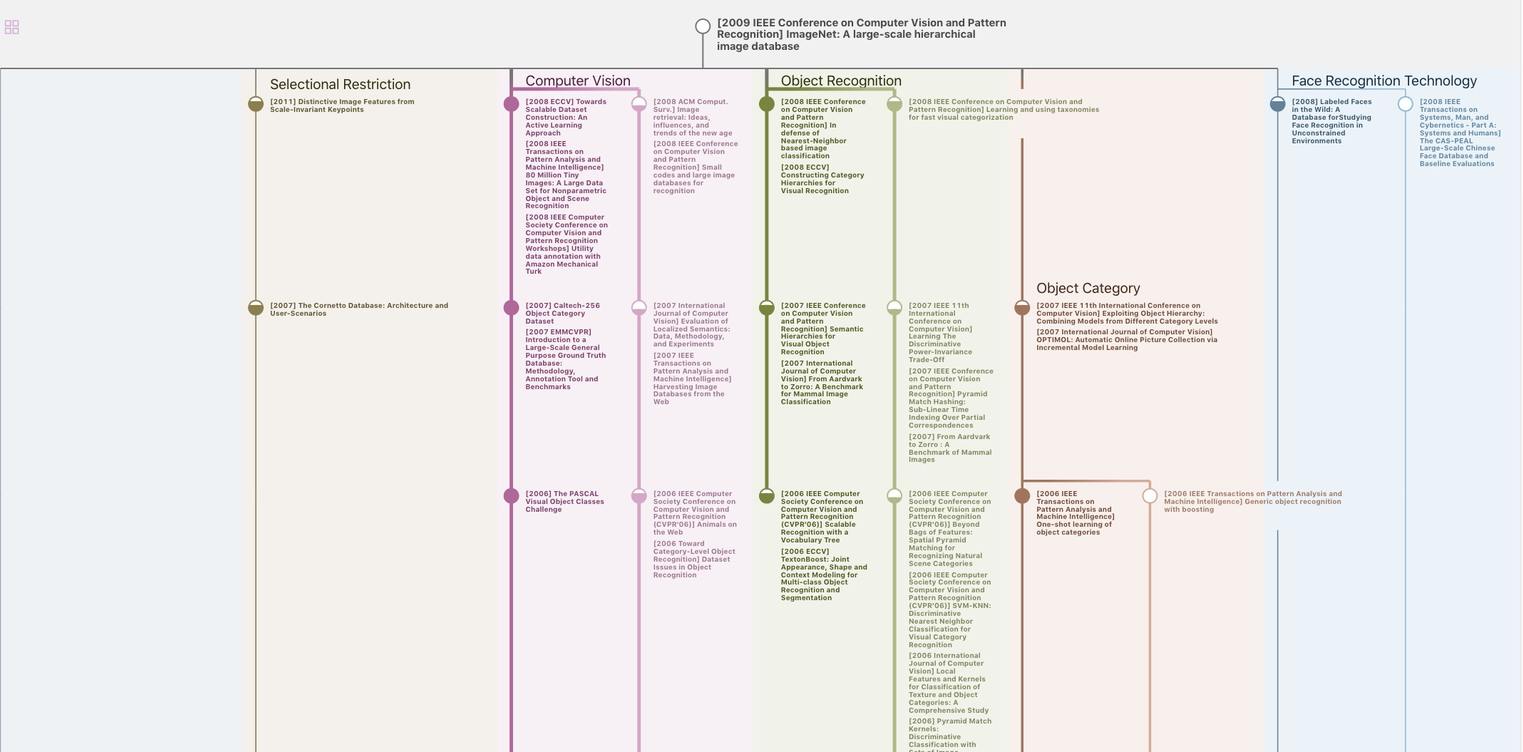
生成溯源树,研究论文发展脉络
Chat Paper
正在生成论文摘要