Knowledge extraction and performance improvement of Bi2Te3-based thermoelectric materials by machine learning
Materials Today Physics(2023)
摘要
Major advances in materials research often require serendipity and chemical intuition. Traditional trial-and-error-based experimental studies are becoming insufficient for designing novel high-performance materials because of staggering degree of freedom in materials' processing and composition. This work employed machine learning to aid the design of hot-extruded Bi2Te2.85Se0.15 bulk thermoelectric materials based on a small amount of in-house experimental data. Surprisingly, it was found that a combination of processing/composition design strategy with higher extrusion temperatures, more Cu dopants, and deficiency of Te, which is contradictory to experimental experiences, could enhance the materials’ figure of merit (ZT). Experimental validation demonstrated that such a processing/composition solution effectively suppressed formation of point defects, refined microstructure, and promoted evolution of a “fiber texture”, thus simultaneously improving thermoelectric and mechanical properties. The data-driven strategy breaks the rules of thumb in traditional experimental studies and extracts new knowledge to guide the design of high-performance Bi2Te3-based bulk thermoelectric materials.
更多查看译文
关键词
Bismuth telluride,Thermoelectric materials,Machine learning,Knowledge extraction,Extrusion
AI 理解论文
溯源树
样例
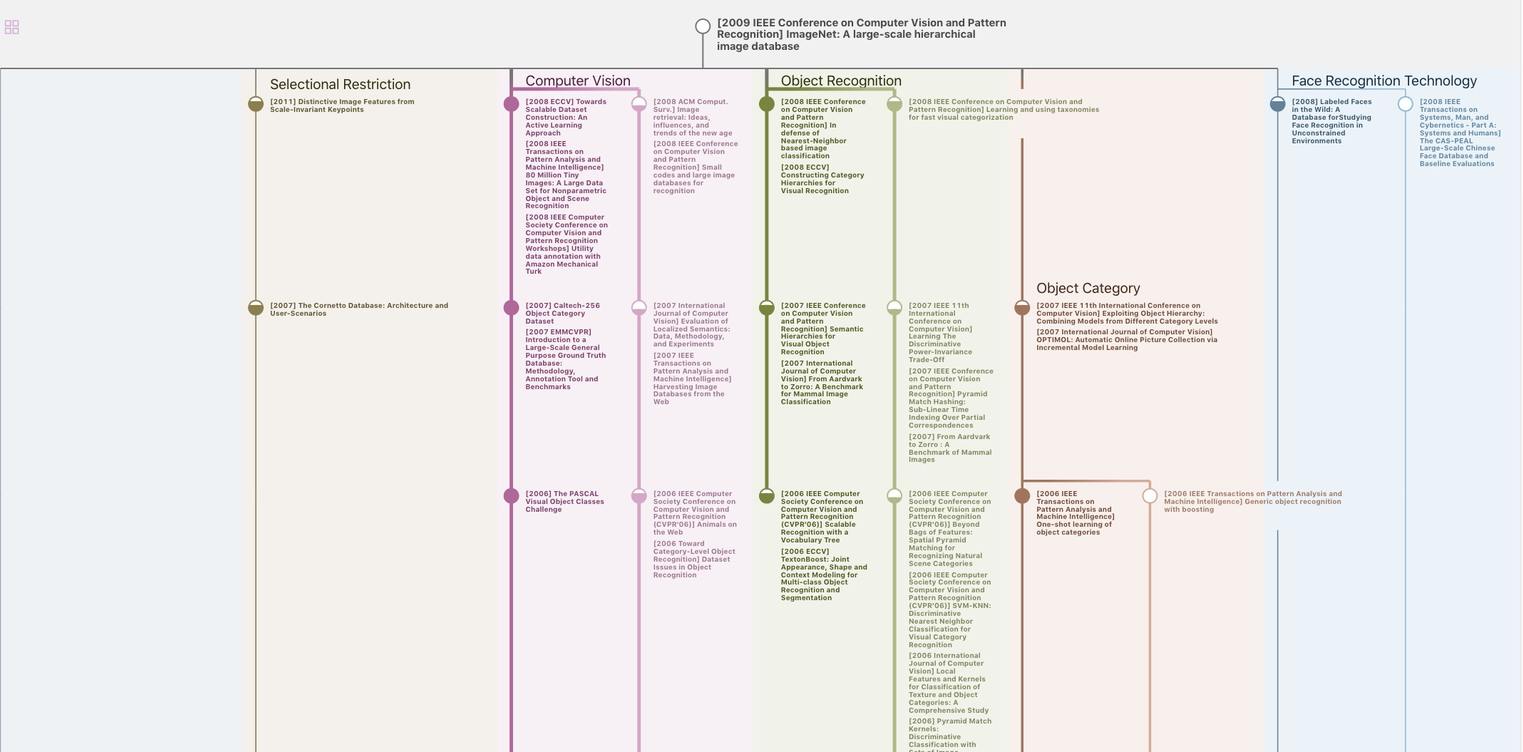
生成溯源树,研究论文发展脉络
Chat Paper
正在生成论文摘要