Prediction of water quality extremes with composite quantile regression neural network
Environmental Monitoring and Assessment(2023)
摘要
Water quality extremes, which water quality models often struggle to predict, are a grave concern to water supply facilities. Most existing water quality models use mean error functions to maximize the predictability of water quality mean value. This paper describes a composite quantile regression neural network (CQRNN) model, which simultaneously estimates non-crossing regression quantiles by minimizing the composite quantile regression error function. This method can improve the prediction of extremes. This paper evaluates the performance of CQRNN for predicting extreme values of turbidity and total organic carbon (TOC) and compares with quantile regression (QR), linear regression (LR), and k -nearest neighbors (KNN) in an application to the Hetch Hetchy Regional Water System, which is the primary water supply for San Francisco, CA. CQRNN is superior to QR, LR, and KNN for predicting the mean trend and extremes of turbidity and TOC, especially for the non-Gaussian turbidity data. The performance of CQRNN is the most stable relative to other methods over different training sample sizes.
更多查看译文
关键词
Composite quantile regression neural network,Machine learning,Water quality,Water supply system
AI 理解论文
溯源树
样例
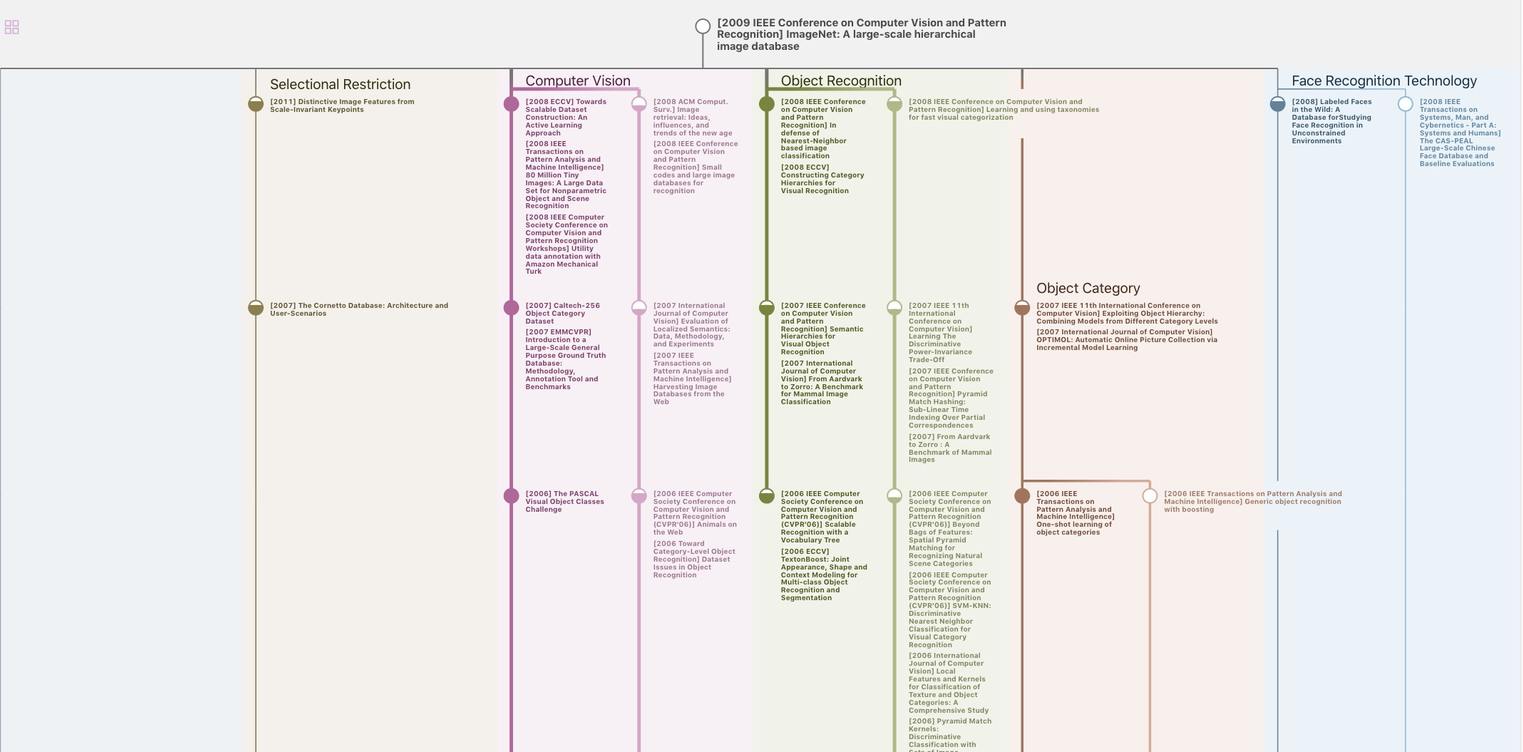
生成溯源树,研究论文发展脉络
Chat Paper
正在生成论文摘要