Seabed sediments classification based on side-scan sonar images using dimension-invariant residual network
Applied Ocean Research(2023)
摘要
Seabed sediment classification has important applications in ocean engineering and submarine pipeline design. This paper proposed the dimension-invariant residual network to improve the seabed sediments classification accuracy. The dimension of each channel of the feature extracted from the dimension-invariant residual block is the same as the original image. Compared with down-sampling, each feature vector has more feature parameters, which can improve the accuracy of seabed sediments classification. Shortcut connections were introduced in the proposed network, which can make the network easier to optimize and achieve good performance by using only a not very deep network. It also has a lower complexity compared to powerful classifiers like Transformer and has a better performance in small-scale seabed sediment classification. And to improve the accuracy of seabed sediment classification without consuming too much time for training the classifier, the network parameters optimization method was proposed in this paper. Recording the classification accuracy and training time of the network with these different parameters. Determining the parameters of the network by the proposed optimization method. The proposed classification model and optimization method were validated by using the side-scan sonar images, acquired from the area around the Shandong Peninsula of China. The layers and convolutional kernel sizes were determined to be 8 and 3, respectively. The classification accuracy of overall, rock, sand, and mud of the dimension-invariant residual network model were 97.83%, 94.04%, 97.80%, and 98.02% respectively. In comparison with state-of-the-art classifiers, the experimental results demonstrated that the proposed classification model achieved better performance, and the network parameters optimization method enables the network to achieve satisfactory classification accuracy while spending less time. The proposed classification model and network parameters optimization method show great potential in seabed sediments classification, which can also be extended to the task of underwater target recognition in future research.
更多查看译文
关键词
Subsea engineering,Dimension-invariant residual network,Convolutional neural network,Seabed sediments classification,Side-scan sonar images,Network parameters optimization
AI 理解论文
溯源树
样例
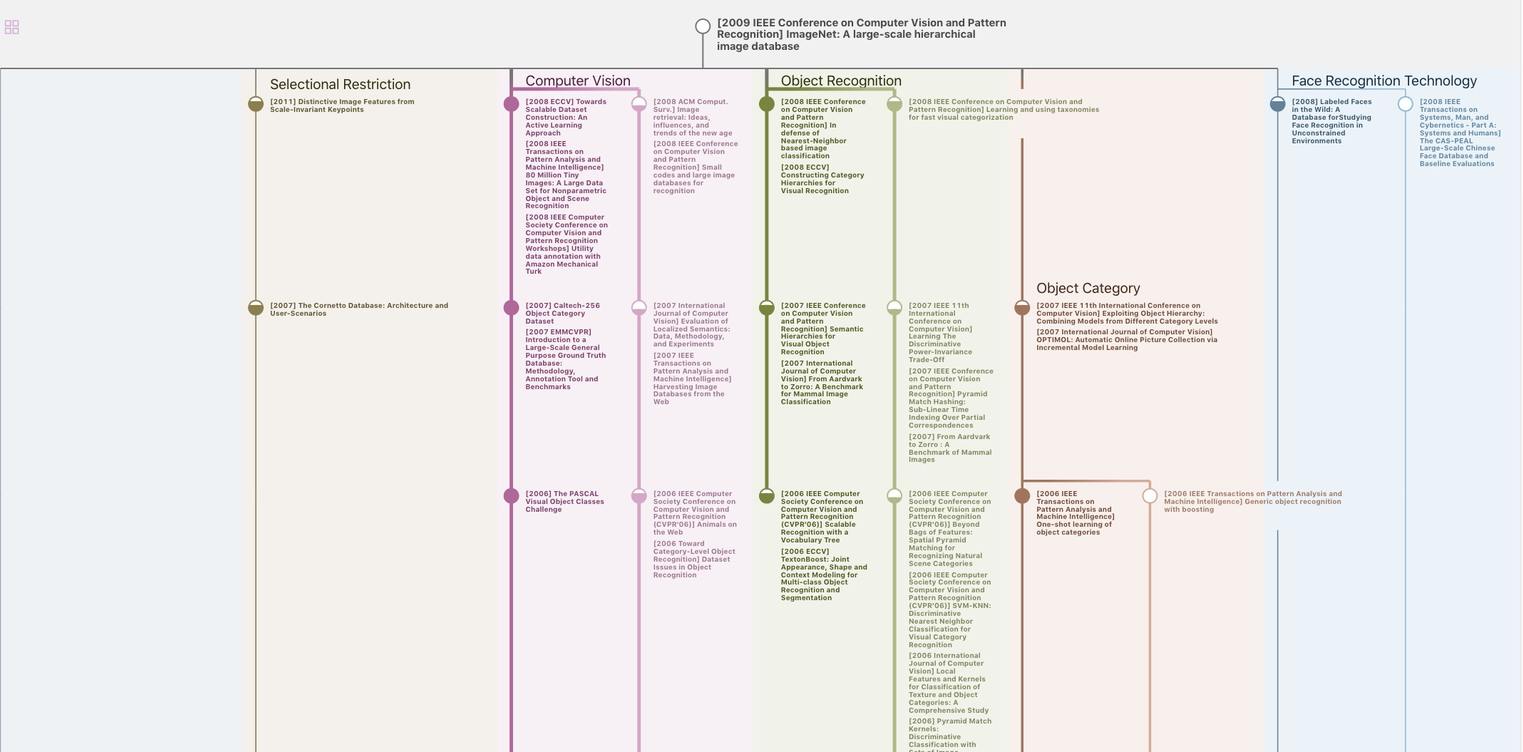
生成溯源树,研究论文发展脉络
Chat Paper
正在生成论文摘要