Granger causality test for heteroskedastic and structural-break time series using generalized least squares
arxiv(2023)
摘要
This paper proposes a novel method (GLS Granger test) to determine causal relationships between time series based on the estimation of the autocovariance matrix and generalized least squares. We show the effectiveness of proposed autocovariance matrix estimator (the sliding autocovariance matrix) and we compare the proposed method with the classical Granger F-test with via a synthetic dataset and a real dataset composed by cryptocurrencies. The simulations show that the proposed GLS Granger test captures causality more accurately than Granger F-tests in the cases of heteroskedastic or structural-break residuals. Finally, we use the proposed method to unravel unknown causal relationships between cryptocurrencies.
更多查看译文
关键词
structural-break
AI 理解论文
溯源树
样例
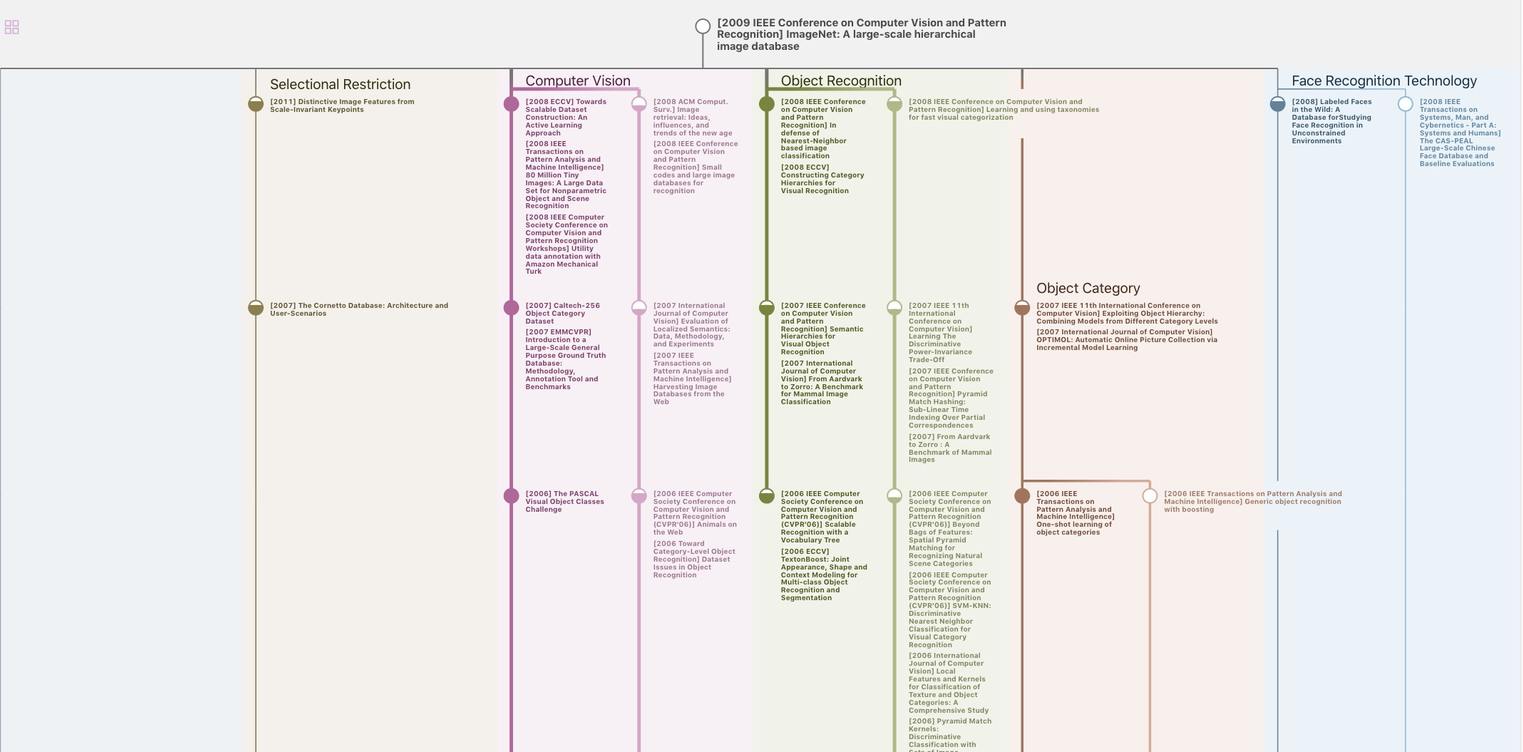
生成溯源树,研究论文发展脉络
Chat Paper
正在生成论文摘要