Forklens: Accurate Weak-Lensing Shear Measurement with Deep Learning
ASTRONOMY & ASTROPHYSICS(2024)
关键词
gravitation,gravitational lensing: weak,methods: data analysis,cosmology: observations
AI 理解论文
溯源树
样例
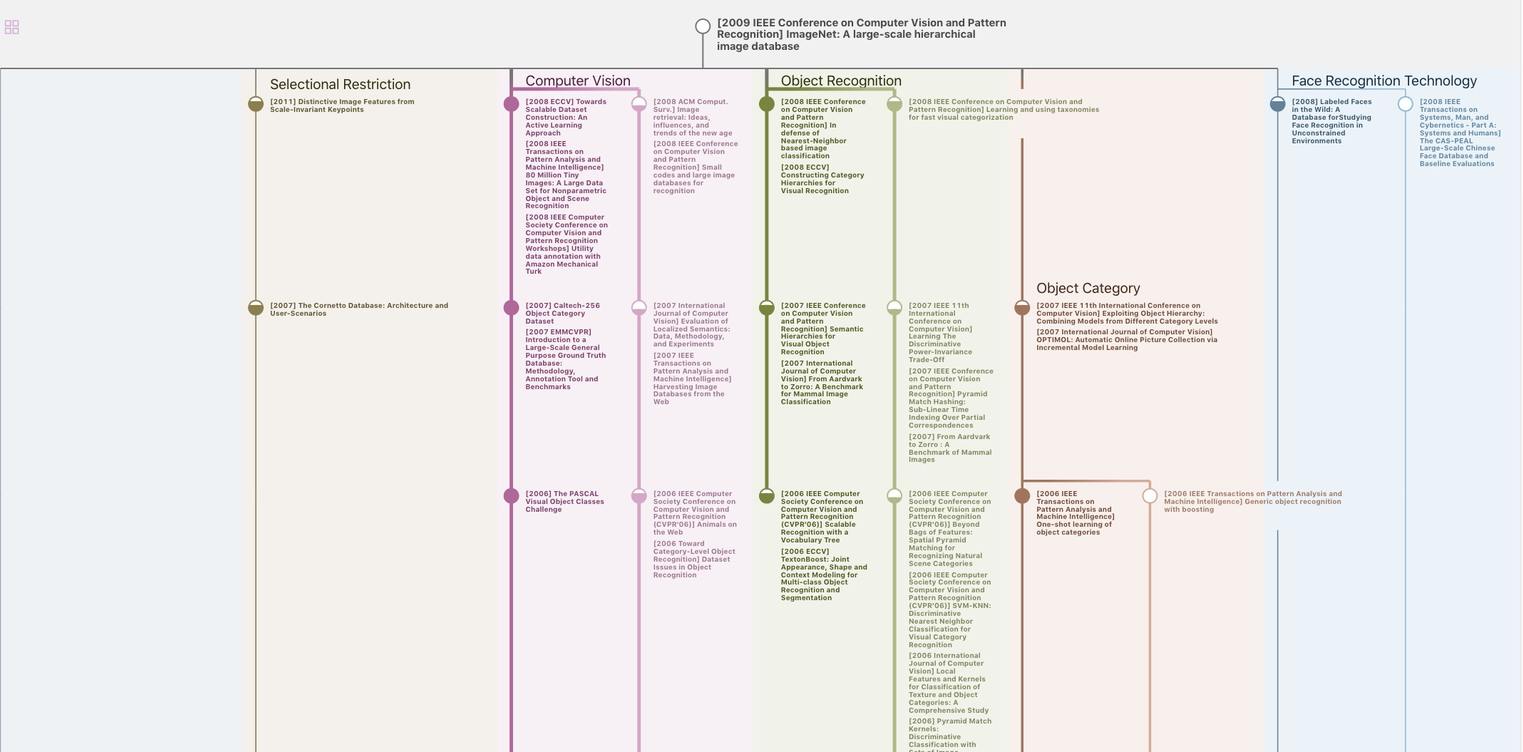
生成溯源树,研究论文发展脉络
Chat Paper
正在生成论文摘要
ASTRONOMY & ASTROPHYSICS(2024)