An enhanced gradient-tracking bound for distributed online stochastic convex optimization
SIGNAL PROCESSING(2024)
摘要
Gradient-tracking (GT) based decentralized methods have emerged as an effective and viable alternative method to decentralized (stochastic) gradient descent (DSGD) when solving distributed online stochastic optimization problems. Initial studies of GT methods implied that GT methods have worse network dependent rate than DSGD, contradicting experimental results. This dilemma has recently been resolved, and tighter rates for GT methods have been established, which improves upon DSGD.In this work, we establish more enhanced rates for GT methods under the online stochastic convex settings. We present an alternative approach for analyzing GT methods for convex problems and over static graphs. When compared to previous analyses, this approach allows us to establish enhanced network dependent rates.
更多查看译文
关键词
Distributed stochastic optimization,Decentralized learning,Gradient-tracking,Adapt-then-combine
AI 理解论文
溯源树
样例
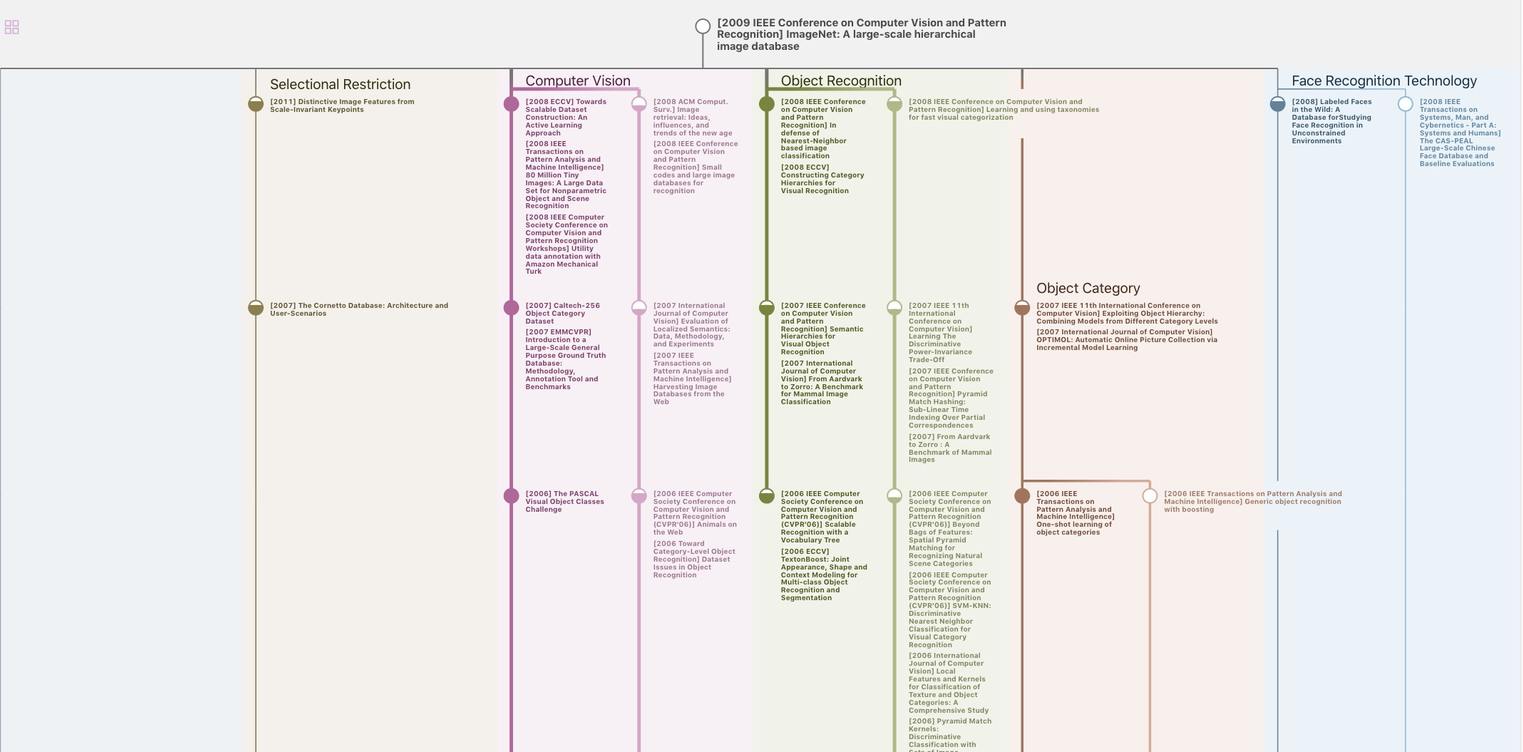
生成溯源树,研究论文发展脉络
Chat Paper
正在生成论文摘要