Deep Learning for Segmentation of Critical Electrical Infrastructure from Vehicle-Based Images
2022 IEEE Electrical Power and Energy Conference (EPEC)(2022)
摘要
Regular monitoring of critical electrical infrastructure is a necessary but laborious task to ensure infrastructure resilience. Typically, a worker must perform the inspection on site, following a checklist. The advent of highly instrumented autonomous vehicles provides an opportunity to automate inspections through repurposing of sensors typically used for navigation. Furthermore, the high frequency of data capture from passing cars can provide unprecedented temporal resolution for monitoring critical infrastructure. Motivated by this opportunity, we here evaluate the state of the art in deep learning algorithms for segmentation of critical electrical infrastructure from ground-based image, which differs from other studies that have largely focused on aerial images. Google Street View (GSV) images are used as a proxy for images captured by vision-based autonomous vehicles to evaluate two state-of-the-art models. ACU-Net, originally trained on power line segmentation from unmanned aerial vehicle (UAV) imagery, is here adapted for use on ground-based imagery. Similarly, HRNet-OCR, a scene segmentation model for ground-based imagery, is used to simultaneously capture both power utility poles and powerlines. This study demonstrates that models trained exclusively on wires or power poles can be successfully adapted for a segmentation of the complete critical electrical infrastructure as a step towards fully automated inspection and increased infrastructure resilience. Wire and pole annotations from our GSV images are shared here.
更多查看译文
关键词
critical electrical infrastructure,deep learning,semantic segmentation,automated inspection,powerline,power utility pole,ground-based images,Google Street View
AI 理解论文
溯源树
样例
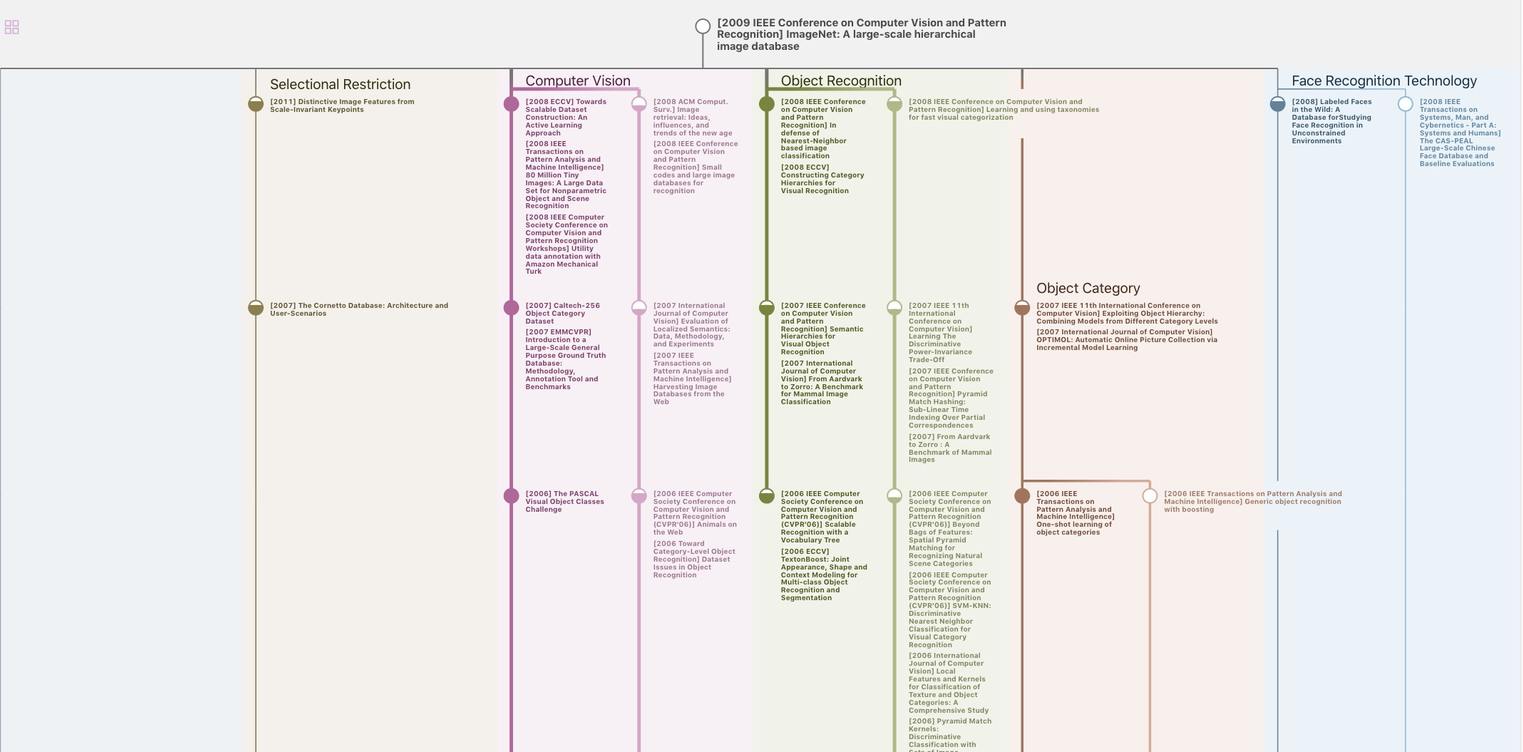
生成溯源树,研究论文发展脉络
Chat Paper
正在生成论文摘要