A Robust Data-Driven Process Modeling Applied to Time-Series Stochastic Power Flow
IEEE TRANSACTIONS ON POWER SYSTEMS(2024)
摘要
In this paper, we propose a robust data-driven process model whose hyperparameters are robustly estimated using the Schweppe-type generalized maximum likelihood estimator. The proposed model is trained on recorded time-series data of voltage phasors and power injections to perform a time-series stochastic power flow calculation. Power system data are often corrupted with outliers caused by large errors, fault conditions, power outages, and extreme weather, to name a few. The proposed model downweights vertical outliers and bad leverage points in the measurements of the training dataset. The weights used to bound the influence of the outliers are calculated using projection statistics, which are a robust version of Mahalanobis distances of the time series data points. The proposed method is demonstrated on the IEEE 33-Bus power distribution system and a real-world unbalanced 240-bus power distribution system heavily integrated with renewable energy sources. Our simulation results show that the proposed robust model can handle up to 25% of outliers in the training data set.
更多查看译文
关键词
Time-series stochastic power flow,robust process modeling,robust mahalanobis distances,generalized maximum likelihood estimator,outlier detection and identification
AI 理解论文
溯源树
样例
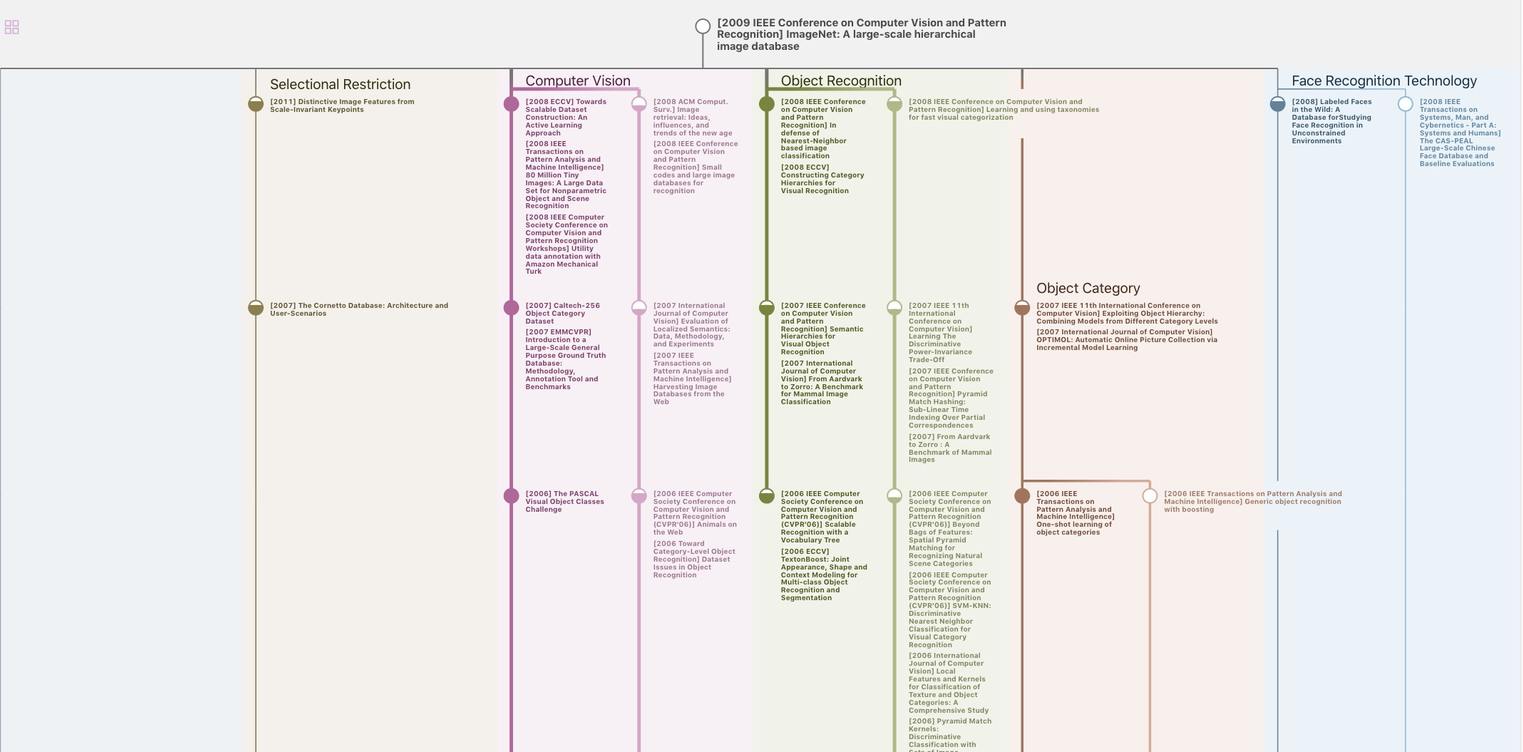
生成溯源树,研究论文发展脉络
Chat Paper
正在生成论文摘要