MR Elastography with Optimization-Based Phase Unwrapping and Traveling Wave Expansion-based Neural Network (TWENN)
arXiv (Cornell University)(2023)
Abstract
Magnetic Resonance Elastography (MRE) can characterize biomechanical properties of soft tissue for disease diagnosis and treatment planning. However, complicated wavefields acquired from MRE coupled with noise pose challenges for accurate displacement extraction and modulus estimation. Here we propose a pipeline for processing MRE images using optimization-based displacement extraction and Traveling Wave Expansion-based Neural Network (TWENN) modulus estimation. Phase unwrapping and displacement extraction were achieved by optimization of an objective function with Dual Data Consistency (Dual-DC). A complex-valued neural network using displacement covariance as input has been constructed for the estimation of complex wavenumbers. A model of traveling wave expansion is used to generate training datasets with different levels of noise for the network. The complex shear modulus map is obtained by a fusion of multifrequency and multidirectional data. Validation using images of brain and liver simulation demonstrates the practical value of the proposed pipeline, which can estimate the biomechanical properties with minimum root-mean-square-errors compared with state-of-the-art methods. Applications of the proposed method for processing MRE images of phantom, brain, and liver show clear anatomical features and that the pipeline is robust to noise and has a good generalization capability.
MoreTranslated text
Key words
Magnetic resonance elastography,modulus estimation,neural network,traveling waves,phase unwrapping
AI Read Science
Must-Reading Tree
Example
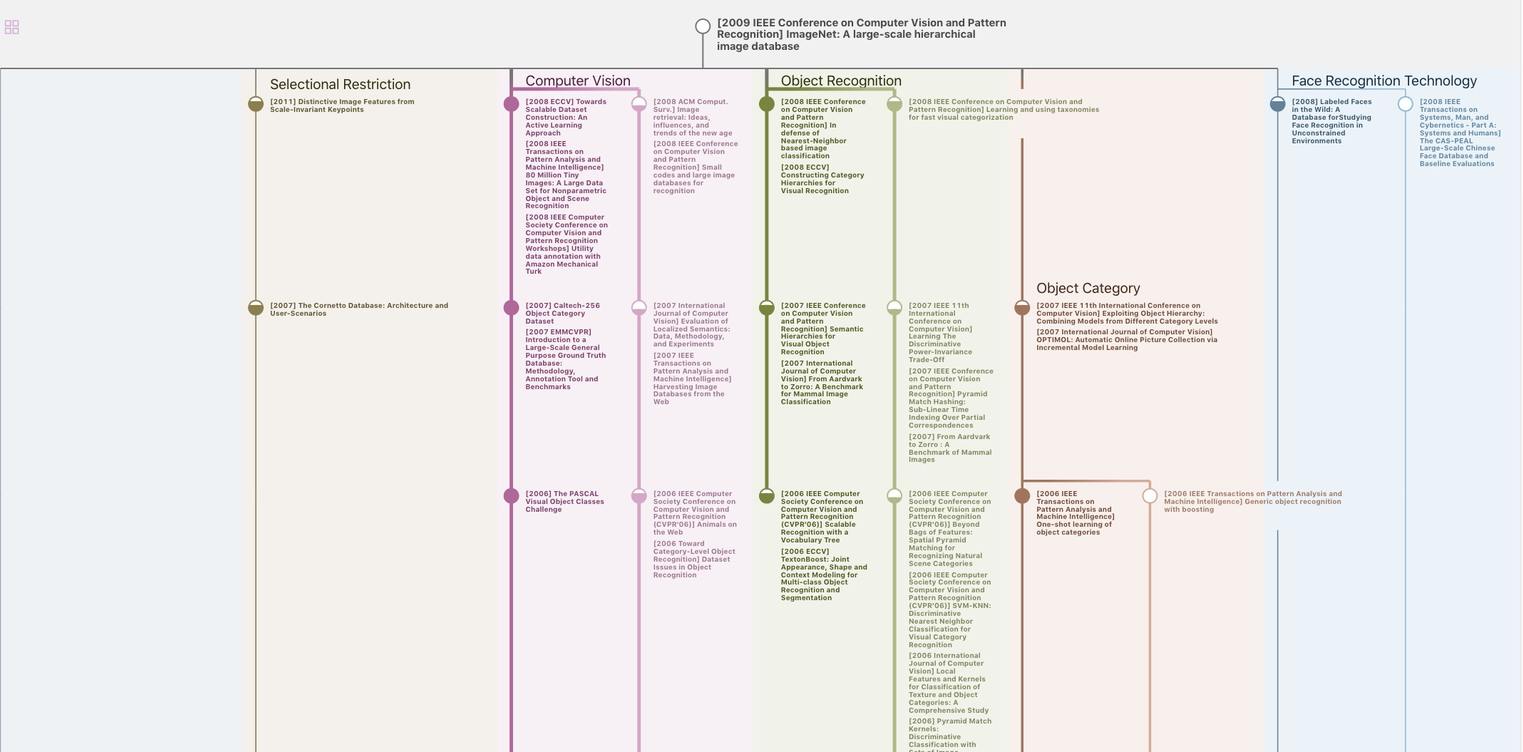
Generate MRT to find the research sequence of this paper
Chat Paper
Summary is being generated by the instructions you defined