Anchor3DLane: Learning To Regress 3D Anchors for Monocular 3D Lane Detection
CVPR 2023(2023)
摘要
Monocular 3D lane detection is a challenging task due to its lack of depth information. A popular solution is to first transform the front-viewed (FV) images or features into the bird-eye-view (BEV) space with inverse perspective mapping (IPM) and detect lanes from BEV features. However, the reliance of IPM on flat ground assumption and loss of context information make it inaccurate to restore 3D information from BEV representations. An attempt has been made to get rid of BEV and predict 3D lanes from FV representations directly, while it still underperforms other BEV-based methods given its lack of structured representation for 3D lanes. In this paper, we define 3D lane anchors in the 3D space and propose a BEV-free method named Anchor3DLane to predict 3D lanes directly from FV representations. 3D lane anchors are projected to the FV features to extract their features which contain both good structural and context information to make accurate predictions. In addition, we also develop a global optimization method that makes use of the equal-width property between lanes to reduce the lateral error of predictions. Extensive experiments on three popular 3D lane detection benchmarks show that our Anchor3DLane outperforms previous BEV-based methods and achieves state-of-the-art performances. The code is available at: https://github.com/tusen-ai/Anchor3DLane.
更多查看译文
关键词
Autonomous driving
AI 理解论文
溯源树
样例
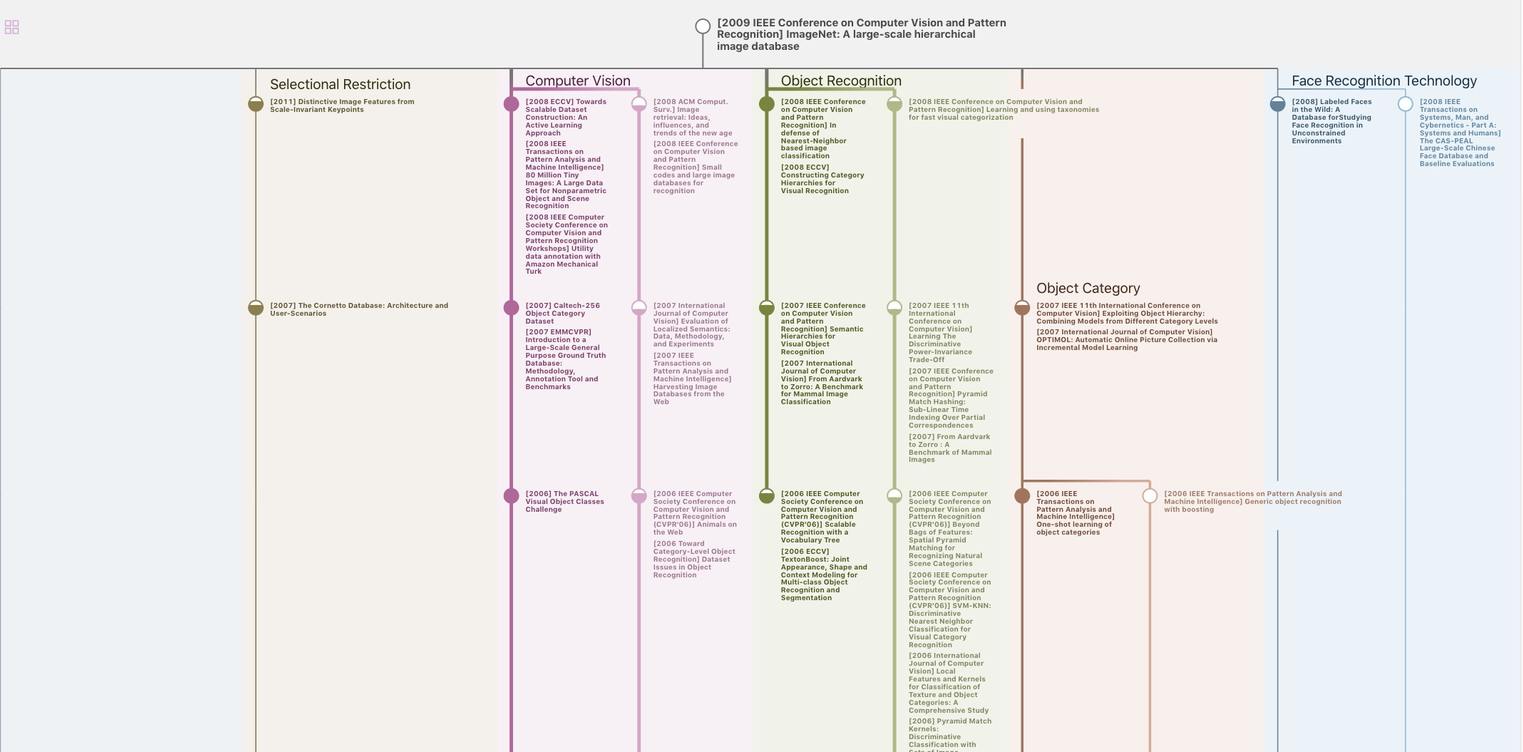
生成溯源树,研究论文发展脉络
Chat Paper
正在生成论文摘要