EnsDTI-kinase: Web-server for Predicting Kinase-Inhibitor Interactions with Ensemble Computational Methods and Its Applications
biorxiv(2023)
摘要
Kinase inhibitors are a major category of drugs. Experimental panel assay protocols are routinely used as a standard procedure to evaluate the efficiency and selectivity of a drug candidate to target kinase. However, current kinase panel assays are time-consuming and expensive. In addition, the panel assay protocols neither provide insights on binding sites nor allow experiments on mutated sequences or newly-characterized kinases. Existing virtual screening or docking simulation technologies require extensive computational resources, thus it is not practical to use them for the panel of kinases. With rapid advances in machine learning and deep learning technologies, a number of DTI tools have been developed over the years. However, these methods are yet to achieve prediction accuracies at the level of practical use. In addition, the performances of current DTI tools vary significantly depending on test sets. In this case, an ensemble model can be used to improve and stabilize DTI prediction accuracies. In this work, we propose an ensemble model, EnsDTI-kinase, that integrates eight existing machine learning and deep learning models into a unified model deployed as a web-server. Upon submission of a compound SMILES string, potential target kinases are automatically predicted and evaluated on the web-server. Importantly, EnsDTI-kinase is a computational platform where newly developed DTI tools can be easily incorporated without modifying core components so that its DTI prediction quality can improve over time.Besides, many useful functionalities are provided on our platform for users to further investigate predicted DTI: it allows confidence experiments by changing the amino acid (AA) at a specific position in a kinase sequence, named in-silico mutagenesis, to investigate the effect of AA changes in binding affinity; it predicts kinase sequential regions where the query compound likely binds to by slidingly masking the sequence of selected kinases so that confidence in the predicted binding sites can be evaluated. Our model was evaluated in three experimental settings using four independent datasets, and showed accuracy of 0.82 compared to the average accuracy of 0.69 from five deep learning methods on the ChEMBL dataset. It achieved average selectivity of 0.95 within kinase families such as TK, CAMK and STE. For 8 out of 17 recent drugs, our model successfully predicted their interactions with 404 proteins at average accuracy of 0.82.
### Competing Interest Statement
The authors have declared no competing interest.
更多查看译文
关键词
ensemble computational methods,ensdti-kinase,web-server,kinase-inhibitor
AI 理解论文
溯源树
样例
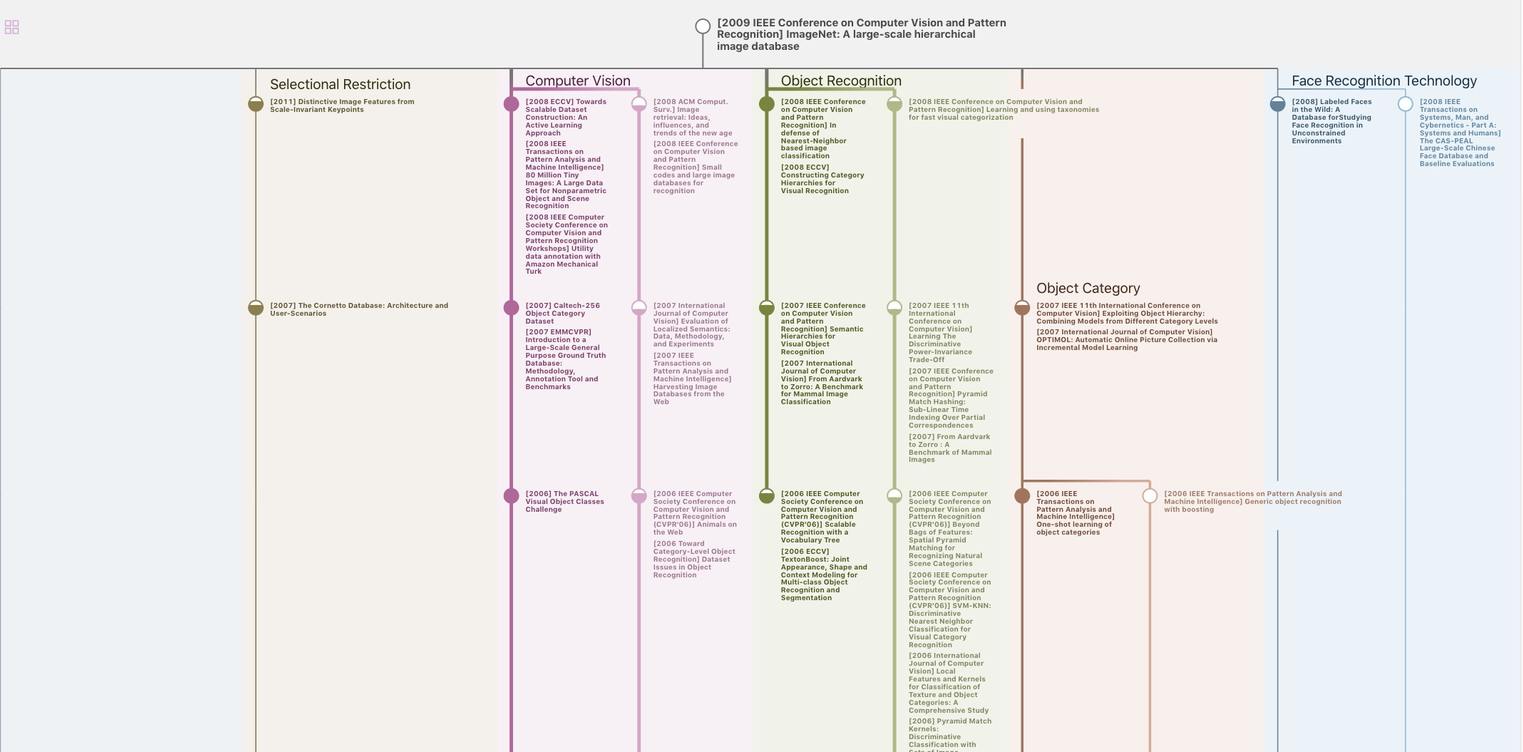
生成溯源树,研究论文发展脉络
Chat Paper
正在生成论文摘要