Predicting input validation vulnerabilities based on minimal SSA features and machine learning
JOURNAL OF KING SAUD UNIVERSITY-COMPUTER AND INFORMATION SCIENCES(2022)
摘要
Structured Query Language injection (SQLi) and Cross-Site Scripting (XSS) are the most renowned kinds of input validation vulnerabilities. Of late, vulnerability prediction models based on machine learning have been gaining acceptance in the domain of Web security. Such models offer an easy and effective way of dealing with web application security concerns. However, most of them, in particular, rely on complex graphs generated from source code or regex patterns based on expert knowledge. This paper proposed a method for extracting features from source code and predicting input validation vulnerabilities using machine learning algorithms. The proposed method can extract all features related to the flow of vulnerabilities among the programs and remove the features that are irrelevant to the vulnerability flow. In addition, each vulnerability's context has been assigned, providing additional data for our model to use in learning about the vulnerability context. Compared to other related methods, the feature extraction method proposed in this paper has been found to have high reusability and better performance. The best model related to the LSTM classifier had a 98.1% recall rate, a 97.9% precision, an accuracy of 98.67%, and a 99.03% area under the curve (AUC) in the test dataset.
更多查看译文
关键词
Input validation vulnerabilities,XSS,SQLi,Minimal SSA,Features extraction,Machine learning
AI 理解论文
溯源树
样例
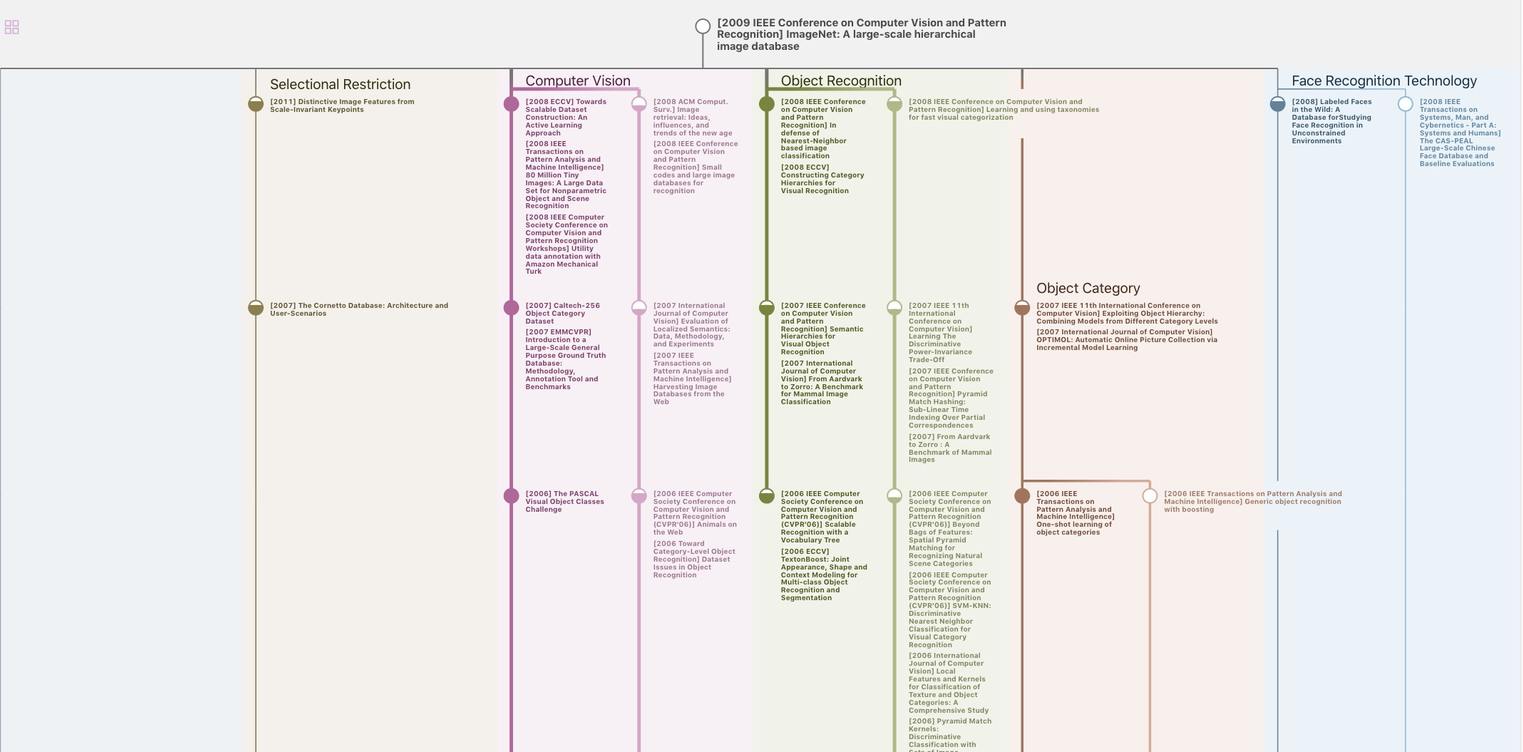
生成溯源树,研究论文发展脉络
Chat Paper
正在生成论文摘要