Image-text matching using multi-subspace joint representation
Multimedia Systems(2023)
Abstract
Joint representation learning has been an attractive way to solve image-text retrieval problem due to its efficiency on both time and storage. On the one hand, the most classical methods model the joint semantic subspace with respect to only semantic relationship at the level of holistic images and sentences, which fails to explore the fine-grained semantic relationship. On the other hand, visual-linguistic pretrain-finetune scheme has achieved an impressive performance in many downstream image-text tasks, but more storage cost and computation burden still notably limit their use in some real applications which have strict requirement for low storage cost or rapid response to image acquired in real-time, such as retrieving textual description of some just taken photos on a storage-limited mobile platform. To mitigate the problem above, we proposed a lightweight cross-modal retrieval for learning the joint representation. Instead of only modeling whole joint semantic space, the proposed model captures semantic relationship in multiple subspaces. Specially, we treat the retrieval problem as not only a ranking process but also a decision process, and propose an entropy-based constraint to preserve as much hierarchy-aware information as possible across various semantic subspaces. Experiments show that the proposed method can achieve competitive performance when compared with the state-of-the-art joint representation learning methods on two publicly available datasets.
MoreTranslated text
Key words
Joint representation,Image-text retrieval,Multi-subspace learning,Cross-modal matching
AI Read Science
Must-Reading Tree
Example
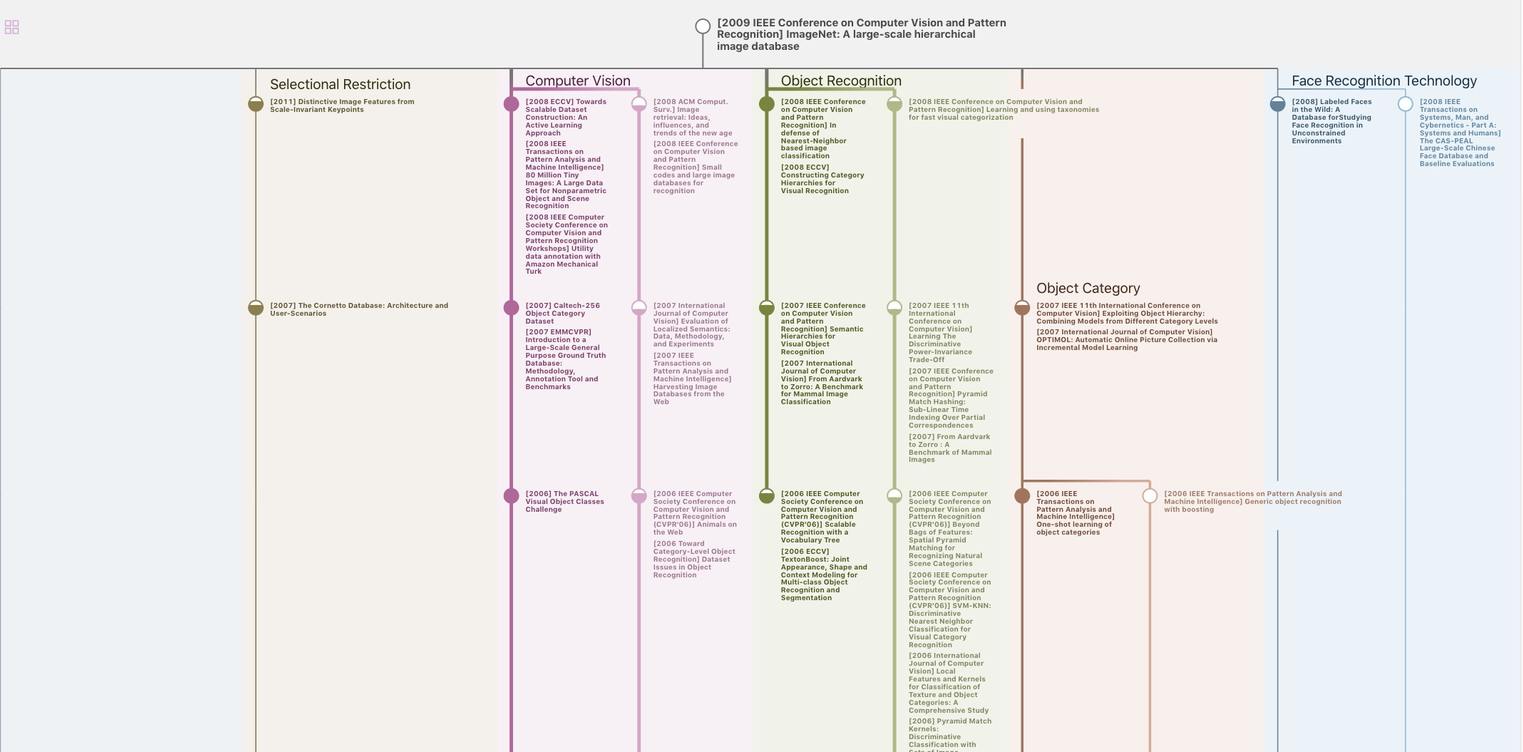
Generate MRT to find the research sequence of this paper
Chat Paper
Summary is being generated by the instructions you defined