Self-repairing infrared electronic nose based on ensemble learning and PCA fault diagnosis
Infrared Physics & Technology(2022)
Abstract
This paper reports an infrared electronic nose based on ensemble learning and principal component analysis (PCA) fault diagnosis, which addresses the self-detection and self-repair of the electronic nose for natural gas monitoring. We construct the detection array of the electronic nose with five non-dispersive infrared gas sensors. Ten (C53) multi-layer perceptual neural networks (MLPNN) are built by exploiting the cross-sensitivity mechanism of sensors, and each MLPNN makes predictions based on data from three infrared sensors. Moreover, the PCA fault diagnosis algorithm is used to identify sensor faults in real-time and select qualified MLPNNs for joint decision-making. The experimental results show that the proposed scheme exhibits high precision and reliability compared with the related methods. When there are two faulty sensors, the root mean squared errors of the electronic nose for methane, ethane, and propane are still within 3%, 2%, and 2%, respectively. In addition, an application case in a natural gas field validates the proposed method. This study presents a paradigm for building a self-repairing electronic nose based on cross-sensitivity. Not only natural gas but also other gases can be monitored by constructing high-reliability infrared electronic noses using similar schemes.
MoreTranslated text
Key words
Infrared sensor,Self-repairing,Ensemble learning,Fault identification,Natural gas
AI Read Science
Must-Reading Tree
Example
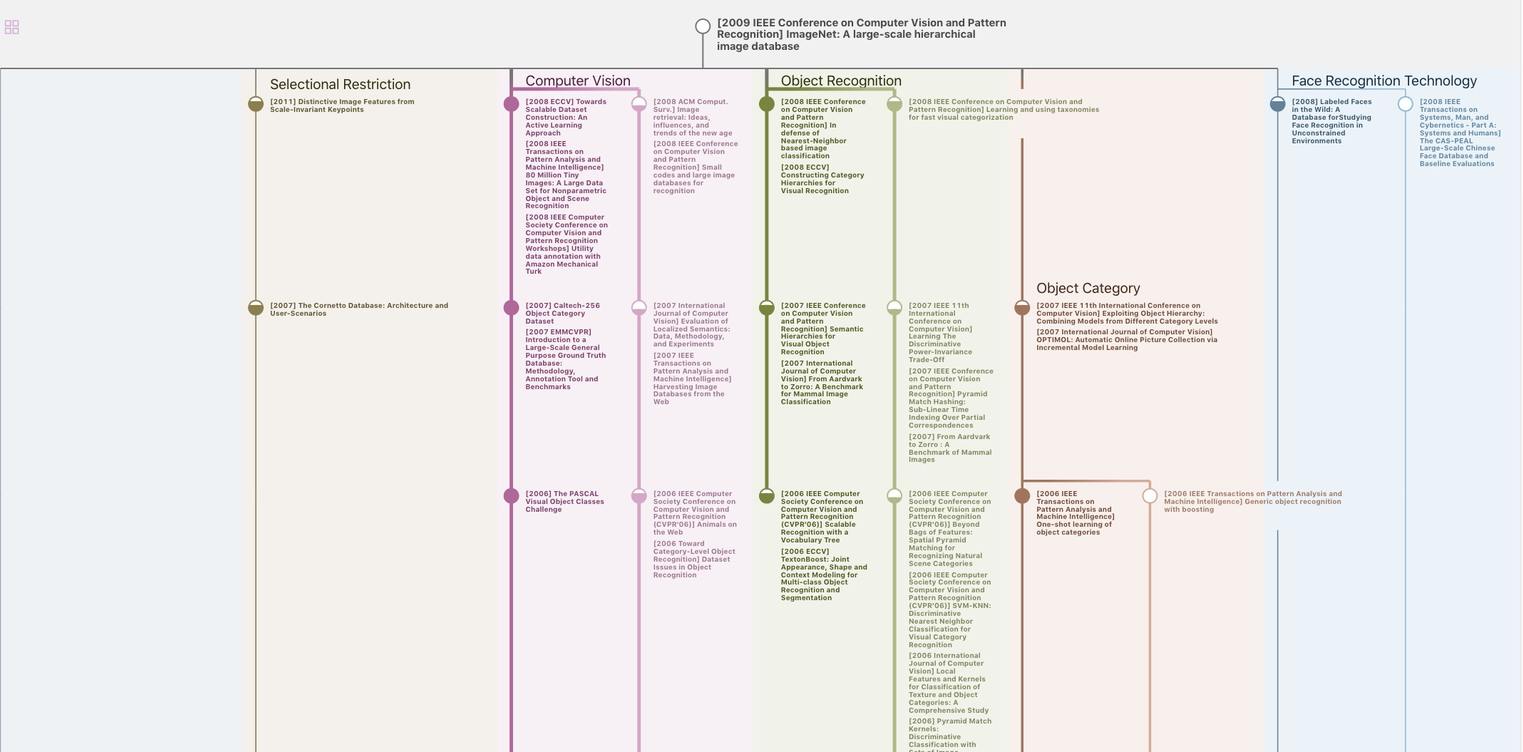
Generate MRT to find the research sequence of this paper
Chat Paper
Summary is being generated by the instructions you defined