Performance-driven closed-loop model learning with Bayesian optimization for the Car-in-the-Loop test bench control with unknown input
IFAC-PapersOnLine(2022)
摘要
A major control task of a full vehicle test bench is the tracking of the wheel hub speed with disturbance and unknown inputs such as the vehicle drive torque. For this control task, Internal Model Control (IMC) and Linear-Quadratic-Gaussian controller (LQG) are investigated on the quarter-vehicle prototype of a full vehicle test bench concept called Car-in-the-Loop (CiL). In a classic model-based control approach, the identification of the model and the optimization of the controller are carried out separately. The effort for system identification is huge and the sequential approach might result in a suboptimal closed-loop performance. With little prior knowledge of the complex system, we can use Bayesian optimization (BO) to learn a model that explicitly optimizes the control performance directly under closed-loop conditions. Experimental results on the CiL prototype show that similar or better control performance can be achieved with the performance-driven approach for both IMC and LQG.
更多查看译文
关键词
Bayesian optimization,closed-loop model learning,model-based control,IMC,LQG,Car-in-the-Loop
AI 理解论文
溯源树
样例
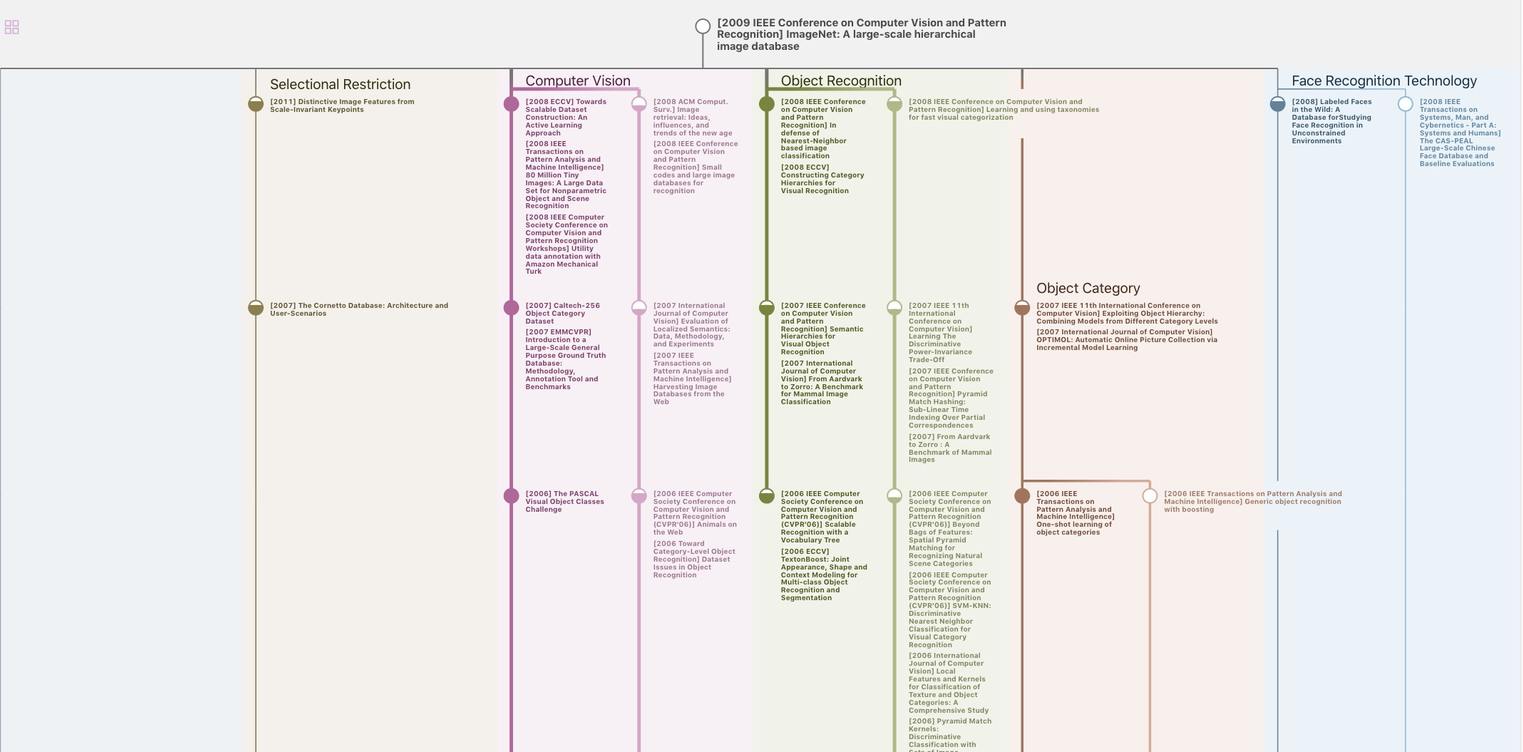
生成溯源树,研究论文发展脉络
Chat Paper
正在生成论文摘要