A novel classification approach based on context connotative network (CCNet): A case of construction site accidents
Expert Systems with Applications(2022)
摘要
Construction sites are the most hazardous workplace in the world. Occupational safety is one of the high-priority safety concerns across the globe. Among all the sectors, the construction sector contributes towards more hazardous fatalities. Along with a huge loss in manpower, it also causes drastic financial loss. In this study, text mining techniques along with deep neural networks are used for text classification. In detail, GloVe word embeddings with 300 dimensions are employed. We propose the context connotative network (CCNet) for accident causes classification. The dataset taken into account in this research work is referred from the Occupational Safety and Health Administration (OSHA) website. Besides, 5 baseline models namely Logistic Regression (LR), K-Nearest Neighbors (KNN), Support Vector Machines (SVM), Naive Bayes (NB), and Decision Tree (DT) are trained for comparison with the CCNet model. The results of this research work show that the proposed CCNet model outperforms all the other standard models employed, in respect of the average weighted F1 score metric. Experiment results prove that using GloVe embeddings helped to capture semantic relationships between words and extracting noun and verb phrases helped in boosting the performance of the proposed model.
更多查看译文
关键词
Construction accident reports classification,Deep learning,GloVe,Attention,Noun and verb phrases
AI 理解论文
溯源树
样例
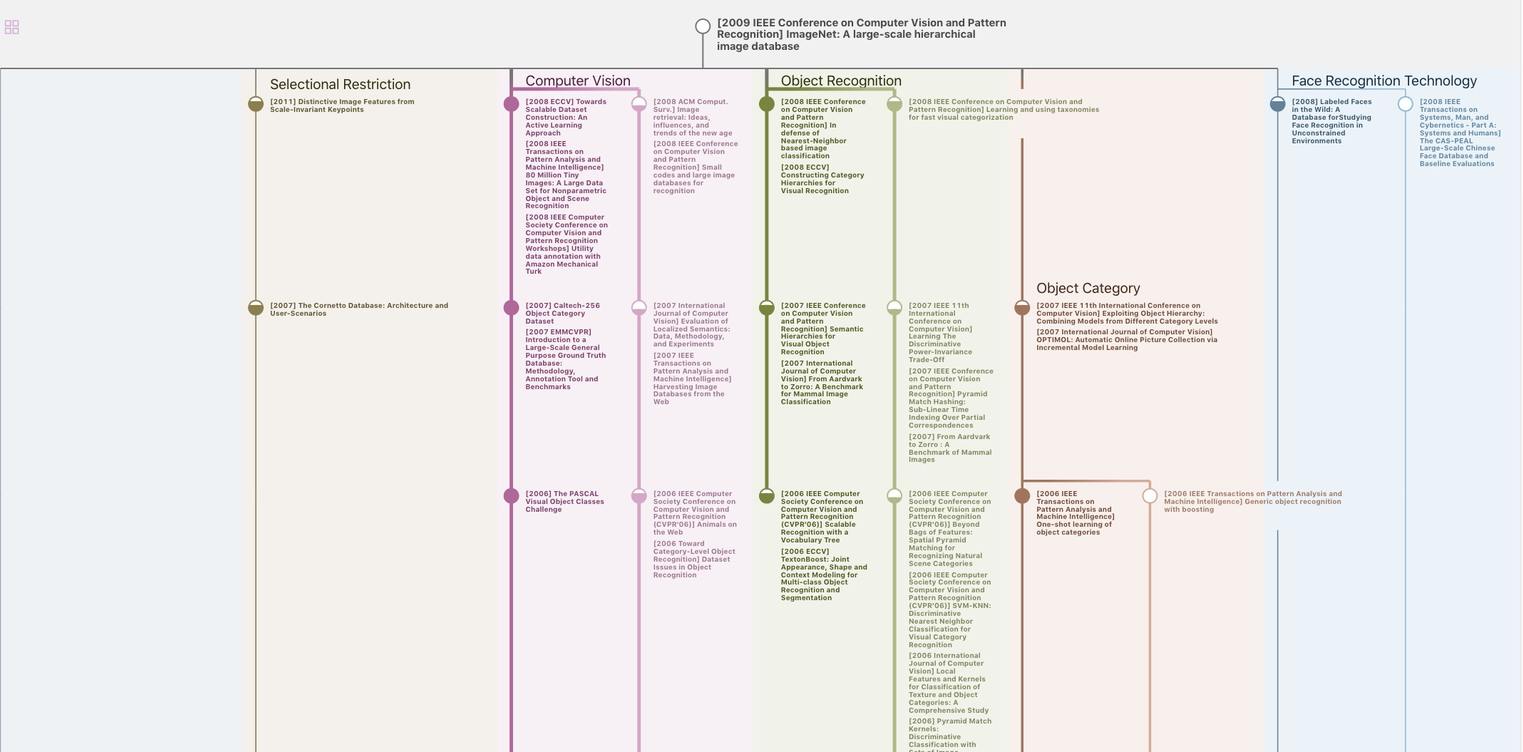
生成溯源树,研究论文发展脉络
Chat Paper
正在生成论文摘要