Review of Well-to-Wheel lifecycle emissions of liquefied natural gas heavy goods vehicles
Applied Energy(2023)
摘要
It has been suggested that using liquefied natural gas as a fuel source for heavy goods vehicles could provide a reduction in greenhouse gas emissions. Various studies have investigated different aspects of the lifecycle emissions of natural gas heavy goods vehicles throughout the past decade, however, there has been little comparative analysis across these studies. This review provides a comprehensive examination of the well-to-wheel lifecycle emissions of liquefied natural gas for heavy goods vehicles in comparison to diesel, the current standard. A systematic selection criteria based on relevance to the defined well-to-wheel system boundary of liquefied natural gas as a fuel source for heavy goods vehicles, including greenhouse gas emissions, were augmented by the authors knowledge of the field. The various data are categorised by engine technology and model year (pre- and post-2015), average speed of the duty cycle, and then statistically analysed to identify clear trends and correlations in the emissions produced. The two primary factors affecting the well-to-wheel greenhouse gas emissions of natural gas heavy hoods vehicles are: (i) natural gas engine fuel efficiency relative to diesel, and (ii) methane leakage across the supply chain. Methane leakage rates are a significant uncertainty and range from 0.3 to 20 % of throughput. With long-term perspective of efficiency penalty (10 %) in natural gas engines, the well-to-wheel greenhouse gas emissions reduction of natural gas fuelled trucks against diesel is up to 10 %, which appears insufficient toward net zero emissions by 2050. The use of biomethane further reduces the greenhouse gas emissions by 34–66 % depending on the engine technology. Controlling fugitive methane emissions in the fuel production and supply chain remains critical.
更多查看译文
关键词
Liquefied natural gas,Heavy goods vehicles,Methane slip,Well-to-wheel,Greenhouse gas emissions
AI 理解论文
溯源树
样例
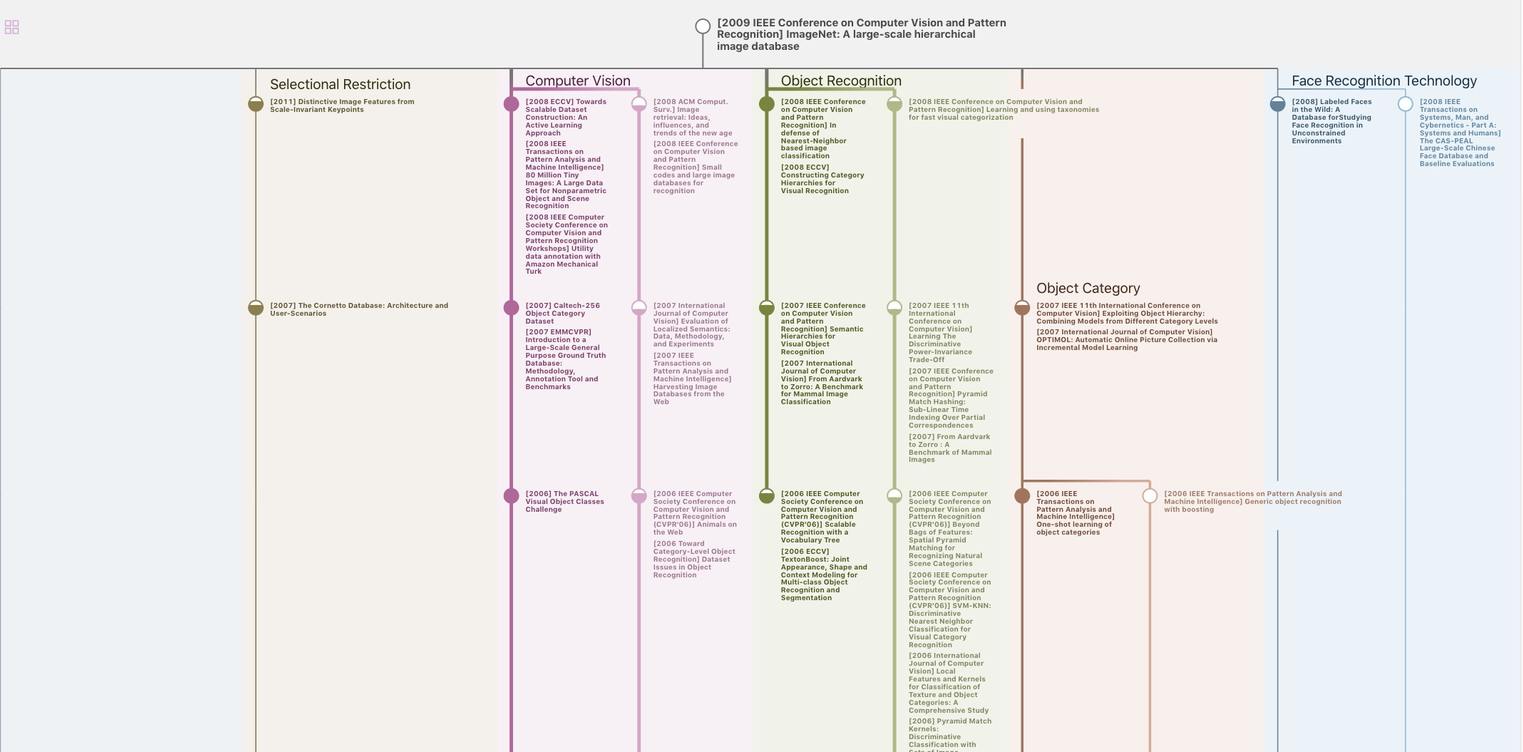
生成溯源树,研究论文发展脉络
Chat Paper
正在生成论文摘要