Traditional machine learning algorithms for breast cancer image classification with optimized deep features
Biomedical Signal Processing and Control(2023)
摘要
For breast cancer diagnosis, computer-aided classification of histopathological images is of critical importance for correct and early diagnosis. Transfer learning approaches for feature extraction have made significant progress in recent years and are now widely used. To select the best representative features to classify breast cancer pathological images and avoid the curse of dimensionality, this work uses optimized deep features. The proposed approach firstly employs ResNet18 architecture for feature extraction to achieve deep features. Then, meta-heuristic algorithms namely Particle Swarm Optimization (PSO), Atom Search Optimization (ASO) and Equilibrium Optimizer (EO) algorithms, are employed to provide more representative features of breast cancer pathological images. To understand the effect of optimized deep features on classification, traditional machine learning (ML) algorithms are used. The experimental analysis of the proposed approach has been done on the public benchmark dataset BreakHis. Experimental results illustrate that, for features obtained from ResNet18-EO, the proposed approach achieves a 97.75% F-score by using the Support Vector Machine (SVM) with gaussian and radial-based functions (RBF).
更多查看译文
关键词
Histopathological image classification,Transfer learning,Meta-heuristic algorithms,Machine learning
AI 理解论文
溯源树
样例
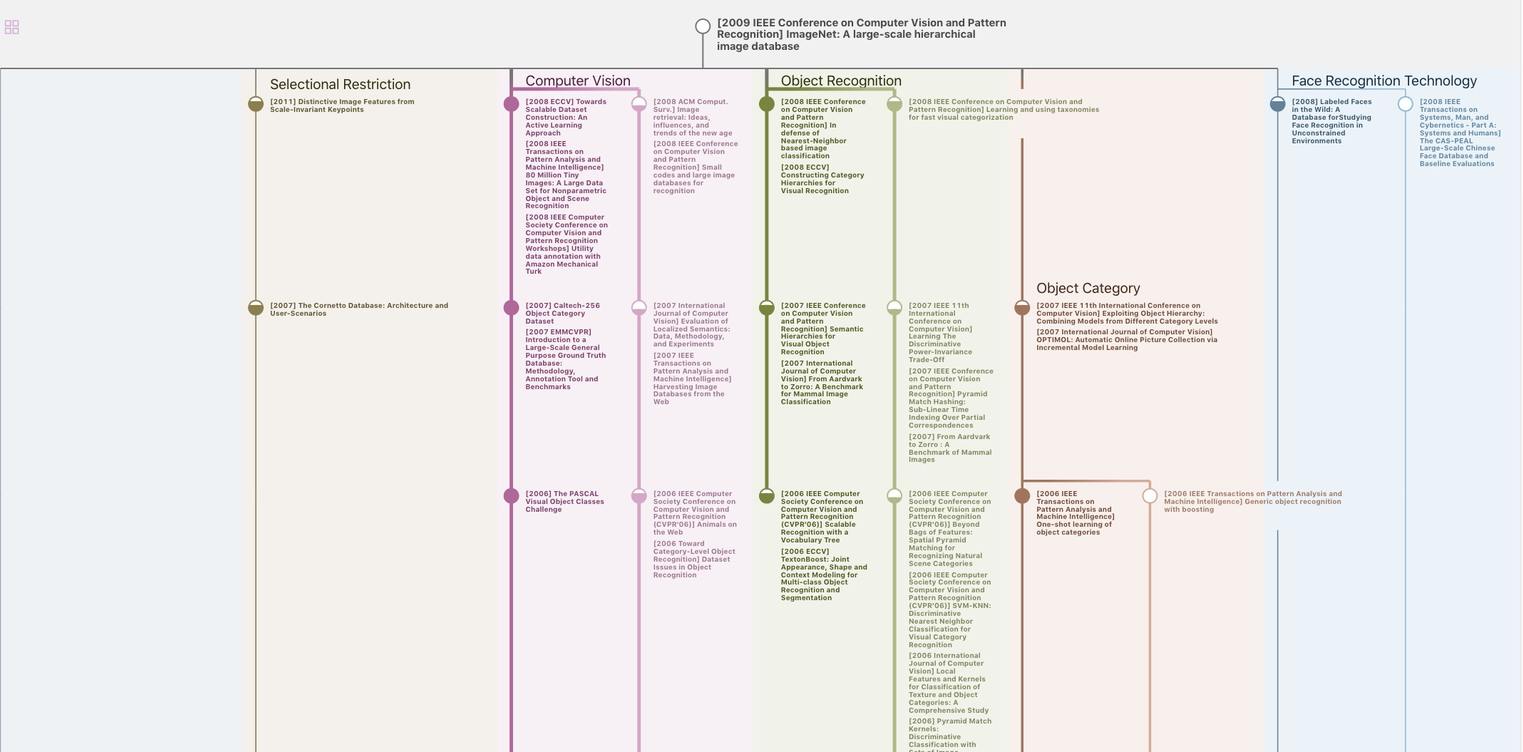
生成溯源树,研究论文发展脉络
Chat Paper
正在生成论文摘要