SAR-CGAN: Improved generative adversarial network for EIT reconstruction of lung diseases
Biomedical Signal Processing and Control(2023)
摘要
The image reconstruction of electrical impedance tomography (EIT) is a nonlinear ill-posed inverse problem, and the reconstructed images tend to have artifacts due to noise in the measurement system. The shallow neural networks are difficult to fully express the nonlinear relationship of the EIT imaging problems. Deep neural networks have demonstrated great potential to remove artifacts from initial conductivity images caused by system noise. In the paper, a novel deep learning method, namely, conditional generative adversarial network with soft-attention gates (AGs) and residual learning (SAR-CGAN), is proposed to solve the above problems of EIT imaging. SAR-CGAN consists of generator and discriminator. Specifically, the AGs are employed by the generator to improve sensitivity and accuracy for lung EIT imaging by suppressing feature activation in irrelevant EIT region. And residual learning framework is introduced in the discriminator to address the problem of model degradation and easily enjoy accuracy gains from greatly increased depth. Extensive confirmatory and comparative experiments with three kinds of lung disease are conducted and compared with CNN, DnCNN, Attention U-Net and CGAN methods. The imaging results indicate that SAR-CGAN method can accurately recover the position and boundaries of lesions in lung images and effective reconstruction can be accomplished for the new conductivity distribution and noisy samples.
更多查看译文
关键词
Electrical impedance tomography,Image reconstruction,Generative adversarial network,Lung disease
AI 理解论文
溯源树
样例
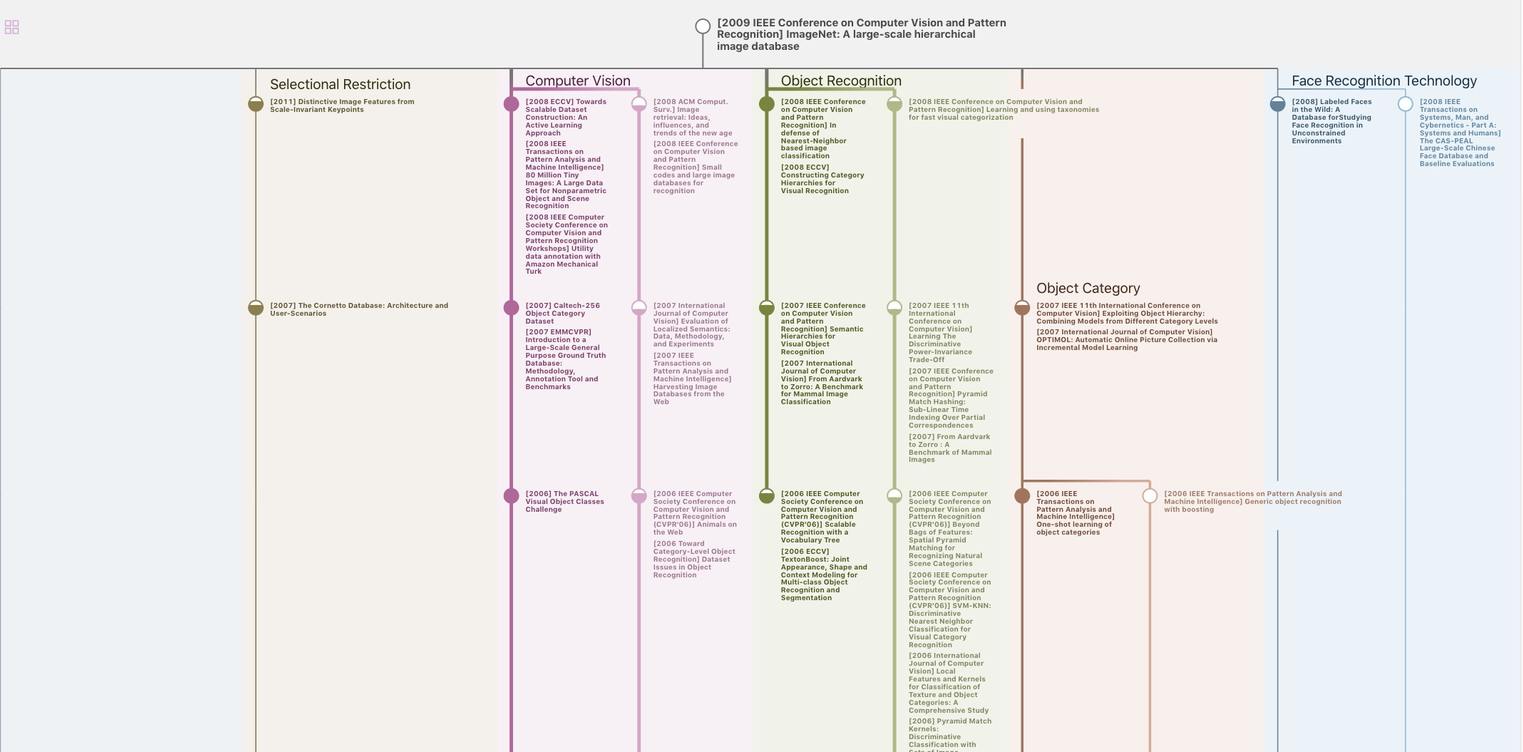
生成溯源树,研究论文发展脉络
Chat Paper
正在生成论文摘要