An explainable attention-based TCN heartbeats classification model for arrhythmia detection
Biomedical Signal Processing and Control(2023)
摘要
Background and Objective:
Electrocardiogram (ECG) is a non-invasive tool to measure the heart’s electrical activity. ECG signal based automatic heartbeat classification is a critical task for arrhythmia detection and continues to be challenging. While diverse automated classification methods have been developed, they still cannot provide acceptable performance in classifying different heartbeats because of their poor ability to extract abstract patterns comprehensively. Besides, the performance of previous work drops sharply when dealing with imbalanced datasets and lacks interpretability.
Methods:
This paper proposes a novel, explainable attention-based temporal convolutional network(TCN) heartbeat classification method. The first contribution of our approach is that we fuse the TCN architecture and self-attention mechanism to encode the ECG heartbeat sequences. Specifically, TCN and the self-attention block are designed to capture global variation tends and local features, respectively, to best serve the classification. Meanwhile, multi-class focal loss helps model training overcome the class imbalance problem. In the end, the dynamic perturbation based high-fidelity explanation module was introduced to understand the AI-based model and enhance the model’s transparency to clinicians.
Conclusions:
Experiments on the MIT-BIH-AD dataset demonstrate that our model with a simpler architecture can achieve 99.84% accuracy, 99.90% specificity and 99.60% precision for the intra-patient scheme and 87.81% accuracy, 91.85% sensitivity and 89.81% precision for the inter-patient scheme, which outperforms most of the state-of-the-art(SOTA) works, especially for minority classes.
更多查看译文
关键词
Heartbeat classification,Arrhythmia,Deep learning,Imbalanced dataset classification,Explainable artificial intelligence (XAI)
AI 理解论文
溯源树
样例
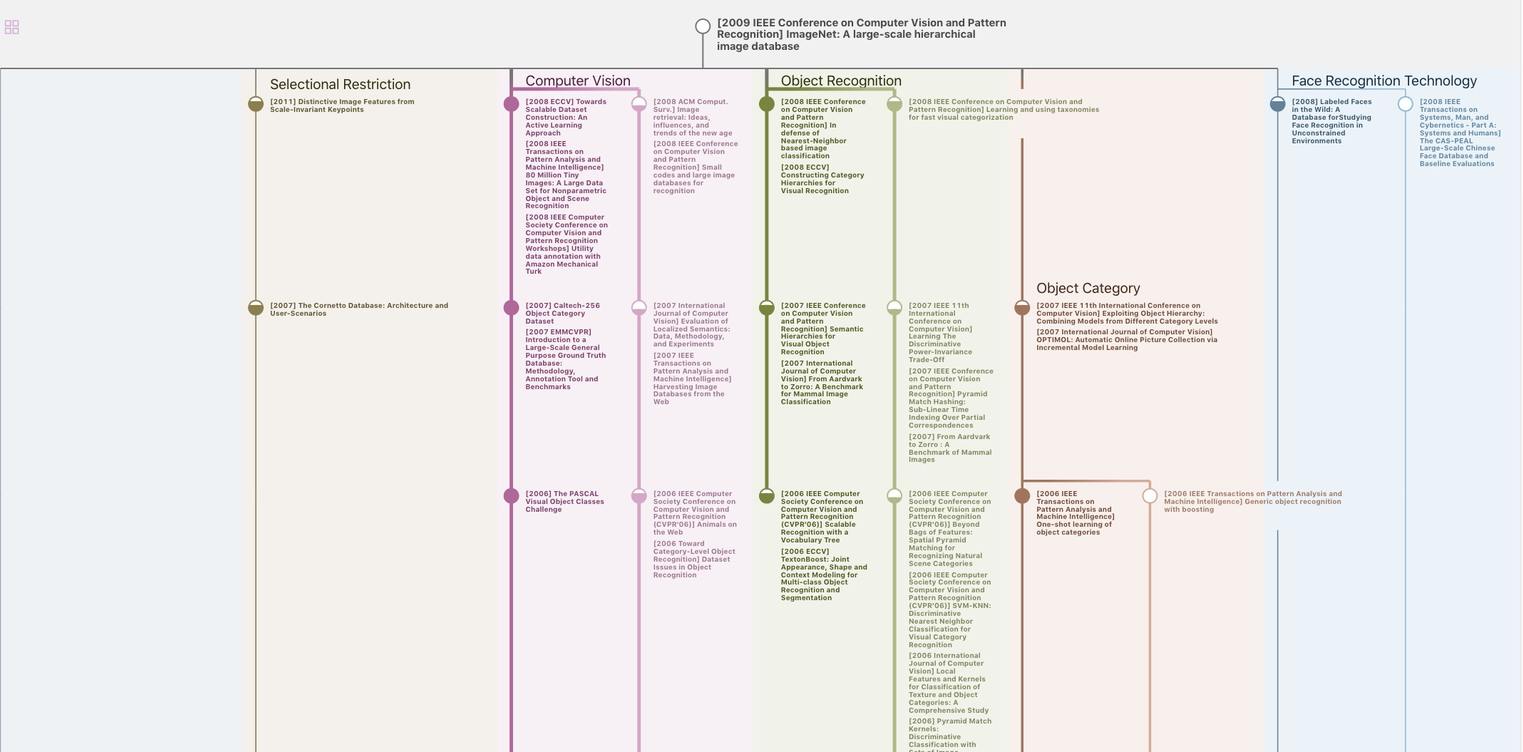
生成溯源树,研究论文发展脉络
Chat Paper
正在生成论文摘要