Task-Effective Compression of Observations for the Centralized Control of a Multi-agent System Over Bit-Budgeted Channels
IEEE Internet Things J(2023)
Abstract
We consider a task-effective quantization problem that arises when multiple agents are controlled via a centralized controller (CC). While agents have to communicate their observations to the CC for decision-making, the bit-budgeted communications of agent-CC links may limit the task-effectiveness of the system which is measured by the system's average sum of stage costs/rewards. As a result, each agent should compress/quantize its observation such that the average sum of stage costs/rewards of the control task is minimally impacted. We address the problem of maximizing the average sum of stage rewards by proposing two different Action-Based State Aggregation (ABSA) algorithms that carry out the indirect and joint design of control and communication policies in the multi-agent system. While the applicability of ABSA-1 is limited to single-agent systems, it provides an analytical framework that acts as a stepping stone to the design of ABSA-2. ABSA-2 carries out the joint design of control and communication for a multi-agent system. We evaluate the algorithms - with average return as the performance metric - using numerical experiments performed to solve a multi-agent geometric consensus problem. The numerical results are concluded by introducing a new metric that measures the effectiveness of communications in a multi-agent system.
MoreTranslated text
Key words
Semantic communications,task-effective data compression,goal-oriented communications,communications for machine learning,multi-agent systems,reinforcement learning
AI Read Science
Must-Reading Tree
Example
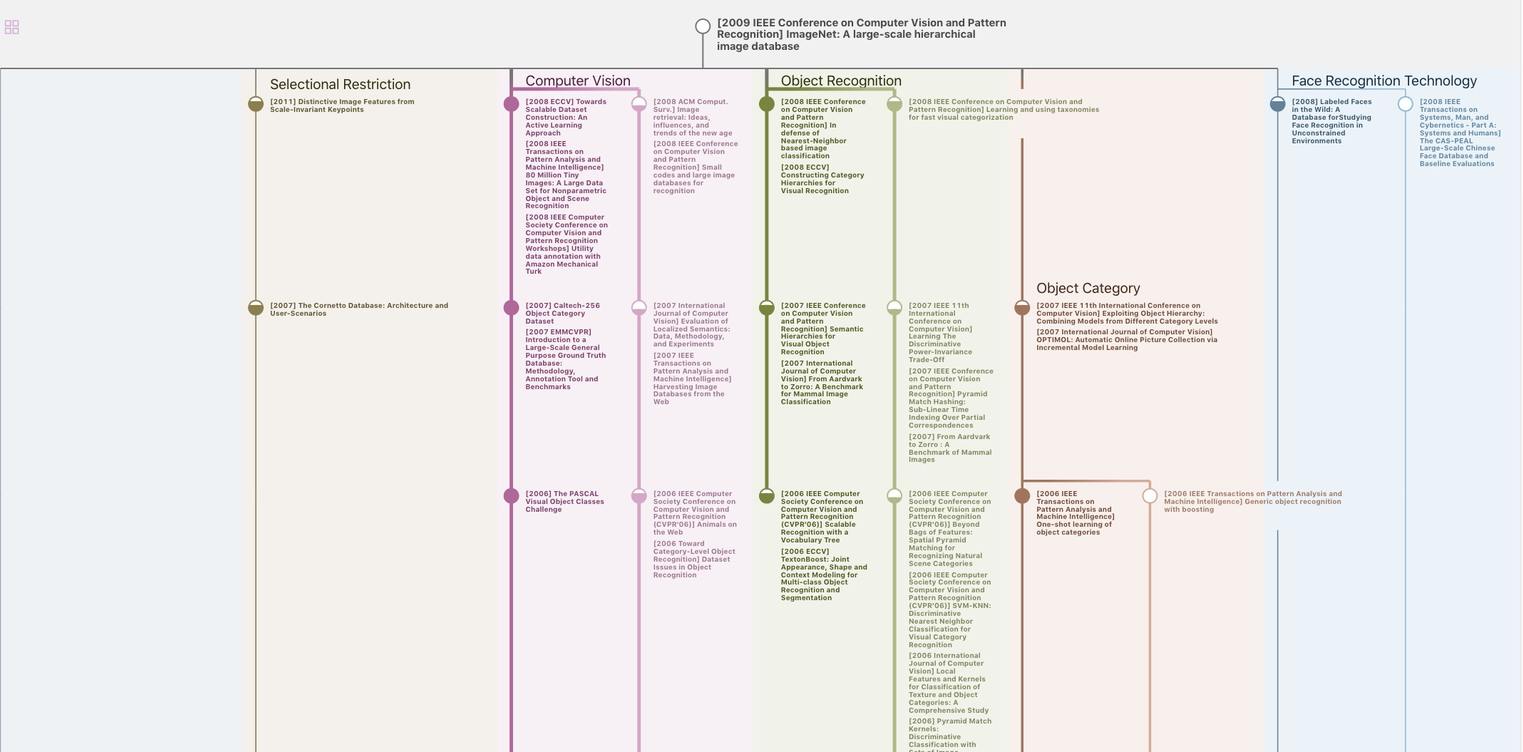
Generate MRT to find the research sequence of this paper
Chat Paper
Summary is being generated by the instructions you defined