Strong correlation effects observed by an ANN-MFT encoder trained on $\alpha$-RuCl$_3$ high magnetic field data
arXiv (Cornell University)(2023)
摘要
$\alpha$-RuCl3 is a magnetic insulator exhibiting quantum spin liquid phases possibly found in the Kitaev honeycomb model. Much of the effort towards determining Hamiltonian parameters has focused on low magnetic field ordered phases. We study this problem in the high magnetic field limit where mean-field theory is better justified. We do so by machine-learning model parameters from over 200,000 low dimensional data points by combining available magnetization, torque, and torsion data sets. Our machine, an artificial neural network-mean-field theory (ANN-MFT) encoder, maps thermodynamic conditions (temperature and field vector) to model parameters via a fully connected time-reversal covariant (equivariant) neural network and then predicts observable values using mean-field theory. To train the machine, we use PyTorch to enable backpropagation through mean-field theory with a pure PyTorch implementation of the Newton-Raphson method. The results at $20$ K and $34.5$ T are consistent with other parameter inference studies in the literature at low magnetic field but strikingly have magnitudes that scale with temperature from 1.3 K up to 80 K in the $34.5-60$ T range. We conclude that the data presents physics beyond the scope of the mean-field theory and that strong interactions dominate the physics of $\alpha$-RuCl$_3$ up to field strengths of at least 60 Tesla.
更多查看译文
关键词
strong correlation effects,correlation effects,ann-mft
AI 理解论文
溯源树
样例
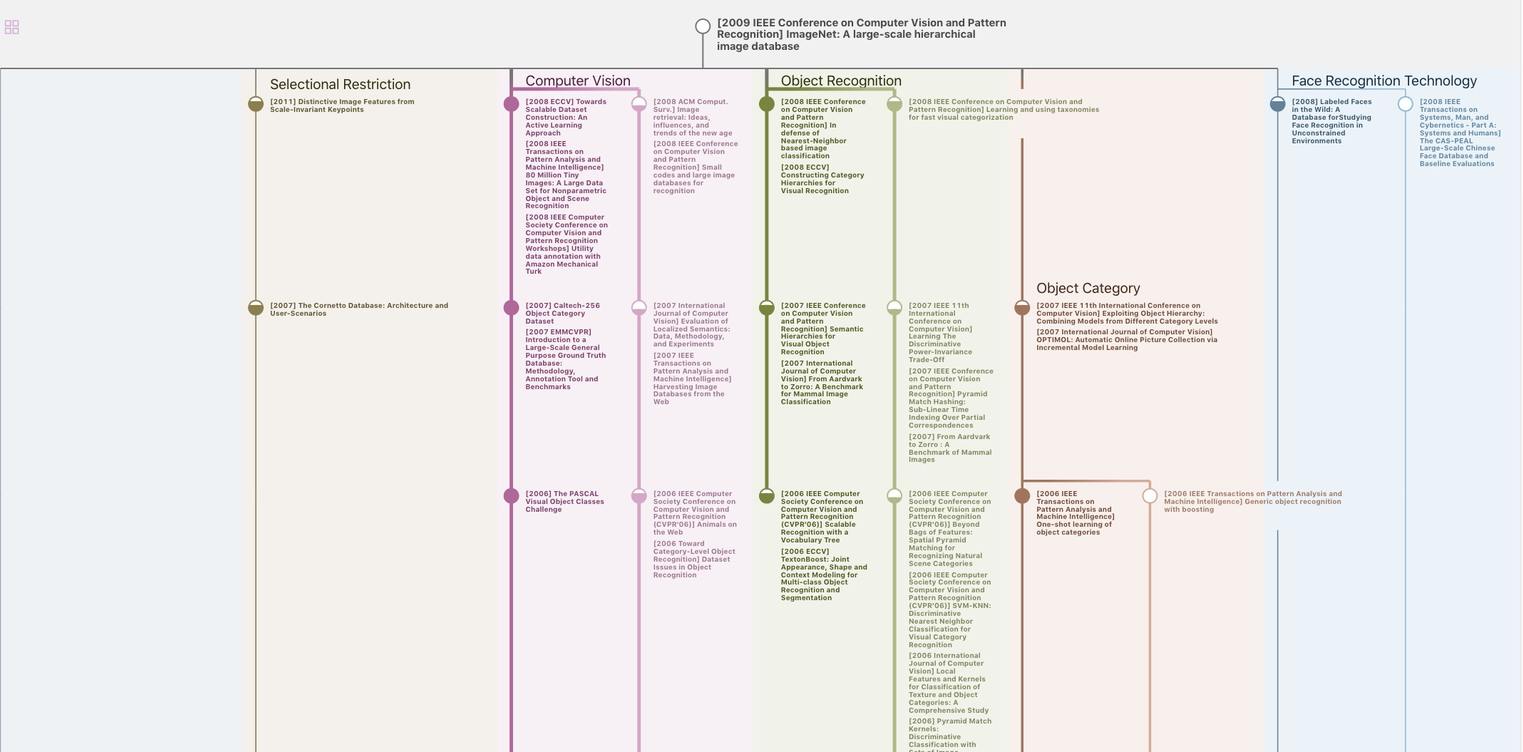
生成溯源树,研究论文发展脉络
Chat Paper
正在生成论文摘要