Classification of BATSE, Swift, and Fermi Gamma-Ray Bursts from Prompt Emission Alone
arxiv(2023)
摘要
Although it is generally assumed that there are two dominant classes of gamma-ray bursts (GRB) with different typical durations, it has been difficult to unambiguously classify GRBs as short or long from summary properties such as duration, spectral hardness, and spectral lag. Recent work used t-distributed stochastic neighborhood embedding (t-SNE), a machine learning algorithm for dimensionality reduction, to classify all Swift gamma-ray bursts as short or long. Here, the method is expanded, using two algorithms, t-SNE and UMAP, to produce embeddings that are used to provide a classification for the 1911 BATSE bursts, 1321 Swift bursts, and 2294 Fermi bursts for which both spectra and metadata are available. Although the embeddings appear to produce a clear separation of each catalog into short and long bursts, a resampling-based approach is used to show that a small fraction of bursts cannot be robustly classified. Further, 3 of the 304 bursts observed by both Swift and Fermi have robust but conflicting classifications. A likely interpretation is that in addition to the two predominant classes of GRBs, there are additional, uncommon types of bursts which may require multi-wavelength observations in order to separate from more typical short and long GRBs.
更多查看译文
关键词
Gamma-ray bursters,Gamma-ray bursts,Dimensionality reduction,Classification systems
AI 理解论文
溯源树
样例
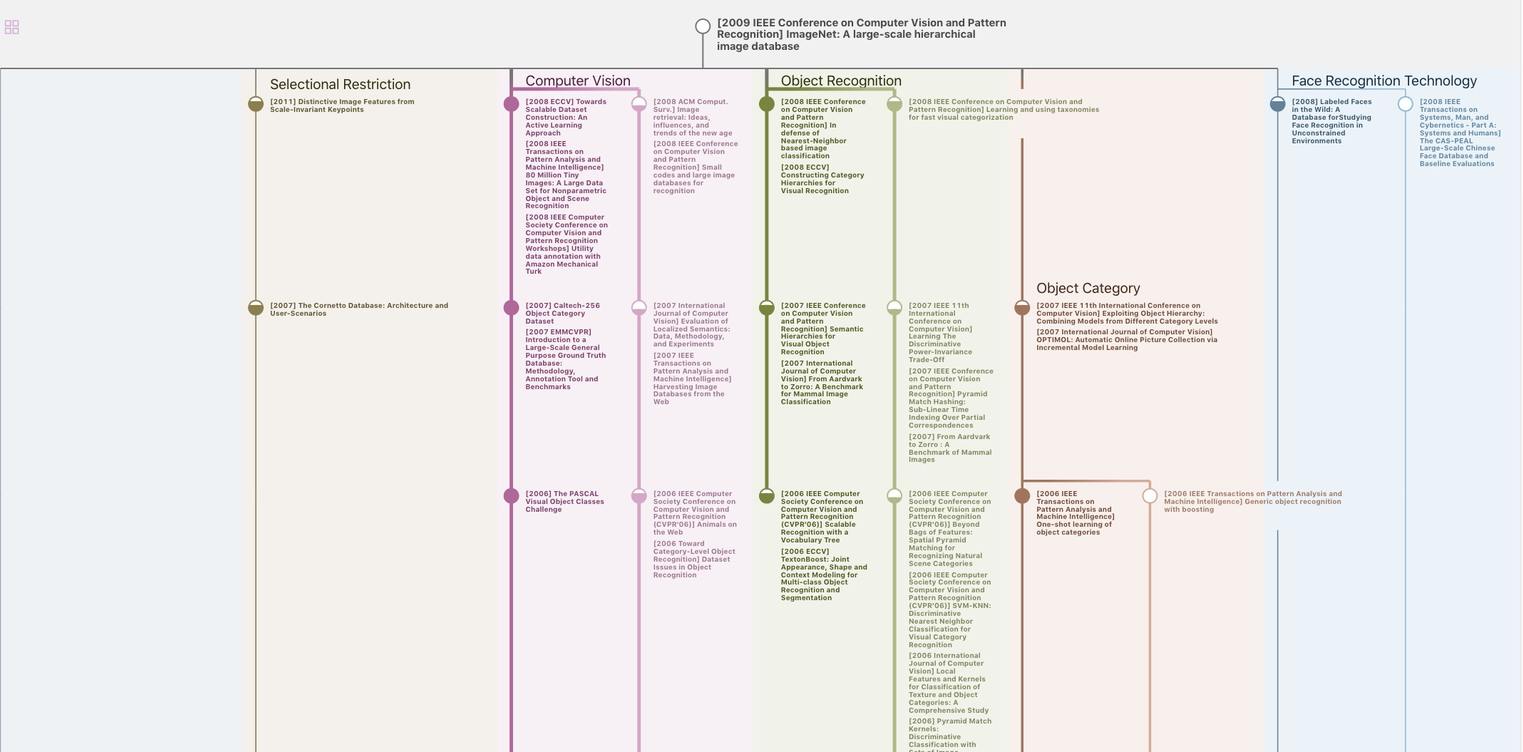
生成溯源树,研究论文发展脉络
Chat Paper
正在生成论文摘要