PET Normalizations to Improve Deep Learning Auto-Segmentation of Head and Neck Tumors in 3D PET/CT.
International Conference on Medical Image Computing and Computer-Assisted Intervention (MICCAI)(2021)
摘要
Auto-segmentation of head and neck cancer (HNC) primary gross tumor volume (GTVt) is a necessary but challenging process for radiotherapy treatment planning and radiomics studies. The HEad and neCK TumOR Segmentation Challenge (HECKTOR) 2021 comprises two major tasks: auto-segmentation of GTVt in FDG-PET/CT images and the prediction of patient outcomes. In this paper, we focus on the segmentation part by proposing two PET normalization methods to mitigate impacts from intensity variances between PET scans for deep learning-based GTVt auto-segmentation. We also compared the performance of three popular hybrid loss functions. An ensemble of our proposed models achieved an average Dice Similarity Coefficient (DSC) of 0.779 and median 95% Hausdorff Distance (HD95) of 3.15 mm on the test set. Team: Aarhus_Oslo.
更多查看译文
关键词
3d pet,neck tumors,deep learning,auto-segmentation
AI 理解论文
溯源树
样例
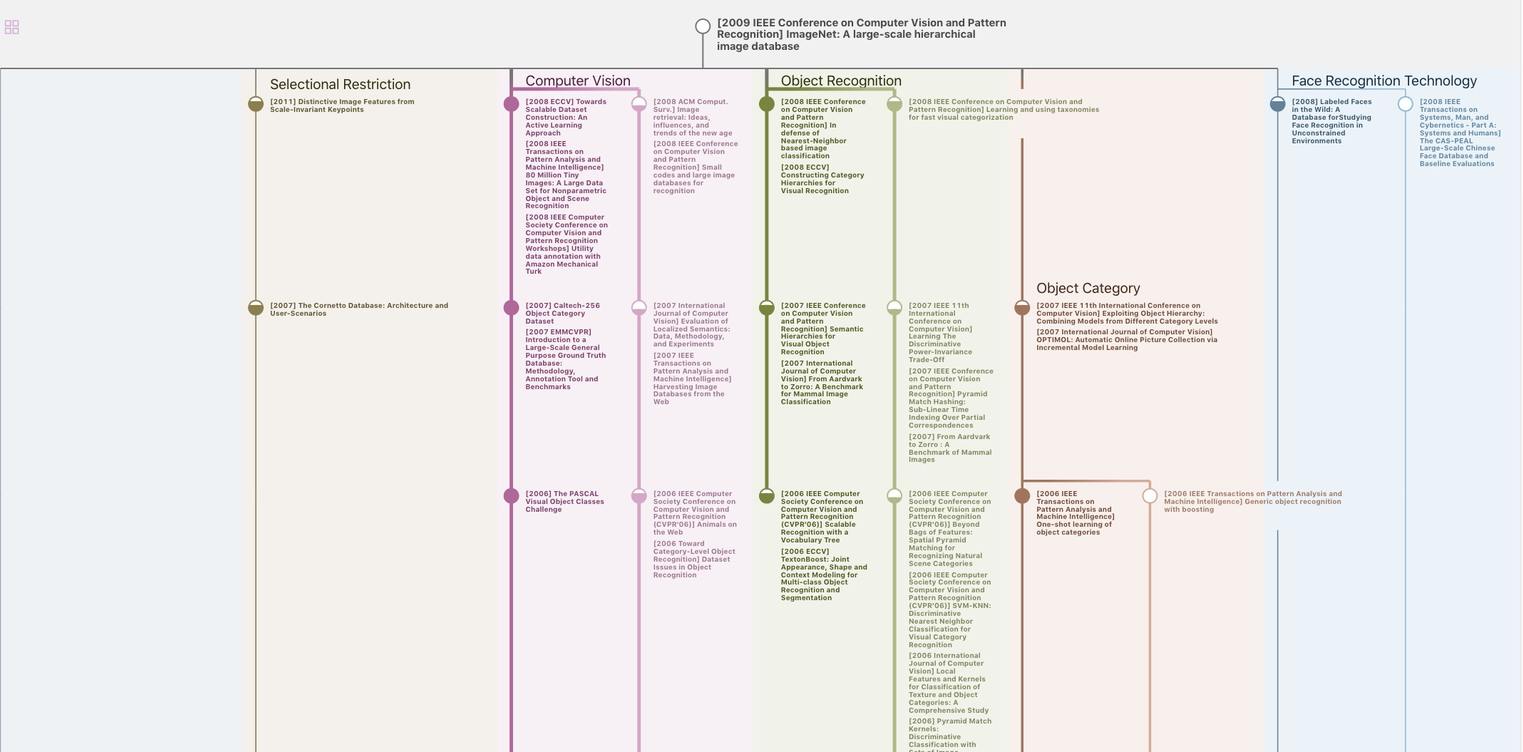
生成溯源树,研究论文发展脉络
Chat Paper
正在生成论文摘要