Multi-D3QN: A Multi-strategy Deep Reinforcement Learning for Service Composition in Cloud Manufacturing.
International Conference on Collaborative Computing (CollaborateCom)(2021)
摘要
Service composition is an indispensable technology in the cloud manufacturing process to ensure the smooth execution of tasks. To implement effective and accurate service composition strategies, many researchers choose to use Meta-heuristics algorithms with strong optimization capabilities. However, as users’ demand of personalized products increasing, dynamic service composition is essential. Meta-heuristics algorithms lack dynamic adaptability, so they are not suitable for solving complex and dynamic service composition problems. Deep Reinforcement Learning (DRL) algorithm is difficult to reach a stable state, when the hyper-parameters and rewards in the algorithm are not properly designed. To solve these problems, we propose a Multi-strategy Deep Reinforcement Learning (DRL) algorithm, named Multi-D3QN, which combines the basic DQN algorithm, the dueling architecture, the double estimator and the prioritized replay mechanism. Meanwhile, we add some strategies such as instant reward, the ɛ-greedy policy and a heuristic strategy to ensure better performance of the algorithm in dynamic environment. Experiments show that our proposed method not only adapt to the dynamic environment, but also obtain a better solution.
更多查看译文
关键词
Cloud manufacturing,Dynamic service composition,Quality of service,Deep reinforcement learning
AI 理解论文
溯源树
样例
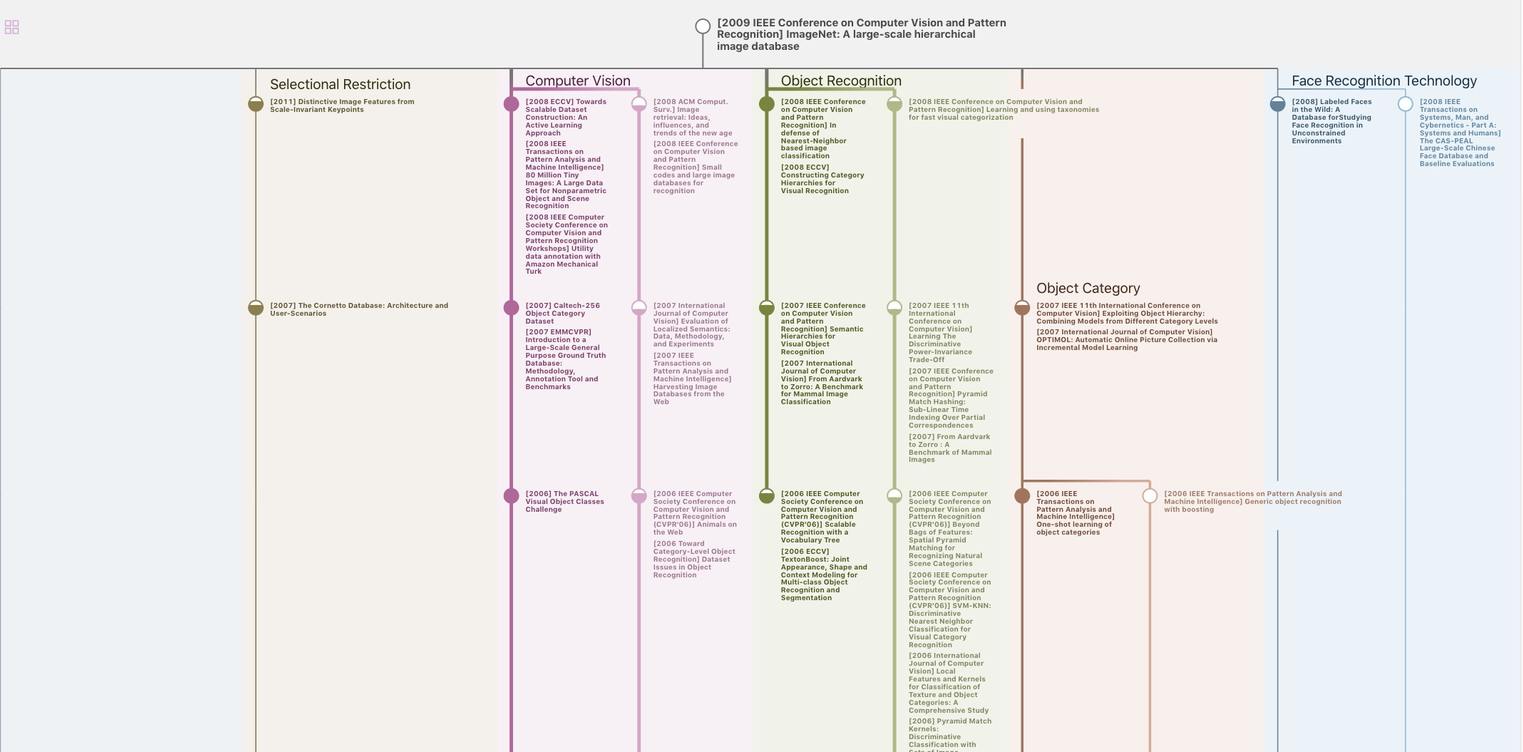
生成溯源树,研究论文发展脉络
Chat Paper
正在生成论文摘要