Combining Global Information with Topological Prior for Brain Tumor Segmentation.
International Workshop on Brainlesion: Glioma, Multiple Sclerosis, Stroke and Traumatic Brain Injuries (BrainLes)(2021)
Abstract
Gliomas are the most common and aggressive malignant primary brain tumors. Automatic brain tumor segmentation from multimodality magnetic resonance images using deep learning methods is critical for gliomas diagnosis. Deep learning segmentation architectures, especially based on fully convolutional neural network, have proved great performance on medical image segmentation. However, these approaches cannot explicitly model global information and overlook the topology structure of lesion regions, which leaves room for improvement. In this paper, we propose a convolution-and-transformer network (COTRNet) to explicitly capture global information and a topology aware loss to constrain the network to learn topological information. Moreover, we exploit transfer learning by using pretrained parameters on ImageNet and deep supervision by adding multi-level predictions to further improve the segmentation performance. COTRNet achieved dice scores of 78.08%, 76.18%, and 83.92% in the enhancing tumor, the tumor core, and the whole tumor segmentation on brain tumor segmentation challenge 2021. Experimental results demonstrated effectiveness of the proposed method.
MoreTranslated text
Key words
Brain tumor segmentation,Convolutional neural network,Transformer
AI Read Science
Must-Reading Tree
Example
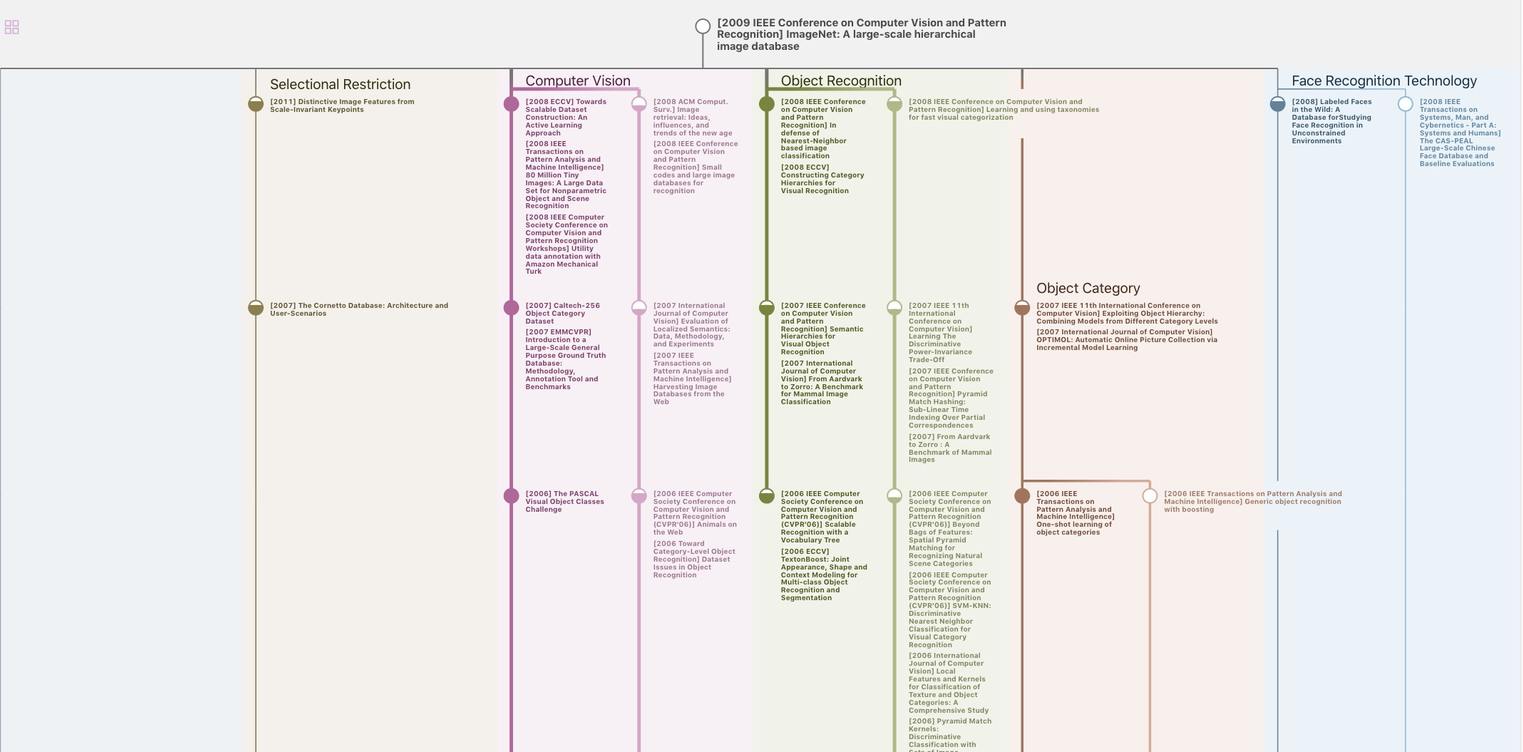
Generate MRT to find the research sequence of this paper
Chat Paper
Summary is being generated by the instructions you defined