Taking the Confusion Out of Multinomial Confusion Matrices and Imbalanced Classes.
Australasian Data Mining Conference (AusDM)(2021)
摘要
Classification is a fundamental task in machine learning, and the principled design and evaluation of classifiers is vital to create effective classification systems and to characterise their strengths and limitations in different contexts. Binary classifiers have a range of well-known measures to summarise performance, but characterising the performance of multinomial classifiers (systems that classify instances into one of many classes) is an open problem. While confusion matrices can summarise the empirical performance of multinomial classifiers, they are challenging to interpret at a glance-challenges compounded when classes are imbalanced. We present a way to decompose multinomial confusion matrices into components that represent the prior and posterior probabilities of correctly classifying each class, and the intrinsic ability of the classifier to discriminate each class: the Bayes factor or likelihood ratio of a positive (or negative) outcome. This approach uses the odds formulation of Bayes' rule and leads to compact, informative visualisations of confusion matrices, able to accommodate far more classes than existing methods. We call this method confusR and demonstrate its utility on 2-, 17-, and 379-class confusion matrices. We describe how confusR could be used in the formative assessment of classification systems, investigation of algorithmic fairness, and algorithmic auditing.
更多查看译文
关键词
Classification,Multiclass,Visualisation,Performance,Fairness,Auditing
AI 理解论文
溯源树
样例
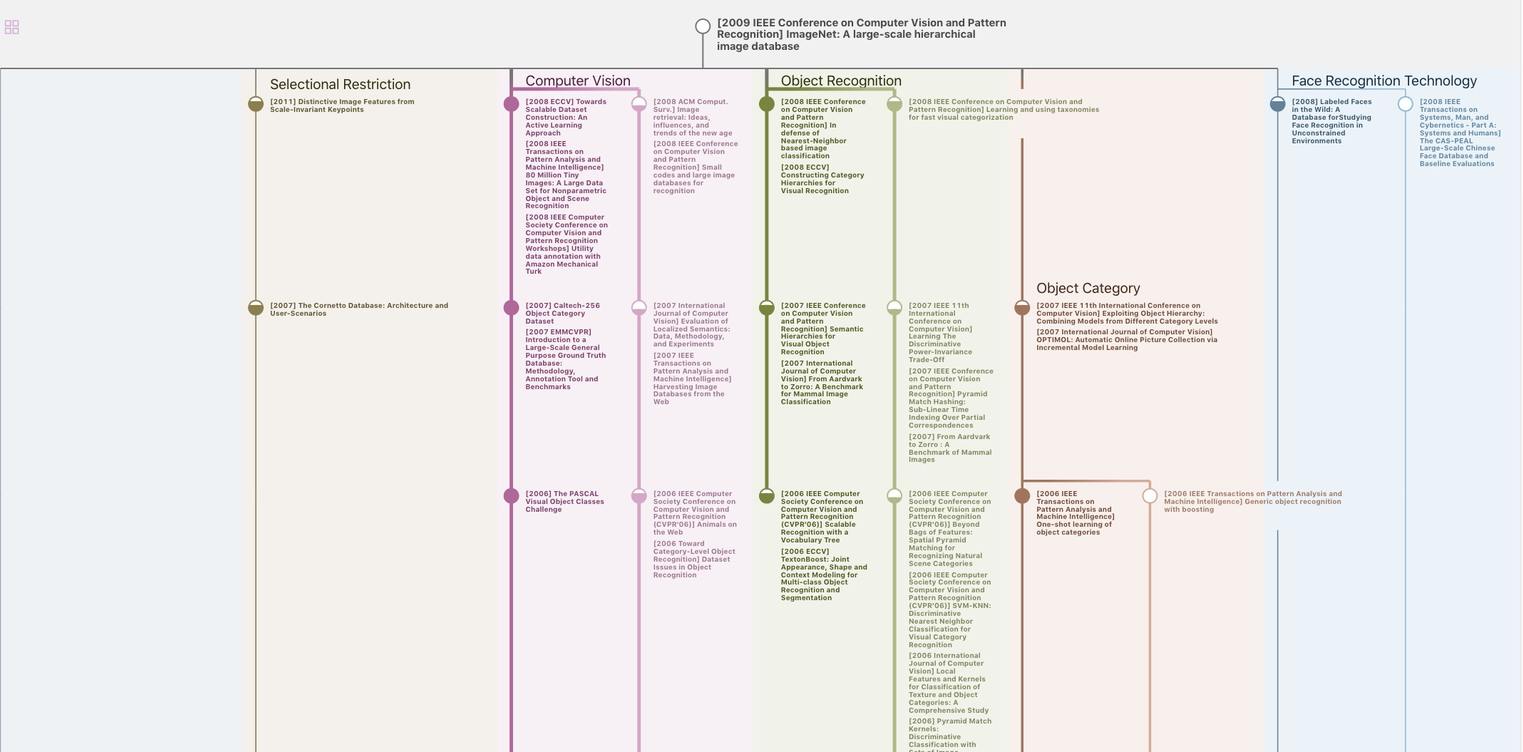
生成溯源树,研究论文发展脉络
Chat Paper
正在生成论文摘要