KIDNet: A Knowledge-Aware Neural Network Model for Academic Performance Prediction.
ACM International Conference on International Agent Technology (IAT)(2021)
Abstract
Academic performance prediction and analysis in educational data mining is meaningful for instructors to know the student's ongoing learning status, and also provide appropriate help to students as early as possible if academic difficulties appear. In this paper, we first collect the basic information of students and courses as features. Then, we propose a novel knowledge extraction framework to obtain course knowledge features to reinforce feature groups. The comparative analyses of the knowledge similarity and average grades of the courses in all terms demonstrate a strong correlation between them. Furthermore, we build the Knowledge Interaction Discovery Network (KIDNet) model, based on factorization machine (FM) and deep neural network (DNN) algorithms. This model uses FM to model lower-order interactions of sparse features and employs DNN to model higher-order interactions of both dense and sparse features. The effectiveness of KIDNet has been validated by conducting experiments based on a real-world dataset.
MoreTranslated text
Key words
Educational Data, Academic Prediction, Knowledge Graph Embedding, Knowledge Interaction
AI Read Science
Must-Reading Tree
Example
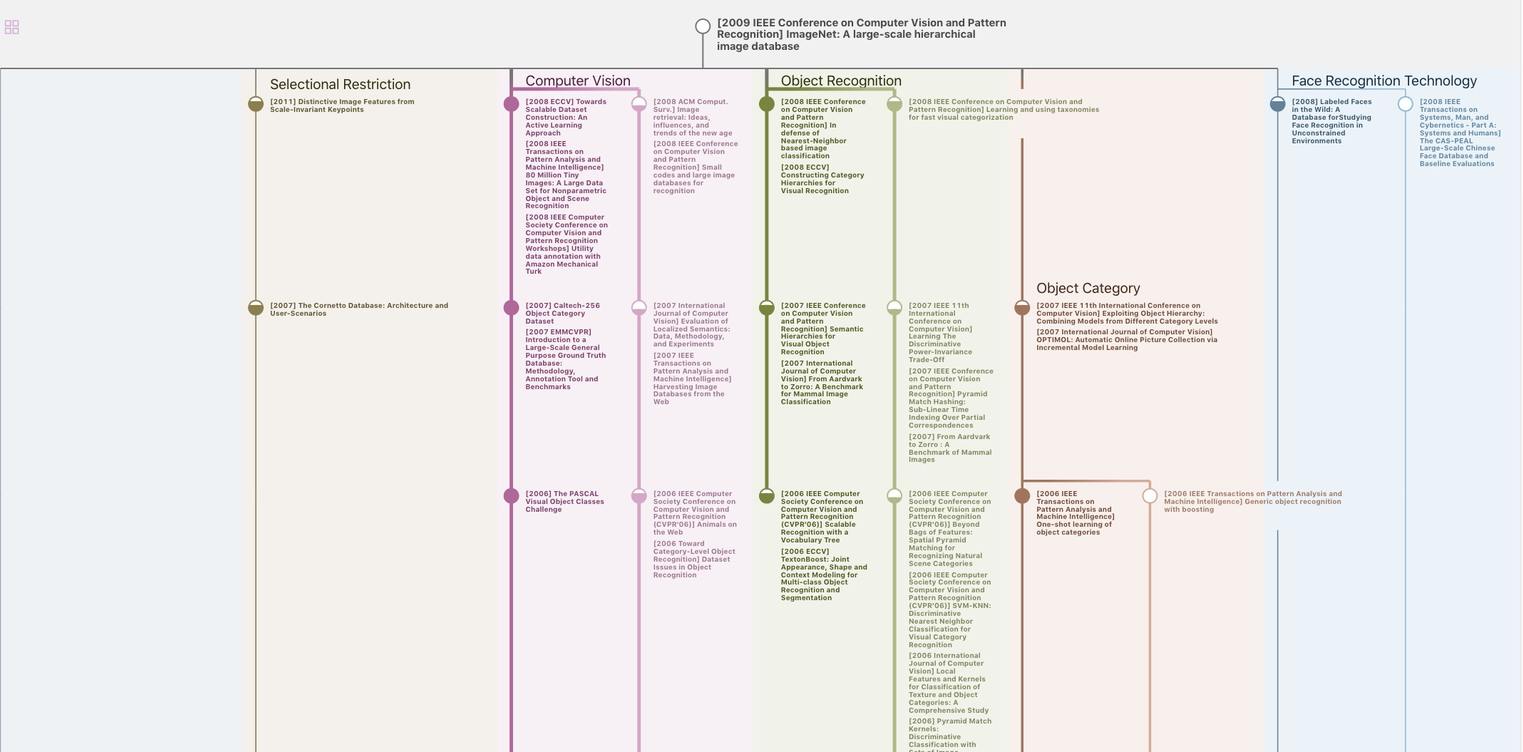
Generate MRT to find the research sequence of this paper
Chat Paper
Summary is being generated by the instructions you defined