Toward Out-of-Distribution Generalization Through Inductive Biases
Philosophy and Theory of Artificial Intelligence 2021(2022)
关键词
Inductive biases, Generalization, Decision making, Causality, Hybrid AI
AI 理解论文
溯源树
样例
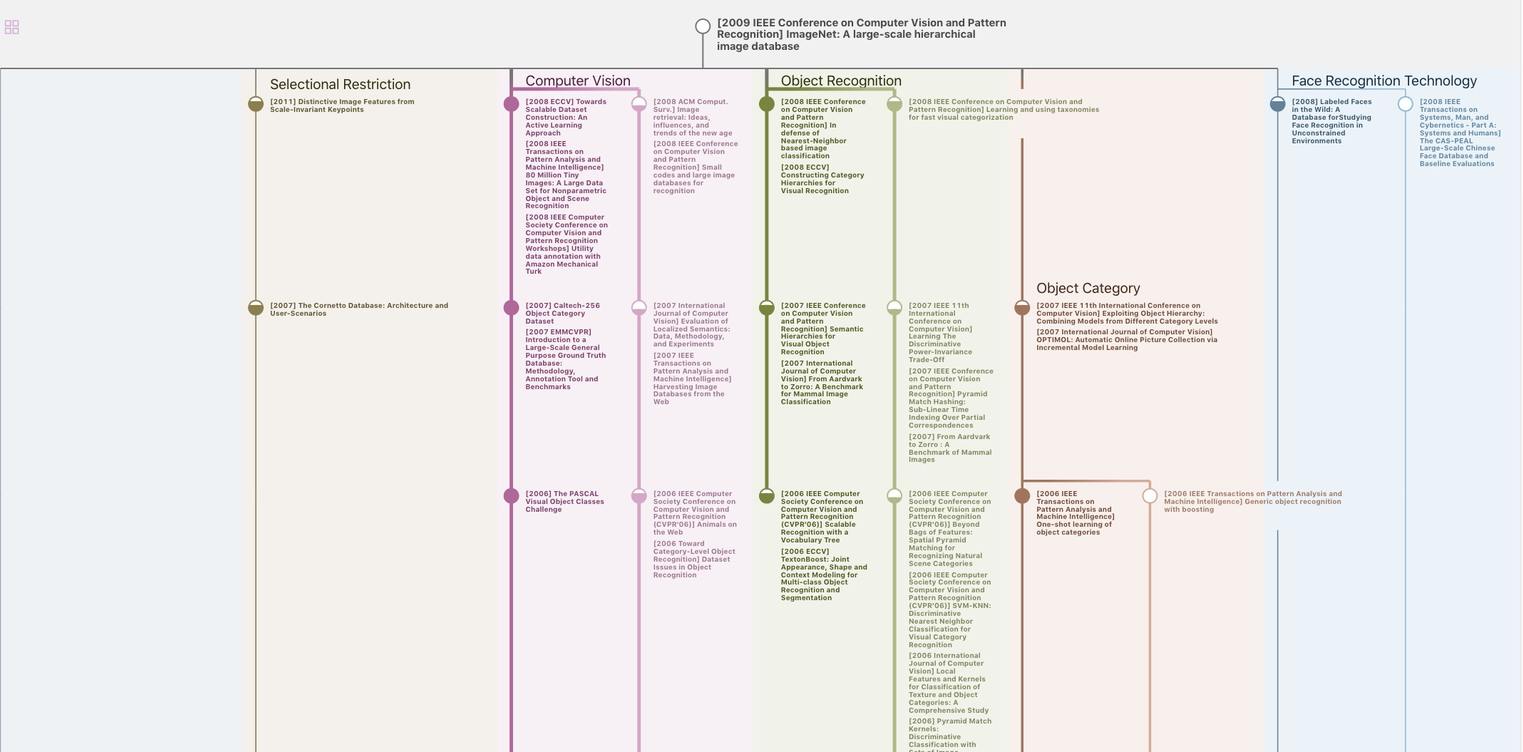
生成溯源树,研究论文发展脉络
Chat Paper
正在生成论文摘要