Exploratory Time-Dependent Flow Visualization via In Situ Extracted Lagrangian Representations
In Situ Visualization for Computational Science(2022)
摘要
This chapter considers exploratory flow visualization of time-dependent vector fields via in situ extraction of Lagrangian representations. The Lagrangian perspective is more capable than the traditional approach of incorporating the increased spatiotemporal data afforded by in situ processing, creating significantly better trade-offs with respect to accuracy and storage. For example, in situ Lagrangian-based flow analysis has delivered the same accuracy as the traditional approach with less than 2% of the storage, or 10X greater accuracy with the same storage. The chapter begins by discussing the Lagrangian frame of reference and how this frame of reference can be used as an in situ operator for data reduction. Next, opportunities for achieving maximum information per byte—where particles are placed, how they are terminated, and how much information to store per particle trajectory are discussed. The chapter then considers post hoc exploration using the Lagrangian representation, as well as the corresponding challenges involved. Finally, the chapter concludes with a qualitative evaluation to demonstrate the efficacy of the technique and a discussion of the current state of the art.
更多查看译文
关键词
visualization,flow,exploratory,time-dependent
AI 理解论文
溯源树
样例
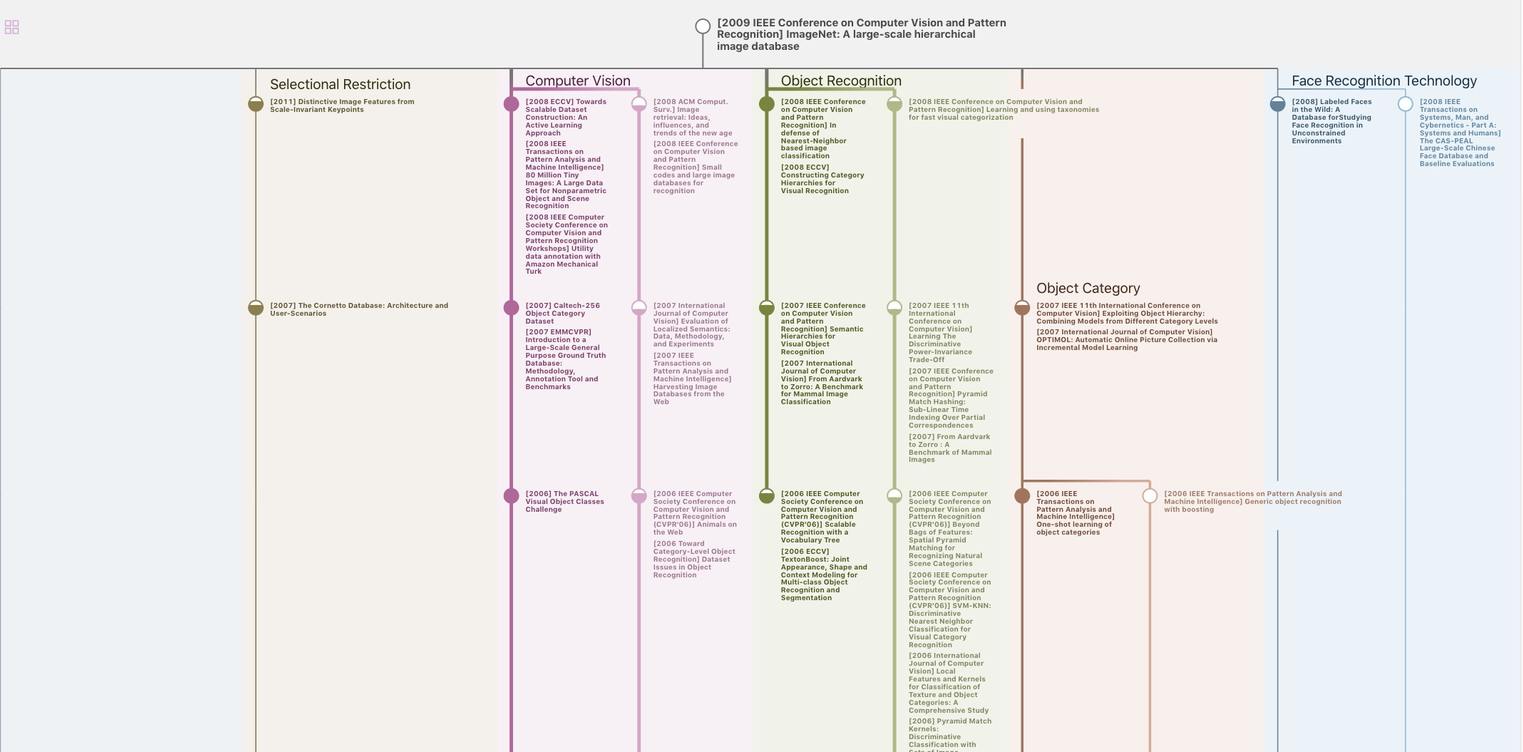
生成溯源树,研究论文发展脉络
Chat Paper
正在生成论文摘要