A Tensor Robust Model Based on Enhanced Tensor Nuclear Norm and Low-Rank Constraint for Multi-view Cancer Genomics Data
Bioinformatics Research and Applications(2023)
摘要
Cancer genomics data often contain multi-view resources of different data types, which provide rich complementary information. Analyzing multi-view cancer genomics data can effectively advance cancer research. The main process of analyzing multi-view cancer genomics data in this paper is to discover the relationship between cancers and genes by clustering cancer samples and identification of differentially expressed genes. To make full use of the consistency and complementarity between genomics data, we propose a new tensor robust model based on enhanced tensor nuclear norm and low-rank constraint (EPTR-TV). First, we define the concept of the enhanced partial sum of tensor nuclear norm (EPSTNN). It dramatically improves the flexibility of the tensor nuclear norm (TNN), effectively avoiding some errors brought by TNN when approximating tensor rank. Then, the anisotropic spatial-temporal total variation (TV) regularization is introduced, which enables the model to exploit the relationship between the structures of tensor data while focusing on the details of the tensor data features. In addition, EPSTNN and TV regularization are unified into the low-rank tensor framework for in-depth analysis of cancer genomics data. Finally, the iterative optimization problem of EPTR-TV is solved by alternating direction method of multipliers (ADMM). Experimental results from clustering and feature selection experiments performed on three multi-view cancer genomics datasets show that EPTR-TV outperforms other comparative models. These suggest that EPTR-TV plays an important role in identifying cancer subtypes and finding new carcinogenic information, thus providing important insights into cancer mechanisms.
更多查看译文
关键词
Cluster analysis, Enhanced tensor nuclear norm, Feature selection, Cancer genomics data, Multi-view model
AI 理解论文
溯源树
样例
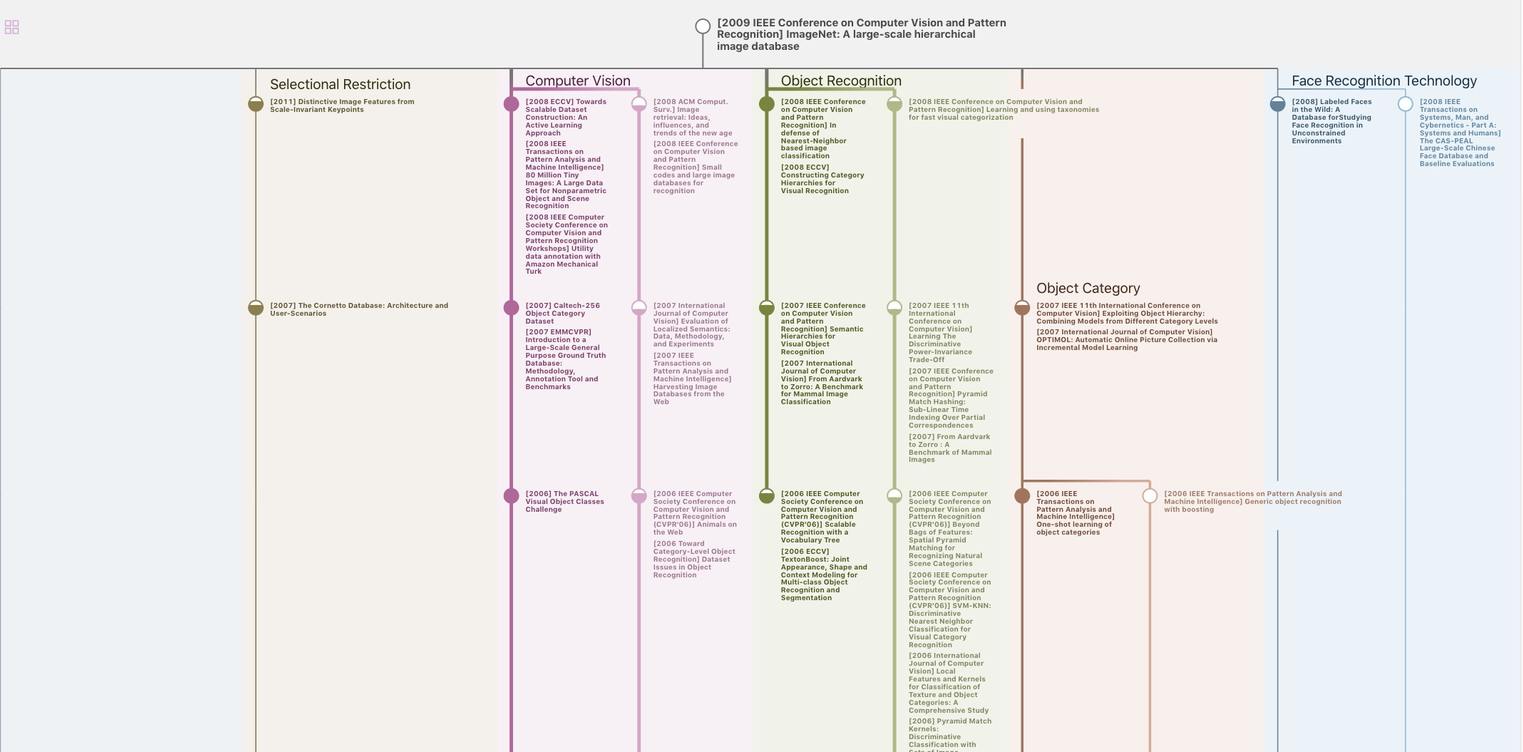
生成溯源树,研究论文发展脉络
Chat Paper
正在生成论文摘要