Scaling neighbor joining to one million taxa with dynamic and heuristic neighbor joining
BIOINFORMATICS(2022)
摘要
Motivation:The neighbor-joining (NJ) algorithm is a widely used method to perform iterative clustering and forms the basis for phylogenetic reconstruction in several bioinformatic pipelines. Although NJ is considered to be a computationally efficient algorithm, it does not scale well for datasets exceeding several thousand taxa (> 100 000). Optimizations to the canonical NJ algorithm have been proposed; these optimizations are, however, achieved through approximations or extensive memory usage, which is not feasible for large datasets. Results:In this article, two new algorithms, dynamic neighbor joining (DNJ) and heuristic neighbor joining (HNJ), are presented, which optimize the canonical NJ method to scale to millions of taxa without increasing the memory requirements. Both DNJ and HNJ outperform the current gold standard methods to construct NJ trees, while DNJ is guaranteed to produce exact NJ trees.
更多查看译文
AI 理解论文
溯源树
样例
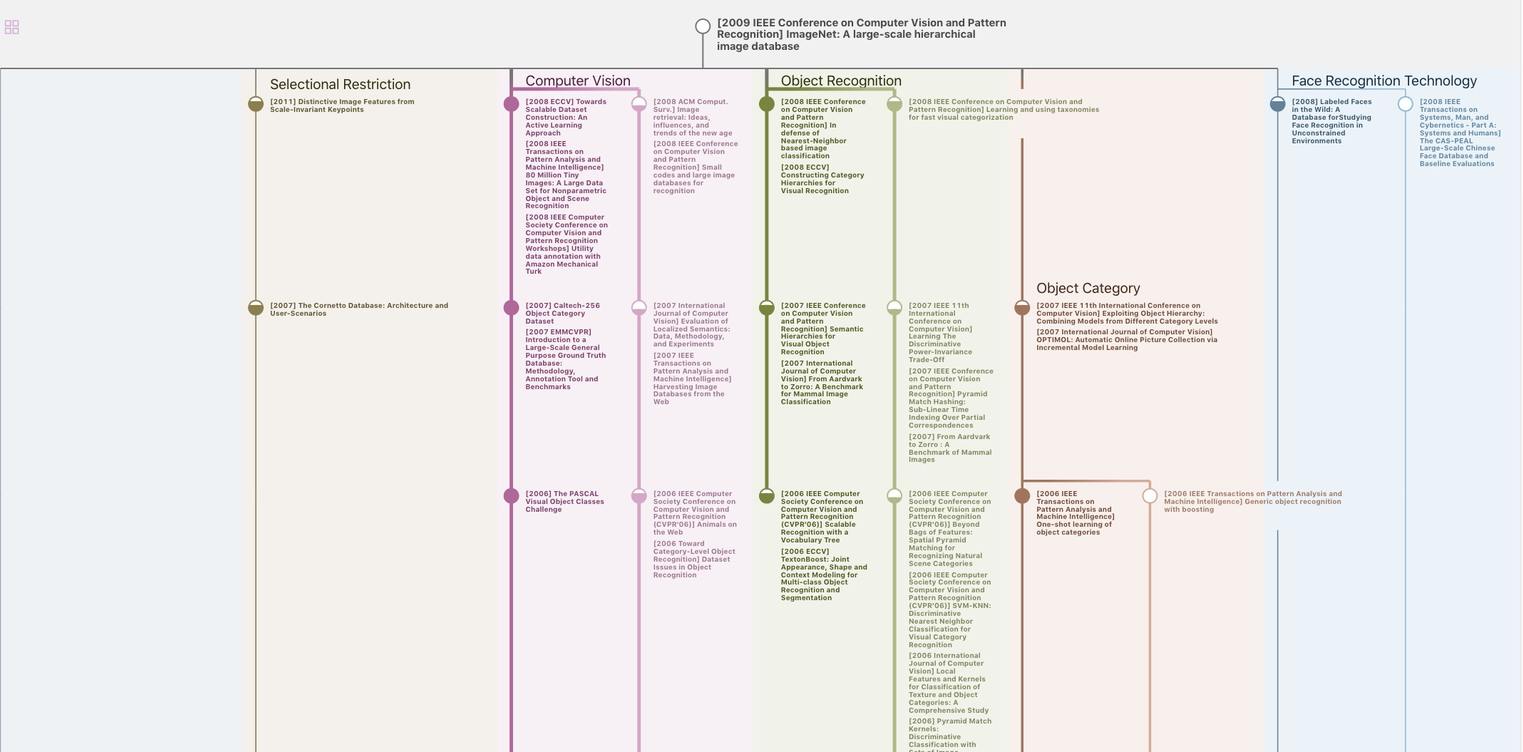
生成溯源树,研究论文发展脉络
Chat Paper
正在生成论文摘要