Deep Learning-based Localization in Limited Data Regimes.
Wireless Network Security (WISEC)(2022)
摘要
As demand for radio spectrum increases with the widespread use of wireless devices, effective spectrum allocation requires more flexibility in terms of time, space, and frequency. In order to protect users in next-generation wireless networks from interference, spectrum managers must have the ability to efficiently and accurately locate transmitters. We present TL;DL, a practical deep-learning based technique for multiple transmitter localization on crowdsourced data where all sensors and transmitters may be mobile and transmit with unknown power. We map sensor readings to an image representing the sensor location, then use a convolutional neural network to learn to generate a target image of transmitter locations. We also introduce a novel data-augmentation technique to drastically improve generalization and enable accurate localization on limited data. In our evaluation, TL;DL outperforms previous approaches on small real-world datasets with low sensor density, in terms of both accuracy and detection.
更多查看译文
关键词
localization,data augmentation,RF sensing,deep learning
AI 理解论文
溯源树
样例
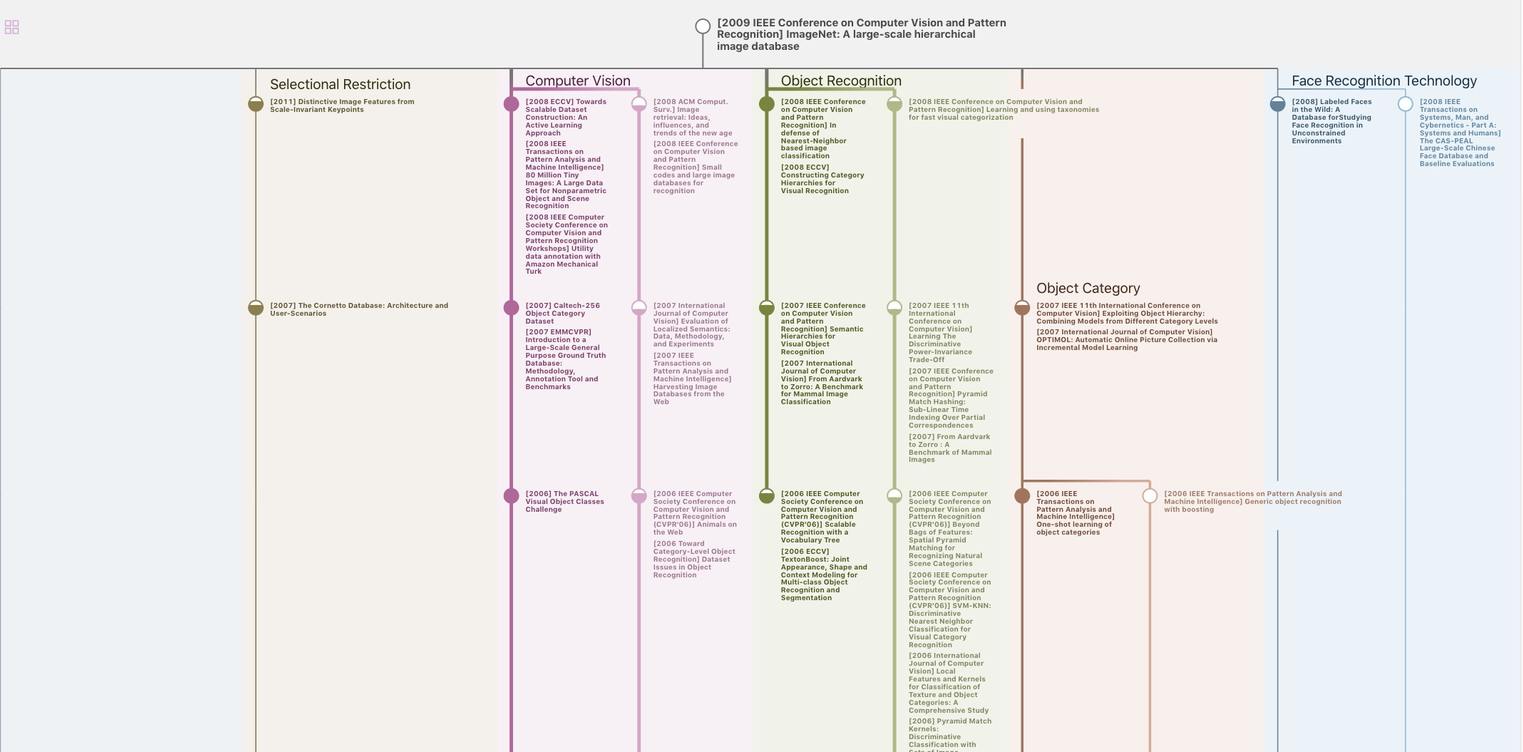
生成溯源树,研究论文发展脉络
Chat Paper
正在生成论文摘要